We analyzed 8,590 scientists’, leading entrepreneurs’ and community’s predictions for quick answers on Artificial General Intelligence (AGI) / singularity timeline:
- Will AGI/singularity ever happen: According to most AI experts, AGI is inevitable.
- When will the singularity/AGI happen: Current surveys of AI researchers are predicting AGI around 2040. However, just a few years before the rapid advancements in large language models (LLMs), scientists were predicting it around 2060. Entrepreneurs are even more bullish, predicting it around ~2030.
- What is our current status: Although narrow AI surpasses humans in specific tasks, a generally intelligent machine doesn’t exist, though some researchers believe large language models demonstrate emerging generalist capabilities.1 According to our AGI benchmark, machines are far from generating economic value autonomously.
- How can we reach AGI: Either by putting more compute and data behind current architectures like transformers or inventing new approaches. There is not yet scientific consensus on the method to achieve AGI or to validate it.
Explore key predictions on AGI from experts like Sam Altman and Demis Hassabis, insights from five major AI surveys on AGI timelines, and arguments for and against the feasibility of AGI:
Artificial General Intelligence timeline
This timeline outlines the anticipated year of the singularity, based on insights gathered from 15 surveys, including responses from 8,590 AI researchers, scientists, and participants in prediction markets:
As you can see above, survey respondents are starting to think that singularity will take place earlier than previously expected.
Below you can see the studies and predictions that make up this timeline or skip to understanding singularity.
Results of major surveys of AI researchers
We examined the results of 10 surveys involving over 5,288 AI researchers and experts, where they estimated when AGI/singularity might occur.
While predictions vary, most surveys indicate a 50% probability of achieving AGI between 2040 and 2061, with some estimating that superintelligence could follow within a few decades.
AAAI 2025 Presidential Panel on the Future of AI Research
475 respondents mainly from the academia (67%) and North America (53%) were asked about progress in AI. Though the survey didn’t ask for a timeline for AGI, 76% of respondents shared that scaling up current AI approaches would be unlikely to lead to AGI.2
2023 Expert Survey on Progress in AI
In October, AI Impacts surveyed 2,778 AI researchers on when AGI might be achieved. This survey included nearly identical question with the 2022 survey. Based on the results, the high-level machine intelligence is estimated to occur until 2040.3
2022 Expert Survey on Progress in AI
The survey was conducted with 738 experts who published at the 2021 NIPS and ICML conferences. AI experts estimate that there’s a 50% chance that high-level machine intelligence will occur until 2059.4
Experts also predicted that hardware cost, algorithmic progress and work on training sets would be the biggest factors in AI progress.
Forecasting AI progress survey in 2019
Baobao Zhang conducted a survey of 296 AI experts, asking them to predict when machines would surpass the median human worker in performing over 90% of economically relevant tasks. Half of the respondents estimated this would happen before 2060.5
AI experts survey on AGI timing in 2019
The predictions of 32 AI experts on AGI timing6 are:
- 45% of respondents predict a date before 2060.
- 34% of all participants predicted a date after 2060.
- 21% of participants predicted that singularity will never occur.
Survey on AI’s potential impact of labor displacement in 2018
Ross Gruetzemacher surveyed 165 AI experts to assess the potential impact of AI on labor displacement. The experts were asked to estimate when AI systems would be capable of performing 99% of tasks for which humans are currently paid, at a level equal to or exceeding that of an average human.
Half of the respondents predicted this milestone would be reached before 2068, while 75% anticipated it would occur within the next 100 years.7
AI experts in 2015 NIPS and ICML conferences survey in 2017
In 2017 May, 352 AI experts who published at the 2015 NIPS and ICML conferences were surveyed.8
Based on survey results, experts estimate that there’s a 50% chance that AGI will occur until 2060. That said, there’s a significant difference of opinion based on geography:
- Asian respondents expect AGI in 30 years,
- North Americans expect it in 74 years.
Some significant job functions that are expected to be automated until 2030 are call center reps, truck driving, and retail sales.
Future Progress in Artificial Intelligence survey in 2012/2013
Vincent C. Muller, the president of the European Association for Cognitive Systems, and Nick Bostrom from the University of Oxford, who published over 200 articles on superintelligence and artificial general intelligence (AGI), conducted a survey of AI researchers. 550 participants answered the question: When is AGI likely to happen?9
According to the results:
- The surveyed AI experts estimate that AGI will probably (over 50% chance) emerge between 2040 and 2050 and is very likely (90% chance) to appear by 2075.
