Processing, storing, and retrieving vast amounts of information rapidly and efficiently is paramount for businesses. Vector databases are a critical emerging technology in addressing this demand. Unlike traditional databases, vector databases focus on high-dimensional vector data, offering unique advantages for certain use cases.
Businesses and leaders that use emerging technology such as LLM and Generative AI or plan to invest in a project involving such technology need to understand vector databases. We delve into vector databases’ use cases, exploring their most prevalent applications and why it’s becoming indispensable for many industries.
1. Image and Video Recognition
Given the high-dimensional nature of images and videos, vector databases are naturally suited for tasks like similarity search within visual data. For instance, companies with vast image databases can use vector databases to find similar images, facilitating tasks like duplicate detection or image categorization.
Real-life example
Consider a platform like Pinterest. Users often pin images without detailed descriptions. A vector database can represent each image as a high-dimensional vector. When a user pins an image of a coastal sunset, the system can search through its vector database to suggest similar images, perhaps other beach landscapes or sunsets, enhancing content discovery and user engagement.
2. Natural Language Processing (NLP)
In Natural Language Processing (NLP), words or sentences can be represented as vectors through embeddings. With vector databases, finding semantically similar texts or categorizing large volumes of textual data based on similarity becomes feasible, becoming apparent in the Semantic Analysis step (Figure 1).
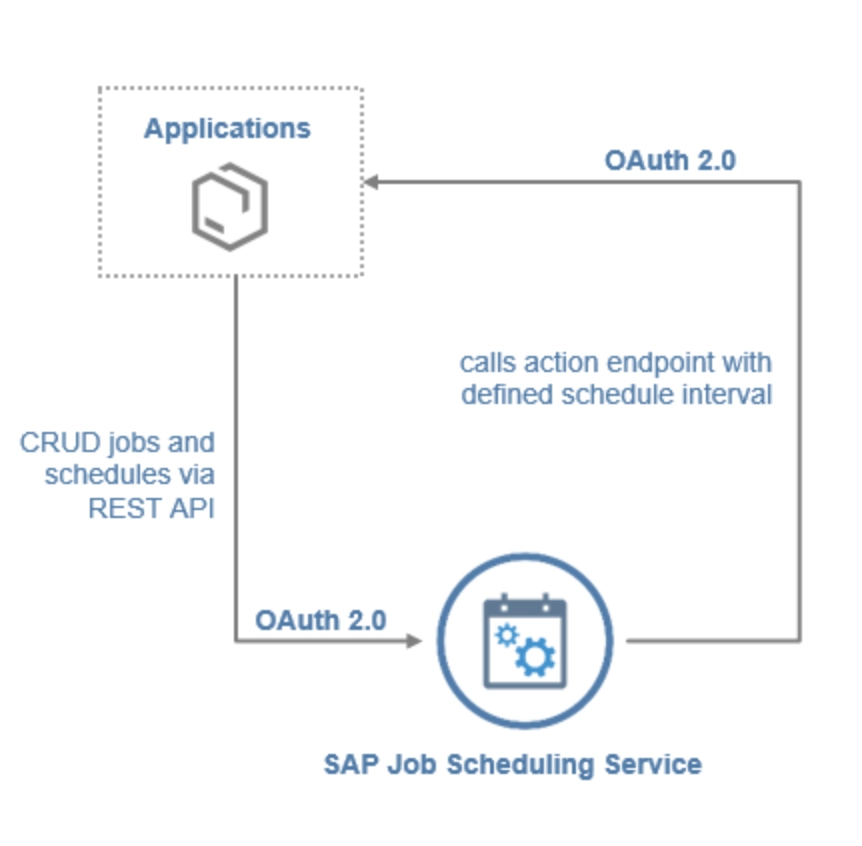
Figure 1: How Does NLP Work? 1
Real-life example
For example in a customer support chatbot system, customer queries are transformed into vectors using embeddings. When a user asks, “How do I reset my password?” the vector database can identify semantically similar queries like “Steps for password change” to provide a relevant response even if the exact phrasing isn’t in the system.
3. Retrieval-Augmented Generation (RAG)
RAG combines the strengths of information retrieval systems with generative AI models, enabling dynamic generation of answers or content based on external data sources. Vector databases are integral to RAG, as they enable efficient retrieval of contextually relevant information by storing document embeddings and quickly finding the most semantically similar documents during queries.
Real-life example
Consider a customer support system enhanced with RAG. When a user asks a question like, “How does the refund policy work?” the system converts the query into a vector and searches a vector database containing policy documents. The retrieved documents are then used to generate a precise, contextually accurate response.
This approach is also widely used in advanced AI-powered search systems like ChatGPT with plugins or Bing AI, where the generative model dynamically incorporates retrieved knowledge for real-time answers.
