SAP Datasphere is AI tool that can help deliver meaningful data to the enterprises that struggle to utilize 60% and 73% of their data for analytics, as the latest big data statistics indicate.
Explore SAP Datasphere AI developments, such as new integrations and AI-based features to understand how the tool can help businesses manage their enterprise data:
What is SAP Datasphere AI and why is it important?
SAP Datasphere is one of these tools that SAP has been integrating AI-driven tools and features to meet the data consumer and provider needs. SAP Datasphere AI can improve user experience and support large-scale data sharing by:
- Automated data provisioning: AI can streamline the process of data provisioning by automatically establishing relevant connections and creating necessary artifacts in SAP Datasphere. This reduces the need for manual intervention, making it easier for users to integrate data products.
- Metadata management: AI can enhance the handling of metadata, which is crucial for making data provisioning easier. By utilizing metadata described via the Open Resource Directory (ORD) protocol, AI can ensure that the integration process is smooth and efficient.
Here is a video explaining how SAP Datasphere combines generative AI capabilities with its Datasphere:
SAP has implemented AI in different tools, such as SAP BTP generative AI applications. It also integrates other SAP and non-SAP platforms to extend its tool capabilities, like:
- SAP job schedulers
- SAP process orchestration tools
- SAP utility solutions
- SAP meter-to-cash process technologies.
2 SAP Datasphere AI features
Here is a full list of features provided by SAP datasphere:
1. Knowledge graph
Datasphere Knowledge Graph provides a comprehensive understanding of an organization’s data landscape. By capturing complex data relationships and metadata, it enriches the context for generative AI use cases, enhancing insights and decision-making.
A key feature is automated ontology creation, where the knowledge graph generates an ontology as data is integrated, including crucial business context from SAP sources like S/4HANA. This automation saves time and resources by pre-populating the graph with existing content, such as connections to source systems and foreign keys.
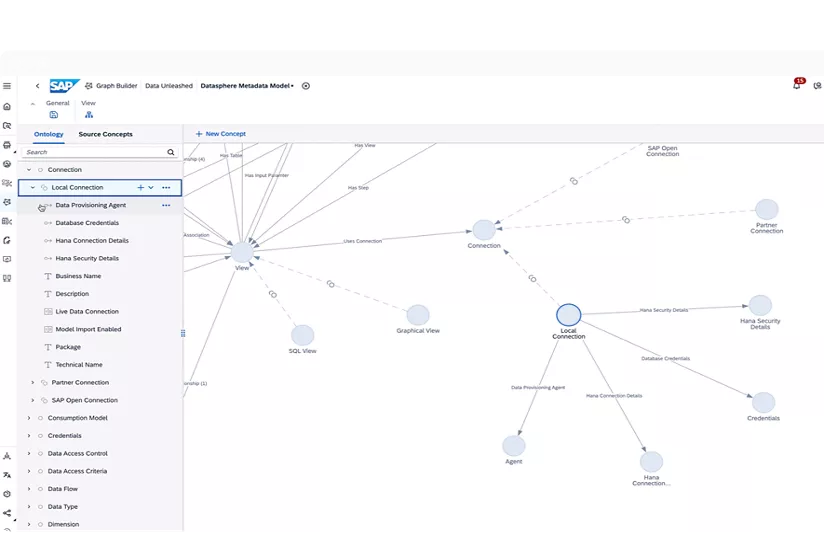
The Knowledge Graph facilitates complex data analysis, enabling better reasoning, insights, and pattern discovery across applications and systems. It supports AI capabilities by providing context to large language models (LLMs) and preventing AI-generated “hallucinations.”
Real-world applications range from marketing optimization to supply chain management, allowing organizations to derive actionable insights and drive innovation.
Here is a video explaining the Knowledge graph capability:
2. Semantic onboarding
Semantic onboarding refers to the seamless import of semantically rich objects from SAP systems like SAP S/4HANA and SAP Business Warehouse (BW)/4HANA into SAP Datasphere. SAP extended this to include support for SAP HANA Cloud and other SAP data products, offering a unified onboarding experience.
Key aspects of semantic onboarding include:
- Preservation of semantic information: When importing calculation views from SAP HANA Cloud into SAP Datasphere, semantic details such as measures, attributes, hierarchies, aggregations, and currencies/units are retained. This ensures that the integrity and meaning of the data are preserved within a semantically rich data layer.
- Synchronization: It keeps objects in SAP HANA Cloud and SAP Datasphere in sync, allowing customers to leverage their existing investments in SAP HANA modeling.
- Business context inheritance: By enabling the semantic onboarding of SAP data products, it allows for the inheritance of business context from SAP line-of-business applications and industry solutions. This transfer of semantics into the business data fabric lays a strong foundation for improved analytic workflows.