- Once AGI is reached, most experts believe it will progress to super-intelligence relatively quickly, with a timeframe ranging from as little as 2 years (unlikely, 10% probability) to about 30 years (high probability, 75%).
2009 survey with AI experts participating the in AGI-09 conference
Based on the results of the survey with 21 AI experts participating the in AGI-09 conference, it is believed that AGI will occur around 2050, and plausibly sooner.10 You can see below their estimates regarding specific AI achievements: passing the Turing test, passing third grade, accomplishing Nobel worthy scientific breakthroughs and achieving superhuman intelligence.
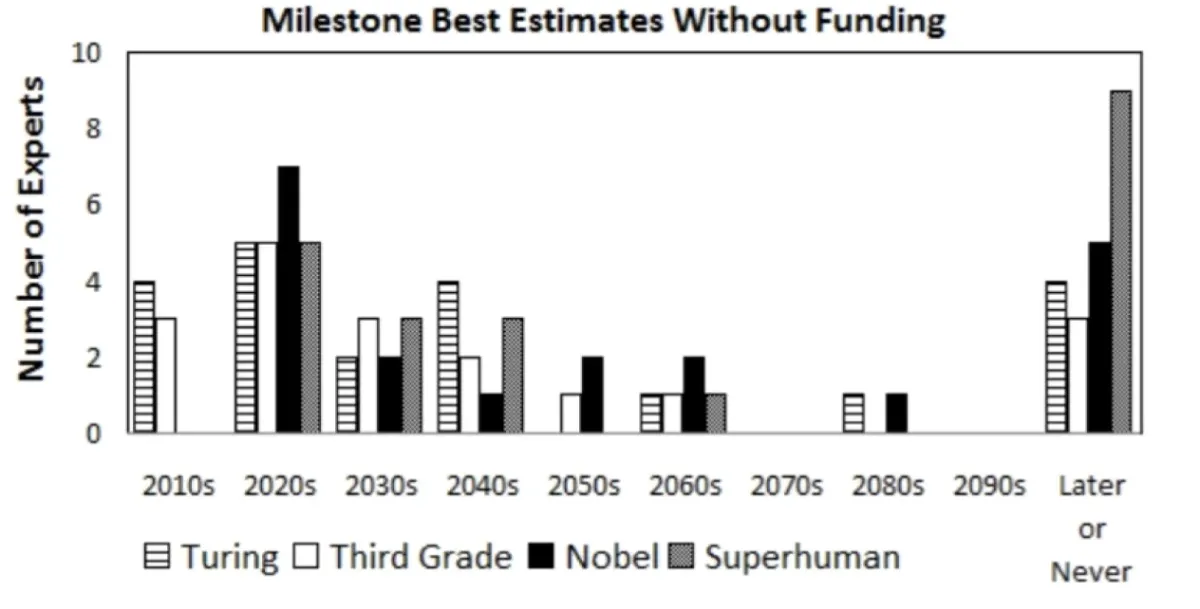
Figure 1: Results from the survey distributed to attendees of the Artificial General Intelligence 2009 (AGI-09) conference
Microsoft’s report on early experiments with GPT-4
Microsoft Research studied an early version of OpenAI’s GPT-4 in 2023. The report claimed that it showed greater general intelligence than previous AI models, performed at a human level in areas like math, coding, and law. This sparked debate on whether GPT-4 was a preliminary form of artificial general intelligence. 11
Community insights
We also evaluated Metaculus community predictions on AGI which involved the predictions of more than 3,290 participants:
- In 2022, 172 participants answered the question “When will an AI first pass a long, informed, adversarial Turing test?” and their prediction was 2029.12
- In 2022, 81 participants answered the question “When will top forecasters expect the first Artificial General Intelligence to be developed and demonstrated?” and their prediction was 2035.13
- In 2020, 1,563 participants answered the question “When will the first weakly general AI system be devised, tested, and publicly announced?” and their prediction was 2026.14
- In 2020, 1,474 participants answered the question “When will the first general AI system be devised, tested, and publicly announced?” and their prediction was 2030.15
Insights from AI entrepreneurs & individual researchers
AI entrepreneurs are also making estimates on when we will reach singularity and they are more optimistic than researchers. This is expected as they benefit from increased interest in AI.