4. Recommendation Systems
Whether for movies, music, or e-commerce products, recommendation systems often rely on understanding the similarity between user preferences and item features. Vector databases can accelerate this process, making real-time, personalized recommendations a reality.
Real-life example(s)
For example, on Netflix, movies and TV shows are represented as vectors based on their genres, actors, and user reviews. When a user watches a psychological thriller starring a particular actor, the vector database can suggest other movies in the same genre or films with the same actor, offering a tailored viewing experience.
The ‘Top Picks for X’ section we encounter in most streaming platforms are concrete examples. For example, the author of this article watches political TV shows often, and Netflix advises him to watch House Of Cards. See Figure 2.
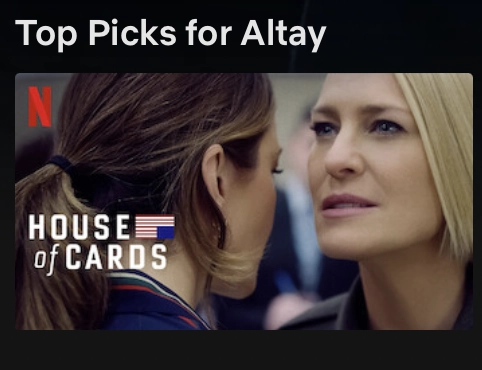
Figure 2: ‘Top Picks’ Feature on Netflix
On a music streaming platform like Spotify, each song can be represented as a vector based on features such as genre, rhythm, melody, and instrumentals. When a user listens to a jazz song with a particular tempo and mood, the platform can use the vector database to suggest other tracks with a similar vibe, enhancing the user experience.
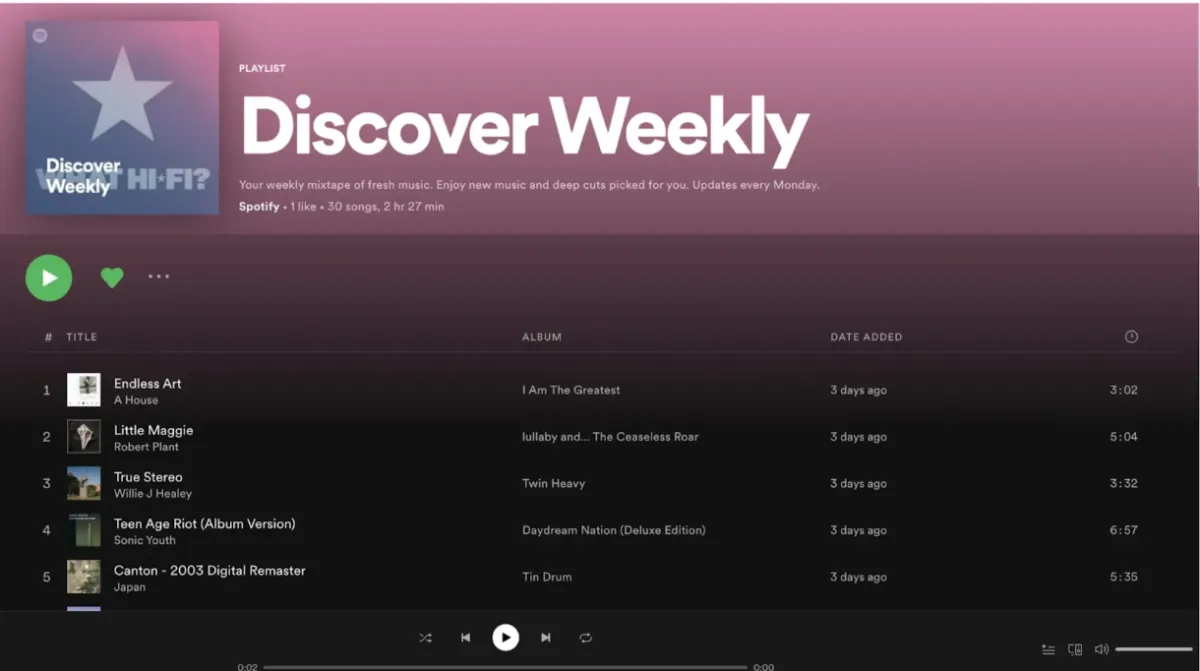
Figure 3: Spotify Discover Weekly
5. Biometrics and Anomaly Detection
From face recognition systems to fingerprint databases, biometric data is high-dimensional and requires efficient similarity search capabilities. Similarly, anomaly detection in systems like network security can benefit from vector databases, where “normal” patterns are vectors, and deviations or anomalies can be quickly identified.
