With industries prioritizing generative AI for innovation and automation, its potential grows. However, risks of generative AI like accuracy and ethical concerns remain. Addressing these challenges is key to ensuring AI benefits humanity.
Explore the top risks of generative AI and steps to mitigate them:
Tools to mitigate risks of generative AI
To reduce risks in generative AI initiatives, organizations can adopt measures like AI governance frameworks and tools focused on securing large language models.
AI governance tools enforce standards, monitor outputs, and provide frameworks for audits and user training. They can track and verify AI-generated content, ensuring AI compliance with licensing and copyright laws.
LLM security tools monitor and correct biases in real-time, ensuring compliance with ethical guidelines to maintain fair content. They implement differential privacy techniques, monitor for data leakage, and secure data processing. These tools also provide frameworks for regular audits to detect and rectify security issues promptly.
1- Accuracy risks of generative AI
Generative AI tools like ChatGPT rely on large language models that are trained on massive datasets. To answer a question or to create a response to a certain prompt, these models interpret the prompt and induce a response based on their training data. Although their training data sets consist of billions of parameters, they are finite pools and the generative models may hallucinate responses from time to time.
There can be many potential accuracy risks caused by generative AI models:
- Generalization over specificity: Since generative models are designed to generalize across the data they’re trained on, they may not always produce accurate information for specific, nuanced, or out-of-sample queries.
- Lack of verification: Generative models can produce information that sounds plausible but is inaccurate or false. Without external verification or fact-checking, users might be misled.
- No source of truth: Generative AI doesn’t have an inherent “source of truth”. It doesn’t “know” things in the way humans do, with context, ethics, or discernment. It’s generating outputs based on patterns in data, not a foundational understanding.
How to Mitigate
Mitigating the accuracy risks of generative AI requires a combination of technical and procedural strategies. Here are some ways to address those risks:
- Data quality and diversity: Ensure that the AI is trained on high-quality, diverse, and representative data. By doing this, the likelihood of the AI producing accurate results across a broad range of queries increases.
- Regular model updates: Continually update the AI model with new data to improve its accuracy and adapt to changing information landscapes.
- External verification: Always corroborate the outputs of generative AI with other trusted sources, especially for critical applications. Fact-checking and domain-specific validation are essential.
- User training: Educate users about the strengths and limitations of the AI. Users should understand when to rely on the AI’s outputs and when to seek additional verification.
Limitations
It has been mathematically proven that hallucinations can not be completely eliminated,1 therefore we should focus on minimizing them.
2- Bias risks of generative AI
Generative AI’s potential for perpetuating or even amplifying biases is another significant concern. Similar to accuracy risks, as generative models are trained on a certain dataset, the biases in this set can cause the model to also generate biased content.
Some bias risks of generative AI are:
- Representation bias: If minority groups or viewpoints are underrepresented in the training data, the model may not produce outputs that are reflective of those groups or may misrepresent them.
- Amplification of existing biases: Even if an initial bias in the training data is minor, the AI can sometimes amplify it because of the way it optimizes for patterns and popular trends.
For example, A 280 billion parameter model showed a 29% rise in toxicity compared to a 117 million parameter model from 2018. As AI systems grow, bias risks also increase.2 The figure below illustrates this trend, where larger models generate more toxic responses.
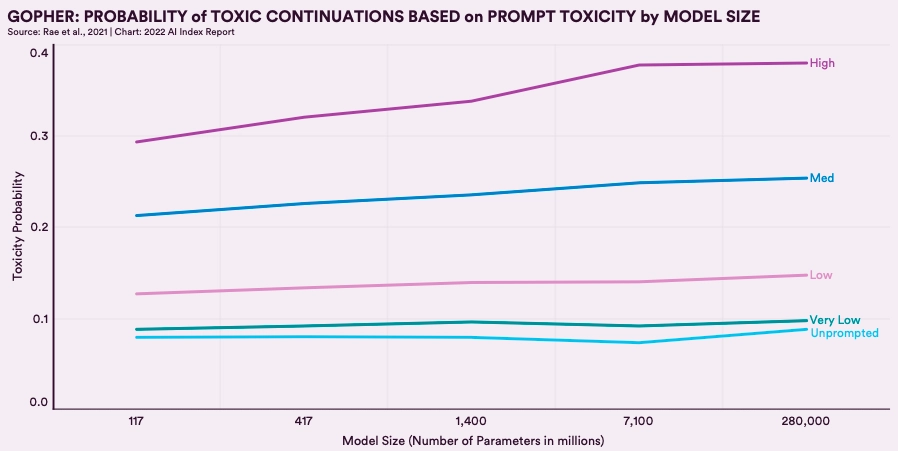
How to Mitigate:
- Diverse training data can help reduce representation bias.