Here are the predictions of 12 of the most prominent AI entrepreneurs and researchers:
- Elon Musk expects development of an artificial intelligence smarter than the smartest of humans by 2026.16
- Dario Amodei, CEO of Anthropic, expects singularity by 2026.17
- In February 2025, entrepreneur and investor Masayoshi Son predicted it in 2-3 years, (i.e. 2027 or 2028)18
- In March 2024, Nvidia CEO Jensen Huang predicted that within five years, AI would match or surpass human performance on any test: 2029.19
- Louis Rosenberg, computer scientist, entrepreneur, and writer, by 2030.
- Ray Kurzweil, computer scientist, entrepreneur, and writer of 5 national best sellers including The Singularity Is Near: Previously 2045,20 , in 2024, 2032.21
- In 2023, Hinton believed that it could take 5-20 years.22
- Demis Hassabis, founder of DeepMind, by 2035.23
- Sam Altman, CEO of OpenAI, by 2035. He mentioned “a few thousand days” in 2024 in his blog “The Intelligence Age”.
- Ajeya Cotra, an AI researcher, analyzed the growth of training computation and estimated a 50% chance that AI with human-like capabilities will emerge by 2040.24
- Patrick Winston, MIT professor and director of the MIT Artificial Intelligence Laboratory from 1972 to 1997: He mentioned 2040 while stressing that while it would take place, it is a very hard-to-estimate date.
- Jürgen Schmidhuber, co-founder at AI company NNAISENSE and director of the Swiss AI lab IDSIA, by 2050.25
Learning from past over-optimism in AI predictions
Keep in mind that AI researchers were over-optimistic before. Examples include:
- Geoff Hinton claimed in 2016 that we wouldn’t need radiologists by 2021 or 2026. So far radiology hasn’t been fully automated and hospitals need thousands of them.26
- AI pioneer Herbert A. Simon in 1965: “machines will be capable, within twenty years, of doing any work a man can do.”27
- Japan’s Fifth Generation Computer in 1980 had a ten-year timeline with goals like “carrying on casual conversations”.28
This historical experience contributed to most current scientists shying away from predicting AGI in bold time frames like 10-20 years, but, this has changed with the rise of generative AI.
Understand what singularity is & why we fear it
Artificial intelligence scares and intrigues us. Almost every week, there’s a new AI scare on the news like developers afraid of what they’ve created or shutting down bots because they got too intelligent.29
Most of these myths result from research misinterpreted by those outside the AI and GenAI fields. Some stoke fear about AI because they may profit from more regulation or it may bring them more attention.
The greatest fear about AI is singularity (also called Artificial General Intelligence or AGI), a system that combines human-level thinking with rapidly accessible near-perfect memory. According to some experts, singularity also implies machine consciousness.
Such a machine could self-improve and surpass human capabilities. Even before artificial intelligence was a computer science research topic, science fiction writers like Asimov were concerned about this. They were devising mechanisms (i.e. Asimov’s Laws of Robotics) to ensure the benevolence of intelligent machines which is more commonly called alignment research today.
Why experts believe AGI is inevitable: Key arguments & evidence
Reaching AGI may seem like a wild prediction, but it seems like quite a reasonable goal when you consider these facts:
- Human intelligence is fixed unless we somehow merge our cognitive capabilities with machines. Elon Musk’s neural lace startup aims to do this but research on brain-computer interfaces is in the early stages.30
- Machine intelligence depends on algorithms, processing power, and memory. Processing power and memory have been growing at an exponential rate. As for algorithms, until now we have been good at supplying machines with the necessary algorithms to use their processing power and memory effectively.
Considering that our intelligence is fixed and machine intelligence is growing, it is only a matter of time before machines surpass us unless there’s some hard limit to their intelligence. We haven’t encountered such a limit yet.
Below is a good analogy for understanding exponential growth. While machines can seem not highly intelligent right now, they can grow quite smart, quite soon.
