Top 7 AI DLP Best Practices in 2024 with Case Studies
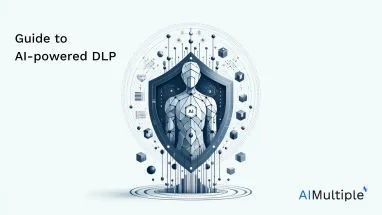
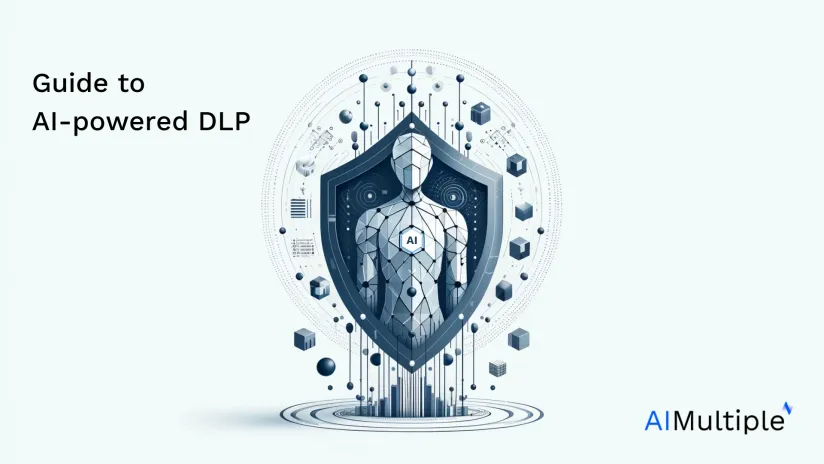
With cyber threats evolving at a fast pace, traditional data loss prevention (DLP) methods are struggling to keep up. Artificial intelligence (AI) has proven to be a game-changer in the fight against data breaches and unauthorized data exfiltration. AI-driven DLP software, or AI DLP, is revolutionizing how organizations safeguard their confidential business data, offering smarter, faster, and more efficient solutions to data security challenges.
This article explores the top 7 ways AI can be harnessed to strengthen your data security and ensure your confidential data remains under lock and key.
1. Leveraging automated DLP software
1.1. AI-enhanced detection and response
The cornerstone of a robust DLP strategy is the implementation of AI-powered DLP software tools. These advanced systems are designed to automatically detect sensitive data across a network, employing machine learning algorithms and natural language processing to identify and classify data elements with precision. Unlike legacy DLP solutions, AI-driven software can adapt and learn from more historical data, reducing false positives and ensuring that high-risk data resources receive the attention they deserve.
1.2. Real-time monitoring and granular controls
With real-time monitoring capabilities, AI DLP tools provide security teams with immediate alerts on potential threats and anomalous activity, facilitating quick response to prevent data leaks. Furthermore, these systems offer granular controls that allow for the fine-tuning of security policies, ensuring that data protection measures are perfectly aligned with the organization’s needs. This dynamic approach not only secures sensitive data against unauthorized access but also supports regulatory compliance, making it an indispensable asset for modern businesses.
The efficiency cannot be matched manually; an automated tool excels in real-time monitoring and granular controls, processing data faster and adjusting security measures more precisely, thus ensuring optimal protection and compliance.
Here is a guide to the top automated DLP software on the market.
Real life example: Aspire Pharmaceuticals1
Aspire Pharmaceuticals needed to safeguard a vast array of sensitive data, requiring detailed policy control and real-time responsiveness to access level adjustments. The company implemented an automated DLP solution that offered the following benefits, making the data protection process more efficient:
- Detailed control over user access rights
- Quicker response to policy changes
- Comprehensive documentation
2. Using predictive analytics to prevent data breaches
2.1. Harnessing the power of AI models
Predictive analytics, powered by AI models, is transforming data security by forecasting potential data breaches before they materialize. By analyzing patterns and hidden trends within data flows, AI tools can identify unusual behavior that may signify a security risk. This preemptive capability allows security teams to address vulnerabilities and strengthen their defenses, effectively reducing the risk of a data breach.
2.2. Enhancing data security with machine learning
Machine learning algorithms play a pivotal role in enhancing predictive analytics for data loss prevention. By continuously learning from past incidents and security breaches, these algorithms become increasingly adept at detecting subtle signs of impending threats. This ongoing improvement in threat detection makes AI-powered predictive analytics an invaluable component of a forward-thinking cybersecurity strategy.
Real life example: John Hopkins2
Johns Hopkins faced challenges in enhancing healthcare data security amidst increasing concerns about patient privacy breaches. It addressed this challenge by implementing an AI-powered application to develop a highly accurate privacy analytics model. This model meticulously reviewed every access point to patient data, identifying potential privacy violations, attacks, or breaches. Through the use of both supervised and unsupervised machine learning techniques as well as transparent AI methods, the institution advanced its data security framework, establishing a predictive, analytics-based, and collaborative privacy analytics infrastructure to protect patient information effectively.
3. AI-driven employee monitoring and access control
3.1. Monitoring for policy violations
One of the more direct approaches to data loss prevention involves monitoring employee access and activities to detect and prevent policy violations. AI DLP solutions excel in this area by providing complete visibility into how sensitive information is handled within the organization. This includes tracking data access, sharing, and storage, ensuring that all actions comply with company policies and data protection standards.