Real-life example
For example, at an international airport, a facial recognition system is used for security concerns. Each passenger’s face is captured and converted into a vector. When a passenger approaches the security check, their face is matched against a vector database of known criminals or persons of interest, ensuring rapid threat detection.
Check our list for biometric authentication software.
6. Drug Discovery and Genomics
In the medical and pharmaceutical fields, molecules and genes can be represented as high-dimensional vectors. Searching for similar compounds or genetic patterns is much more efficient when utilizing a vector database.
Real-life example
For example, chemical compounds are represented as high-dimensional vectors in a pharmaceutical research lab. When researchers identify a compound promising in treating a specific disease, the vector database can find other compounds with similar structures or properties, potentially leading to more efficient drug discovery processes.
Discover other AI applications in the healthcare and pharma industry.
7. Financial Services
High-dimensional data can arise from portfolios, trading patterns, or risk profiles in finance. Vector databases enable rapid similarity searches, which is beneficial for fraud detection or portfolio management tasks.
Real-life example
For example, user transaction patterns are represented as vectors in a digital banking platform. If a user typically makes small, local purchases and suddenly there’s a large international transaction, the system’s vector database can quickly identify this as an anomalous pattern, flagging it for potential fraud investigation.
8. E-commerce Personalization
Imagine an e-commerce platform that sells clothing. A high-dimensional vector can represent each product based on various attributes like color, style, fabric, and customer reviews. When a user browses a product, the system can quickly search the vector database to find items with similar attributes, offering personalized product suggestions.
Over time, this leads to a tailored shopping experience, potentially boosting sales and customer satisfaction. 90% of customers emphasize spending more with companies that personalize their customer service for them.
Check our list for e-commerce personalization software.
9. Healthcare: Patient Similarity Analysis
Vector databases are used extensively in the healthcare industry; one of the wide uses is patient similarity analysis. According to analysis, the total revenue opportunity for the healthcare AI market will exceed $34 billion by 2025.
In a hospital setting, patient records, including symptoms, medical history, and genetics, can be transformed into vectors. If a doctor is treating a patient with a rare set of symptoms, the vector database can identify past patients with similar profiles, enabling the doctor to consider previously effective treatments or identify potential risk factors.
10. Geospatial Data Analysis and Mapping
Vector databases are well-suited for analyzing geospatial data, where locations, routes, or regions can be represented as high-dimensional vectors. These vectors can include coordinates, terrain features, and contextual attributes, making them invaluable for spatial similarity searches and predictive modeling in areas like urban planning, logistics, and navigation.
Real-life example
In logistics, a delivery company like FedEx or UPS can use vector databases to optimize route planning. Delivery points are converted into vectors based on location, traffic patterns, and delivery priorities. The system can find the most efficient routes by comparing these vectors, minimizing costs and delivery times.
Similarly, for urban planning, a city government could analyze urban regions represented as vectors, combining attributes like population density, building types, and land usage to identify areas suitable for development or requiring infrastructure upgrades.
What is a Vector Database?
A vector database is a specialized type of database designed to store, index, and search high-dimensional vectors—typically the kind used in machine learning and AI applications like natural language processing, image recognition, and recommendation systems.
What is a “Vector” in This Context?
A vector is a numerical representation of an object like a word, sentence, image, or video. It’s often generated by a machine learning model and captures the semantic meaning of that object.
For example:
- The word “king” might be represented as
[0.21, -1.03, 0.98, ...]
- A product image might be turned into a 512-dimensional vector
- A user profile might be embedded into a vector to feed into a recommendation engine
These vectors enable machine learning models to perform similarity-based reasoning, which is critical for many AI tasks. There are many open-source vector databases.
Vector Database Use Cases FAQ
How does a vector database differ from a traditional database?
Traditional databases, like SQL and NoSQL, are built for structured data and exact lookups, making them suitable for applications like inventory or transactional data management. Vector databases, however, focus on unstructured data, using specialized indexing to retrieve approximate matches in high-dimensional spaces. This capability makes vector databases essential for applications requiring context-based search and similarity, such as AI recommendations and natural language processing, where finding “similar” rather than “exact” matches is crucial.
How does a vector database work?
A vector database works by storing data as high-dimensional vectors (numerical arrays) that represent complex items like text, images, or audio. These vectors capture relationships in the data, such as similarity or context, which allows the database to perform efficient similarity-based searches rather than exact matches.
1. Data Transformation into Vectors: Data like images or text is processed by a machine learning model, which generates vector embeddings. These embeddings condense complex data into a format that preserves relationships between items—similar items will have similar vectors.
2. Indexing for Efficient Search: To quickly retrieve similar vectors, vector databases use advanced indexing techniques like Hierarchical Navigable Small World (HNSW) graphs, locality-sensitive hashing (LSH), or inverted files. These methods allow the database to find vectors that are close to a given query vector without scanning the entire dataset.