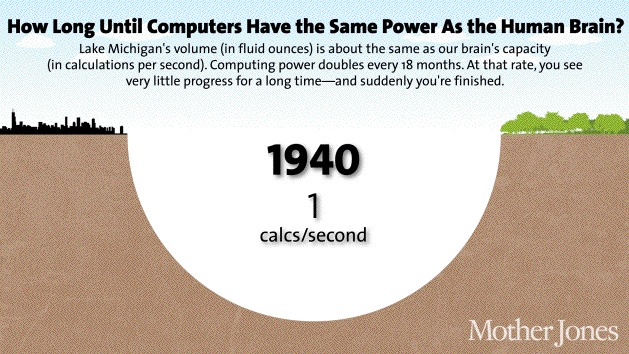
Recent AI growth computing trends
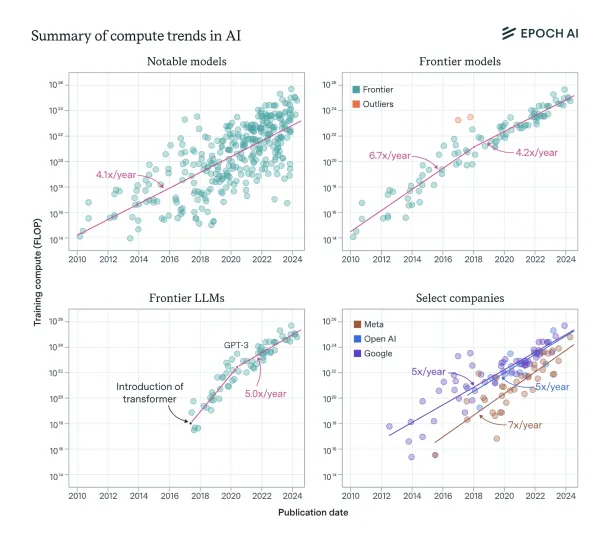
Figure 2: The figure shows a summary of the compute growth patterns observed across various categories: overall notable models (top left), frontier models (top right), leading language models (bottom left), and top models from leading companies (bottom right).
Computational resources for training AI models have significantly increased, with about two-thirds of language model performance attributed to model scale improvements.
According to a 2024 article,31 the growth of compute usage in training AI models has consistently increased by around 4-5x per year, reflecting trends in notable models, frontier models, and top companies like OpenAI, Google DeepMind, and Meta AI (See Figure 2).
However, the growth rate has slowed somewhat since 2018, especially for frontier models, but language models have experienced faster growth up to 9x/year until mid-2020, after which the pace slowed to 4-5x/year.
The overall trend for AI compute growth remains strong, and projections suggest that the growth rate of 4-5x/year will continue unless new challenges or breakthroughs occur. This growth is also seen in the scaling strategies of leading AI companies, though slight variations exist between them.
Despite a slowdown in frontier model growth, the larger models released today, such as GPT-4 and Gemini Ultra, align closely with the predicted growth trajectory.
If classic computing slows its growth, quantum computing could complement it
Classic computing has taken us quite far. AI algorithms on classical computers can exceed human performance in specific tasks like playing chess or Go. For example, AlphaGo Zero beat AlphaGo by 100-0. AlphaGo had beaten the best players on earth.32 However, we are approaching the limits of how fast classical computers can be.
Moore’s law, which is based on the observation that the number of transistors in a dense integrated circuit double about every two years, implies that the cost of computing halves approximately every 2 years.
On the other hand, most experts believe that Moore’s law is coming to an end during this decade.33 However, there are efforts to keep improving efficiency of compute.
For example, DeepSeek surprised global markets with its R1 model by delivering a reasoning model at a fraction of the cost of its competitors like OpenAI.
Quantum Computing, which is still an emerging technology, can contribute to reducing computing costs after Moore’s law comes to an end. Quantum Computing is based on the evaluation of different states at the same time whereas classical computers can calculate one state at one time.
The unique nature of quantum computing can be used to efficiently train neural networks, currently the most popular AI architecture in commercial applications. AI algorithms running on stable quantum computers have a chance to unlock singularity.
Why do some experts believe that we will not reach AGI?
There are 3 major arguments against the importance or existence of AGI. We examined them along with their common rebuttals:
1- Intelligence is multi-dimensional
Therefore, AGI will be different, not superior to human intelligence.
This is true, and human intelligence is also different from animal intelligence. Some animals are capable of amazing mental feats, like squirrels remembering where they hid hundreds of nuts for months.
Yann LeCun, one of the pioneers of deep learning, believes that we should retire the word AGI and focus on achieving “advanced machine intelligence”.34 He argues human mind is specialized and intelligence is a collection of skills and the ability to learn new skills. Each human can only accomplish a subset of human intelligence tasks.35
It is also hard to understand the specialization level of human mind as humans since we don’t know and can’t experience the entire spectrum of intelligence.