3.1. Intelligent access control
To further secure sensitive data, AI-driven systems can implement intelligent access control measures. By analyzing employee roles, behaviors, and data handling patterns, AI tools can automatically adjust access privileges, ensuring that individuals have access only to the data necessary for their roles. This minimizes the risk of internal data leaks and ensures that sensitive information, such as personally identifiable information (PII) and financial data, is accessed responsibly and securely.
For more on access control:
- Mandatory Access Control (MAC)
- Role-Based Access Control (RBAC)
- Granular Access
4. Leveraging AI in device control
4.1. Device control for hybrid work models
To safeguard devices, especially in a hybrid work model, companies can use AI to monitor and control device access to sensitive data. AI tools can analyze data flows and user behavior in real time, identifying anomalous activity that could signal a security risk. By employing machine learning algorithms, AI can adapt security policies to the evolving landscape of threats, offering tailored protection for each device, whether in the office or remotely. This proactive approach ensures comprehensive data protection, helping maintain compliance and prevent data breaches.
Guide to top device control software.
4.2. Use automate USB encryption
To safeguard devices in a hybrid work model, companies can implement AI-driven security measures that include automatic USB encryption. This ensures that any sensitive data transferred to USB drives is automatically encrypted, reducing the risk of data breaches. AI can monitor device behavior and automatically apply encryption protocols to USB devices, enhancing data security without disrupting user productivity.
You can also use software with USB-blocking capabilities.
5. Automated anomaly detection
5.1. Identifying anomalous behavior
Anomaly detection is a critical aspect of AI-powered DLP, enabling organizations to identify unusual patterns and behaviors that could indicate a security threat. By leveraging large language models and machine learning algorithms, AI tools can sift through vast amounts of data in real-time, pinpointing activities that deviate from the norm. This allows security teams to swiftly investigate and mitigate potential threats before they escalate into full-blown data breaches.
5.2. Continuous learning and adaptation
The strength of AI in anomaly detection lies in its ability to learn and adapt over time. As AI models are exposed to more data and incidents, their ability to discern between benign anomalies and genuine threats improves, reducing the incidence of false positives. This continuous refinement of detection capabilities ensures that organizations can stay one step ahead of cybercriminals, safeguarding their data with unparalleled precision.
5.3 Study on the healthcare sector
A study examined the application of AI in enhancing healthcare security, focusing on anomaly detection, predictive analytics, access control, threat intelligence, and incident response. The results showed that AI significantly enhances anomaly detection in healthcare by efficiently processing vast amounts of real-time data, enabling medical professionals to detect deviations in patient data like lab results and medical images more effectively, thereby reducing misdiagnosis risks and improving patient outcomes.3
6. AI-powered education and training programs
Data security cannot be enhanced without improving the organizational culture. Here are some ways AI can help.
6.1. Leveraging chatbots and generative AI
Educating employees about data security and best practices is a crucial component of a comprehensive DLP strategy. AI-powered education and training programs, including AI chatbots and generative AI tools, can provide interactive and personalized learning experiences for employees. These AI applications can simulate potential security scenarios, guide users through the best responses, and offer instant feedback, reinforcing the importance of data protection in everyday activities.
6.2. Continuous learning and engagement
The advantage of using AI tools for education lies in their ability to offer continuous learning opportunities. Unlike traditional training sessions, AI-driven programs can be accessed anytime, allowing employees to stay updated on the latest security policies and practices. This constant engagement not only fosters a culture of data security awareness but also empowers employees to become active participants in the organization’s data loss prevention efforts.
6.2.1. Recommendation
However, since policies and regulations change, you need to make sure your chatbot is regularly trained on the fresh data.
Watch this to see how chatbots can educate, train, and assist employees:
7. Integrating automated DLP with cloud security and SSE platforms
7.1. Cloud-based data loss prevention
As businesses increasingly migrate to cloud environments, integrating automated DLP with cloud security becomes paramount. AI-driven data loss prevention solutions are uniquely equipped to monitor and protect sensitive data across cloud platforms and SaaS apps. These systems provide visibility into data stored in the cloud, detect sensitive data in transit, and enforce security policies to prevent unauthorized access or data leaks.
7.2. Synergy with SSE platforms
The synergy between AI DLP and Security Service Edge (SSE) platforms enhances data protection in cloud environments. By incorporating AI DLP solutions within SSE frameworks, organizations can leverage advanced security features such as real-time monitoring, anomaly detection, and predictive analytics. This integrated approach ensures that sensitive data remains secure, regardless of where it resides, and supports businesses in maintaining regulatory compliance and safeguarding their intellectual property in the cloud.
Further reading
If you need further help in finding a vendor or have any questions, feel free to contact us:
External resources
- 1. Aspire Pharmaceuticals DLP case study. Endpoint Protector. Accessed: 3/April/2024.
- 2. How Artificial Intelligence Can Overcome Healthcare Data Security Challenges and Improve Patient Trust. Health Catalyst Editors. Accessed: 3/March/2024.
- 3. AI for enhanced healthcare security: an investigation of anomaly detection, predictive analytics, access control, threat intelligence, and incident response. Journal of Advanced Analytics in Healthcare Management, 1(1), 21-37. Accessed: 3/April/2024.

Comments
Your email address will not be published. All fields are required.