3. Similarity Search: When a query (like a search or recommendation request) is submitted, the query data is converted into a vector. The database then performs a similarity search using distance metrics (such as cosine similarity or Euclidean distance) to find vectors that are closest to the query vector. This process returns data items that are most contextually or visually similar to the query.
4. Scalable and Real-Time Retrieval: Vector databases are optimized for high-dimensional data and can handle large-scale datasets, making them suitable for real-time applications like recommendation systems, semantic search, and fraud detection.
What role do vector databases play in AI-driven personalization?
AI-driven personalization often relies on vectors to represent user preferences and behaviors, which can then be matched against content vectors to provide tailored recommendations. Vector databases excel at processing these comparisons at scale, enabling personalized experiences across streaming platforms, social media, and e-commerce websites. They help improve engagement by offering content that aligns with individual user interests based on historical interaction data.
What are common methods for creating vector embeddings?
Vector embeddings are created using machine learning models such as Word2Vec, BERT, or OpenAI embeddings. These models convert complex, unstructured data into numerical vectors, effectively capturing semantic relationships and context for various data types.
How do vector databases manage high-dimensional data efficiently?
Vector databases use advanced indexing methods like Hierarchical Navigable Small World (HNSW), Locality Sensitive Hashing (LSH), and approximate nearest neighbor (ANN) algorithms. These techniques significantly reduce query time and efficiently manage high-dimensional data by quickly narrowing down relevant vectors for similarity searches.
Can vector databases store metadata alongside vectors?
Yes, vector databases typically support metadata filtering, enabling more refined and context-specific searches. Metadata might include categories, timestamps, or user-generated tags, enhancing data retrieval accuracy and relevance.
What makes cosine similarity suitable for vector search?
Cosine similarity measures the cosine angle between two vectors, effectively capturing their directional similarity irrespective of magnitude. This makes it particularly suited for textual data and semantic search tasks, where direction rather than exact magnitude is crucial.
Are there specific indexing methods recommended for vector similarity search?
Yes, indexing methods such as HNSW, LSH, and inverted indexes are highly recommended for similarity searches. They enhance query performance by rapidly identifying vectors closest to a given query vector, essential for real-time retrieval tasks.
What are some emerging vector database use cases beyond AI personalization?
Beyond personalization, vector databases are seeing rapid adoption in biometrics, drug discovery, and geospatial analysis. For example, in image similarity search, vector search engines allow efficient comparison of high-dimensional vectors to detect duplicates or find related visuals. In genomics, researchers use vector embeddings of gene sequences to locate similar patterns. These use cases showcase how vector databases provide scalable infrastructure for complex data retrieval in science, security, and logistics.
Why are vector databases important for managing unstructured data?
Traditional databases struggle with unstructured data like text, images, and audio. Vector databases can store vector embeddings that encode semantic or contextual meaning, enabling efficient similarity search in large volumes of unstructured content. This flexibility makes them ideal for use cases involving natural language processing (NLP), semantic search, or vector representation of diverse content types.
What does a query vector represent, and how is it used in a vector database?
A query vector is a numerical representation of the user’s input—such as a sentence, image, or product preference—generated using an embedding model. The vector database compares this query against its indexed vectors using distance metrics like cosine similarity to return the most relevant data points. This underpins features like real-time search, recommendations, and anomaly detection.
How does vector indexing improve efficiency in vector databases?
Indexing is crucial for enabling fast access to similar vectors within large datasets. Vector databases often implement algorithms like Hierarchical Navigable Small World (HNSW) or Locality Sensitive Hashing (LSH) to reduce search complexity. These vector indexing methods support efficient data processing and make it feasible to query billions of high-dimensional vectors with low latency.
Can vector databases handle access control and fault tolerance across multiple nodes?
Yes, many vector DB systems are designed to operate on multiple nodes, ensuring fault tolerance and high availability. Access control mechanisms can be applied at the vector or metadata level, which is especially important for enterprise-grade data management platforms that must comply with security standards and data integrity protocols.
What kind of data structure supports vector databases?
Vector databases are typically built on optimized data structures like graphs or trees tailored for high-dimensional indexing. These structures enable fast nearest neighbor lookups and scale better than those used in relational databases or traditional data models, which aren’t designed for fuzzy or approximate matching.
How do different vector databases vary in performance and capability?
There are many vector databases with varying support for embedding models, data structure, scalability, and search methods. Some focus on cosine similarity, while others use Euclidean distance or inner product. Choosing the right system depends on your data type, query load, and real-time needs. When comparing different vector databases, look at how they handle vectors stored, indexing performance, and compatibility with your development process.
Comments
Your email address will not be published. All fields are required.