- Continuous monitoring and evaluation of model outputs can help identify and correct biases.
- Establishing ethical standards and supervision during AI development helps minimize bias and encourages responsible use.
3- Data privacy & security risks of generative AI
Generative AI technology, especially models trained on vast amounts of data, poses distinct risks concerning the privacy of sensitive data. Here are some of the primary concerns:
- Data leakage: Even if an AI is designed to generate new content, there’s a possibility that it could inadvertently reproduce snippets of training data. If the training data contained sensitive information, there’s a risk of it being exposed.
- Personal data misuse: If generative AI is trained on personal customer data without proper anonymization or without obtaining the necessary permissions, it can violate data privacy regulations and ethical standards.
- Data provenance issues: Given that generative models can produce vast amounts of content, it might be challenging to trace the origin of any specific piece of data. This can lead to difficulties in ascertaining data rights and provenance.
How to Mitigate:
Nonetheless, using generative models to create synthetic data is a good way of protecting sensitive data. Some steps to mitigate data security threats can be:
- Differential privacy: Techniques like differential privacy can be employed during the training process to ensure that outputs of the model aren’t closely tied to any single input. This helps in protecting individual data points in the training dataset.
- Synthetic training datasets: To mitigate the data security risks, generative models can be trained on synthetic data that are previously generated by AI models.
- Data masking: Before training AI models, datasets can be processed to remove or alter personally identifiable information.
- Regular audits and scrutiny: Regularly auditing AI outputs for potential data leakages or violations can help in early detection and rectification.
4- Intellectual property risks of generative AI
Generative AI poses various challenges to traditional intellectual property (IP) norms and regulations. Also, there are concerns around the eligibility of the AI generated content for copyright protection and infringement. Learn what are two key concerns associated with the intellectual property (ip) rights of content in the context of generative ai?
These IP concerns are hard to address given the complex nature of AI generated content. For example, look at the Next Rembrandt painting in the figure below. It is hard to differentiate from an original Rembrandt painting.
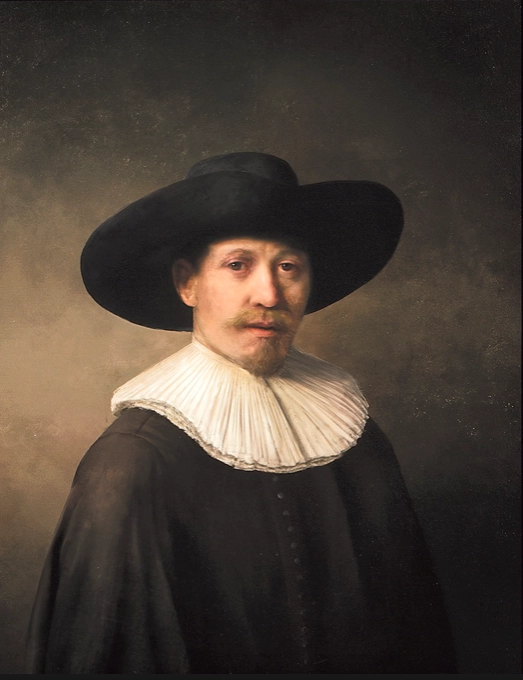
Some of the primary risks and concerns of generative AI around intellectual property are:
- Originality and ownership: If a generative AI creates a piece of music, art, or writing, who owns the copyright? Is it the developer of the AI, the user who operated it, or can it be said that no human directly created it and thus it’s not eligible for copyright? These are problematic concepts when talking about AI generation.