In areas where machines exhibited super-human intelligence, humans were able to beat them by leveraging machine-specific weaknesses. For example, an amateur was able to beat a go program that is on par with go programs that beat world champions by studying and leveraging the program’s weaknesses.36
2- Intelligence is not the solution to all problems
Science
For example, even the best machine analyzing existing data may not be able to find a cure for cancer. It must run experiments and analyze results to discover new knowledge in most areas.
This is true with some caveats. More intelligence can lead to better-designed and managed experiments, enabling more discovery per experiment. History of research productivity should probably demonstrate this but data is quite noisy and there are diminishing returns on research. We encounter harder problems like quantum physics as we solve simpler problems like Newtonian motion.
Economy
Intelligence is not the only ingredient to economic value generation.
- IQ, the most commonly accepted measure of human intelligence, is not correlated with net worth for values above ~$40k (See below image):
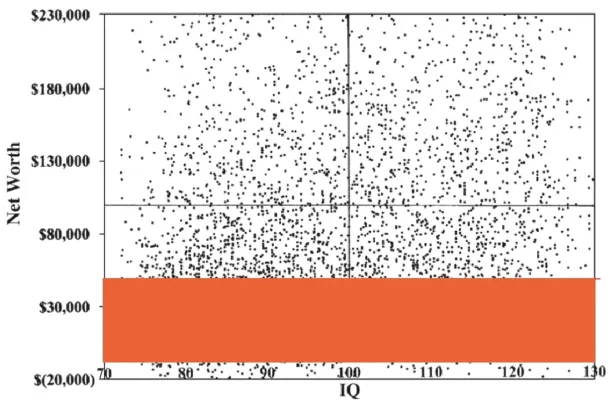
Figure 3: IQ is correlated with wealth at low levels of wealth.37
Figure 4: IQ is not correlated with wealth if we only focus on high levels of wealth. This graph is the same as the one above except that net income levels below $40k have been hidden38
- In the world of investing, the intelligence of a company’s team is not considered a factor of competitiveness. It is implicitly assumed that other companies can also identify intelligent strategies. Investors prefer businesses with unfair advantages that include intellectual property, scale, exclusive access to resources, etc. Most of these unfair advantages can not be replicated only with intelligence.
3- AGI is not possible because it is not possible to model the human brain
Theoretically, it is possible to model any computational machine, including the human brain, with a relatively simple machine that can perform basic computations and access infinite memory and time. This is the universally accepted Church-Turing hypothesis laid out in 1950. However, as stated, it requires certain difficult conditions: infinite time and memory.
Most computer scientists believe that modeling the human brain will take less than infinite time and memory. Nonetheless, there is not a mathematically sound way to prove this belief, as we do not understand the brain enough to precisely understand its computational power. We will just have to build such a machine!
How can we reach AGI?
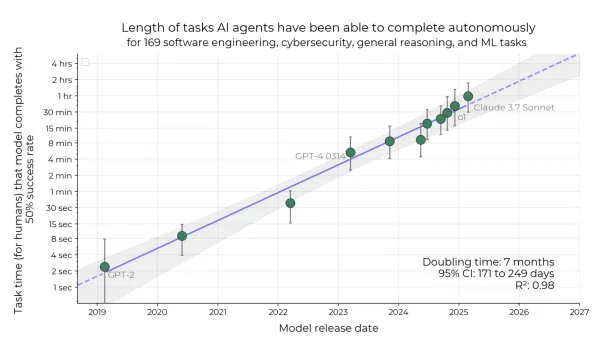
Figure 5: The time horizon of frontier AI models over time shows the longest tasks (in human-equivalent time) each model can complete with 50% reliability.39
The above figure shows how AI agents’ capabilities have progressed over time by measuring the longest tasks they can complete with 50% reliability.
The key finding is that the task length frontier models can handle has grown exponentially—doubling roughly every seven months. This means newer models, like Claude 3.7 Sonnet and o1, can now complete tasks that would take a human nearly an hour, while older models like GPT-2 could barely handle tasks longer than a few seconds.
The shaded region reflects statistical uncertainty, but the overall trend is reliable. If this pattern continues, AI systems could soon handle complex tasks that take humans days or even weeks, marking a significant step toward broader autonomy and AGI-like capabilities.
Scaling as a pathway to AGI
Leaders of frontier AI labs believe that scaling current transformer-based approaches can yield AGI which fuels their predictions about achieving AGI in a few years.