- Licensing and usage rights: Similarly, how should content generated by AI be licensed? If an AI creates content based on training data that was licensed under certain terms (like Creative Commons), what rights apply to the new content?
- Infringement: Generative models could unintentionally produce outputs that resemble copyrighted works. Since they’re trained on vast amounts of data, they might inadvertently recreate sequences or patterns that are proprietary.
- Plagiarism detection: The proliferation of AI-generated content can make it more challenging to detect plagiarism. If two AI models trained on similar datasets produce similar outputs, distinguishing between original content and plagiarized material becomes complex.
How to Mitigate:
- Clear guidelines and policies: Establishing clear guidelines on the use of AI for content creation and IP-related matters can help navigate this complex landscape.
- Collaborative efforts: Industry bodies, legal experts, and technologists should collaborate to redefine IP norms in the context of AI.
- Technological solutions: Blockchain and other technologies can be employed to track and verify the provenance and authenticity of AI-generated content.
5- Ethical risks of generative AI
Over the years, there has been a significant discourse on AI ethics. However, ethical concerns of generative AI is comparatively recent. This conversation has gained momentum with the introduction of various generative models, notably ChatGPT and DALL-E from OpenAI.
- Deepfakes: The biggest ethical concern around generative AI is deepfakes. Generative models can now generate photorealistic images, videos and even sounds of persons. Such AI generated content can be difficult or impossible to distinguish from real media, posing serious ethical implications. These generations may spread misinformation, manipulate public opinion, or even harass or defame individuals.
- Erosion of human creativity: Over-reliance on AI for creative tasks could potentially diminish the value of human creativity and originality. If AI-generated content becomes the norm, it could lead to homogenization of cultural and creative works.
- Unemployment impact: If industries heavily adopt generative AI for content creation, it might displace human jobs in areas like writing, design, music, and more. This can lead to job losses and economic shifts that have ethical implications.
- Environmental concerns: Training large generative models requires significant computational resources, which can have a substantial carbon footprint. This raises ethical questions about the environmental impact of developing and using such models.
How to Mitigate:
- Stakeholder engagement: Engage with diverse stakeholders, including ethicists, community representatives, and users, to understand potential ethical pitfalls and seek solutions.
- Transparency initiatives: Efforts should be made to make AI processes and intentions transparent to users and stakeholders. This includes watermarking or labeling AI-generated content.
- Ethical guidelines: Organizations can develop and adhere to ethical guidelines that specifically address the challenges posed by generative AI.
6- Adversarial Inputs
Adversarial inputs refer to intentionally crafted inputs designed to deceive AI models into making incorrect or harmful outputs. In the context of generative AI, such inputs can subtly manipulate the model to generate biased, false, or even offensive content, which can amplify the existing risks related to accuracy, ethics, and security. The following are examples of such threats:
- Misinformation propagation: Attackers can design prompts that exploit model weaknesses to output misleading or manipulative narratives.
- Toxic content generation: Carefully phrased queries can bypass safety mechanisms and prompt the model to produce offensive or inappropriate content.
- Model exploitation: Adversarial techniques can be used to extract sensitive training data or influence outputs in unintended ways, posing privacy and intellectual property concerns.
How to Mitigate:
- Robust model training: Incorporate adversarial training techniques to expose models to malicious prompts and teach them to respond safely.
- Prompt filtering and sanitization: Implement pre-processing layers to detect and block harmful input patterns.
- Continuous evaluation: Regularly test models with known adversarial inputs to assess their resilience and improve defenses.
External Links
- 1. https://arxiv.org/pdf/2401.11817
- 2. “Artificial Intelligence Index Report 2022.” AI Index.
- 3. “Artificial Intelligence Index Report 2022.” AI Index.
- 4. 'New Rembrandt' to be unveiled in Amsterdam | Rembrandt | The Guardian. The Guardian
Comments
Your email address will not be published. All fields are required.