One proposed pathway to AGI is scaling up existing architectures like transformers by increasing compute and data, while another is developing entirely new approaches.
In support of the scaling hypothesis, a 2024 report by Epoch AI analyzed whether AI compute growth can continue through 2030.
They identified four major constraints—power availability, chip manufacturing capacity, data scarcity, and processing latency (See Figure 6).
Despite these challenges, they argue it’s feasible to train models requiring up to 2e29 FLOPs by the end of the decade, assuming significant investments in infrastructure.
Such advancements could produce AI systems far more capable than today’s state-of-the-art models like GPT-4, pushing us closer to AGI.40
Figure 6: The chart illustrates the estimated upper bounds on AI training compute by 2030 under key constraints—power, chip production, data, and latency—with medians ranging from 2e29 to 3e31 FLOP.
Beyond scaling: The case for new architectures
However, influential AI scientists like Yann LeCun believe that scaling large language models will not lead to human-level intelligence.41 They believe that new architectures or approaches are necessary for AGI.
How can we measure whether we have reached AGI
Large language models are blowing past new benchmarks on a weekly basis but evaluating LLMs is difficult. due to issues like data poisoning and the lack of a generally-accepted scientific definition for human-level intelligence.
Old metrics like the Turing test are no match for today’s machines and new metrics like ARC-AGI may lack the generalization capabilities of more broad benchmarks.
There are a few approaches to benchmarking to handle these challenges:
- Frequently updating benchmark questions. Real-life example: LiveBench
- Using holdout sets to prevent data poisoning: AIMultiple’s benchmarks like the AGI benchmark or ARC-AGI.
However, a broader critique from a recent article42 warns that focusing on AGI as the ultimate goal may distort AI research. It creates an illusion of consensus, encourages bad science, ignores embedded social values, lets hype dictate priorities, builds up “generality debt” (postponing key design questions), and excludes marginalized communities and under-resourced researchers.
The authors recommend setting highly specific, measurable, and transparent goals to course-correct. They also call for embracing a pluralism of approaches and objectives, rather than funneling everything toward AGI.
Finally, they stress the importance of fostering inclusion by involving diverse voices and expertise in shaping AI’s future.
This perspective suggests that while technical benchmarks are essential for tracking LLM and AI progress, they are not enough on their own. They must be paired with thoughtful, pluralistic, and inclusive frameworks that avoid the traps of vague “AGI chasing.” Only then can AI development align with broader human and societal needs.
More about Artificial General Intelligence
David Silver, Principal Research Scientist at Google DeepMind, explains that Artificial General Intelligence (AGI) refers to AI systems capable of learning and excelling at a wide range of tasks—much like humans who can become experts in diverse fields such as science, music, or sports.
Unlike narrow AI limited to a single function, AGI aspires to mirror human adaptability and general problem-solving ability.
He notes that while AGI is a long-term goal, reaching true human-level intelligence will likely require several breakthroughs and will develop gradually over time (See below video).
In the TED Talk “The Exciting, Perilous Journey Toward AGI,” Ilya Sutskever, co-founder and Chief Scientist of OpenAI, explores the rapid progress toward Artificial General Intelligence (AGI).
He predicts AGI could emerge within the next 5 to 10 years, though he acknowledges uncertainty in this timeline.
Sutskever highlights both the immense potential and the profound risks of AGI, stressing the need to align its development with human values. Despite the challenges, he is optimistic that humanity can safely guide this powerful technology (See below video).
Ray Kurzweil reflects on over six decades of AI progress, tracing humanity’s ability to build intelligence-enhancing tools, from primitive implements to large language models.
He also predicts that Artificial General Intelligence will arrive by 2029, leading to technological singularity by 2045. He highlights exponential advances in computing power, medicine, and biotechnology.
He also forecasts breakthroughs like AI-generated cures, digital clinical trials, and longevity escape velocity, where scientific progress could extend life indefinitely (See below video).
Check out the below lectures to learn more about AGI:
For more on how AI changes the world, check out Recurrent Neural Networks (RNNs) and AI applications in marketing, sales, customer service, IT, data or analytics.
Conclusion
Predictions for AGI have shifted notably in recent years. While earlier surveys placed its arrival closer to 2060, recent forecasts—especially from entrepreneurs—suggest it could emerge as early as 2026–2035.
This change is fueled by rapid advances in large language models and growing compute power. Yet, despite these gains, today’s AI still lacks the general flexibility and autonomy associated with human-level intelligence.
Experts remain divided on how AGI will be achieved—some believe scaling current architectures will be enough, while others argue that new methods are needed.
Key challenges include high resource demands, unclear benchmarks, and unresolved ethical concerns. AGI may be closer than ever, but its arrival still hinges on both technical breakthroughs and careful oversight.
External Links
- 1. [2311.02462] Levels of AGI for Operationalizing Progress on the Path to AGI.
- 2. https://aaai.org/wp-content/uploads/2025/03/AAAI-2025-PresPanel-Report-Digital-3.7.25.pdf
- 3. 2023 Expert Survey on Progress in AI [AI Impacts Wiki].
- 4. 2022 Expert Survey on Progress in AI – AI Impacts.
- 5. [2206.04132] Forecasting AI Progress: Evidence from a Survey of Machine Learning Researchers.
- 6. When Will We Reach the Singularity? - A Timeline Consensus from AI Researchers (AI FutureScape 1 of 6).
- 7. AI timelines: What do experts in artificial intelligence expect for the future? - Our World in Data.
- 8. [1705.08807] When Will AI Exceed Human Performance? Evidence from AI Experts.
- 9. https://nickbostrom.com/papers/survey.pdf
- 10. https://sethbaum.com/ac/2011_AI-Experts.pdf
- 11. [2303.12712] Sparks of Artificial General Intelligence: Early experiments with GPT-4.
- 12. When will an AI first pass a long, informed, adversarial Turing test?.
- 13. As of July 1, 2022, when will top forecasters expect the first Artificial General Intelligence to be developed and demonstrated?.
- 14. When will the first weakly general AI system be devised, tested, and publicly announced?.
- 15. When will the first general AI system be devised, tested, and publicly announced?.
- 16. Tesla's Musk predicts AI will be smarter than the smartest human next year | Reuters. Reuters
- 17. https://www.youtube.com/watch?v=Xywqm0vlUxk.
- 18. SoftBank, OpenAI to Offer AI Services in Japan - WSJ. The Wall Street Journal
- 19. Nvidia CEO says AI could pass human tests in five years | Reuters. Reuters
- 20. The Singularity Is Near: When Humans Transcend Biology: Kurzweil, Ray: 8601405784551: Amazon.com: Books.
- 21. If Ray Kurzweil Is Right (Again), You’ll Meet His Immortal Soul in the Cloud | WIRED. WIRED
- 22. "Godfather of artificial intelligence" weighs in on the past and potential of AI - CBS News. CBS News
- 23. DeepMind CEO Demis Hassabis Explains What Has to Happen to Achieve AGI - Business Insider. Business Insider
- 24. The brief history of artificial intelligence: the world has changed fast — what might be next? - Our World in Data.
- 25. The "Father of Artificial Intelligence" Says Singularity Is 30 Years Away. Futurism
- 26. Geoff Hinton: On Radiology - YouTube.
- 27. Artificial general intelligence - Wikipedia. Contributors to Wikimedia projects
- 28. Artificial general intelligence - Wikipedia. Contributors to Wikimedia projects
- 29. A guide to why advanced AI could destroy the world | Vox. Vox
- 30. Brain–computer interface - Wikipedia. Contributors to Wikimedia projects
- 31. Training Compute of Frontier AI Models Grows by 4-5x per Year | Epoch AI.
- 32. AlphaGo Zero - Wikipedia. Contributors to Wikimedia projects
- 33. Moore's law - Wikipedia. Contributors to Wikimedia projects
- 34. I think the phrase AGI should be retired and replaced by "human-level AI".… | Yann LeCun | 125 comments.
- 35. A talk I gave at MIT recently. "Objective-Driven AI: towards AI systems… | Yann LeCun | 139 comments.
- 36. Subscribe to read. Financial Times
- 37. IQ is largely a pseudoscientific swindle | by Nassim Nicholas Taleb | INCERTO | Medium. INCERTO
- 38. ScienceDirect.
- 39. https://arxiv.org/pdf/2503.14499
- 40. Can AI Scaling Continue Through 2030? | Epoch AI.
- 41. Unsupported browser.
- 42. https://arxiv.org/abs/2502.03689?utm_source=substack&utm_medium=email
Comments
Your email address will not be published. All fields are required.