- Enhanced analytic workflow: The preservation and transfer of semantic information into SAP Datasphere streamline the analytic workflow, accelerating and simplifying the process of deriving data-driven insights.Integrations with other tools.
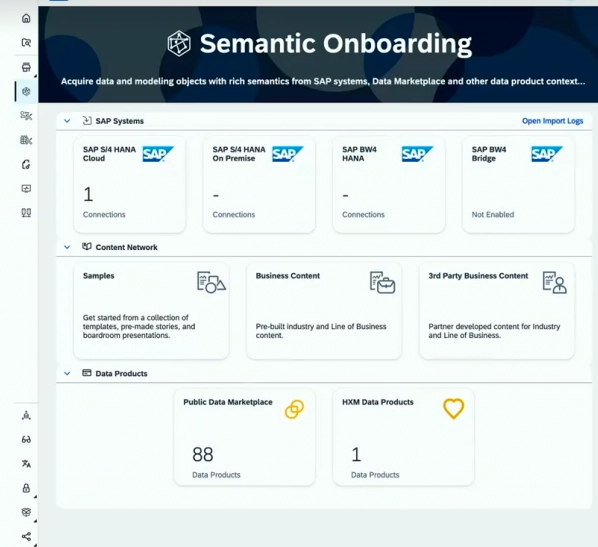
Integrations with other tools
SAP Datasphere integrates AI tools into its open data ecosystem to improve its data management architecture.
1. AI governance with Collibra
SAP partners with Collibra to deliver data governance features, ensuring that trusted data is accessible to all users across the business data fabric.
By integrating with Collibra AI Governance, SAP solutions will allow enterprises to connect the data used for AI with the models themselves on a single platform to track and manage their AI development efforts effectively. By registering the AI and machine learning models created with SAP technology on the Collibra AI Governance platform, users can:
- Gain enhanced visibility into the data lineage and metadata of SAP data utilized in AI modeling
- Check data accuracy and trustworthiness
- Verify the data their AI models were trained on.
As a result, they can:
- Enhance transparency and accountability
- Ensure compliance with regulatory, privacy, and governance policies
- Mitigate risks such as biased decision-making and inaccurate recommendations.
Here is a video explaining how Collier AI governance works:
2. Data streaming with Confluent
Confluent is a platform built around Apache Kafka that provides a real-time data streaming and management service. It enables organizations to efficiently manage large-scale data pipelines and stream data across various systems and applications.
Here’s how Confluent improves SAP Datasphere and enhances SAP data assets:
- Real-time data streaming: Confluent integration allows real-time data streaming to and from SAP Datasphere. This capability is crucial for organizations needing to process and analyze data in real-time, enabling faster decision-making and operational responsiveness.
- Schema registry capabilities: The integration with Confluent’s schema registry extends SAP Datasphere’s outbound capabilities. It allows for the seamless handling of data schemas, ensuring that data is accurately interpreted and processed across systems.
- Superior user experience: The integration provides a superior user experience by facilitating connections at the application level without requiring additional custom application development. This ease of integration helps organizations quickly deploy and utilize streaming data solutions.
- Efficient data processing: SAP Datasphere’s integration with Confluent includes pre-established replication flows that support delta and real-time processing natively. This efficiency means that data is only loaded when changes occur, reducing unnecessary data transfer and processing overhead.
- Enhanced data ingestion and integration: The integration supports data ingestion into Kafka and Confluent, with additional capabilities for both outbound and inbound data integration becoming generally available. This expanded functionality further enhances SAP Datasphere’s ability to handle a wide range of data sources and use cases.
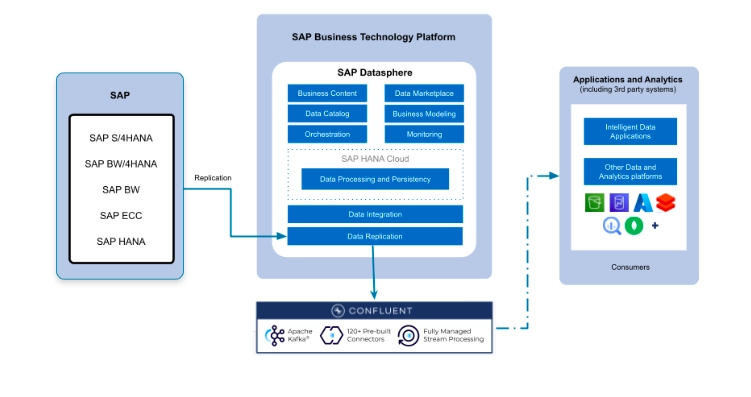
Integration with SAP Analytics Cloud & SAP Hana Cloud Vector Engine
Users can combine SAP Datasphere, SAP Analytics Cloud, and SAP HANA Cloud Vector Engine together to achieve single data management system and analytics solution. Here’s what a user can do with these tools combined:
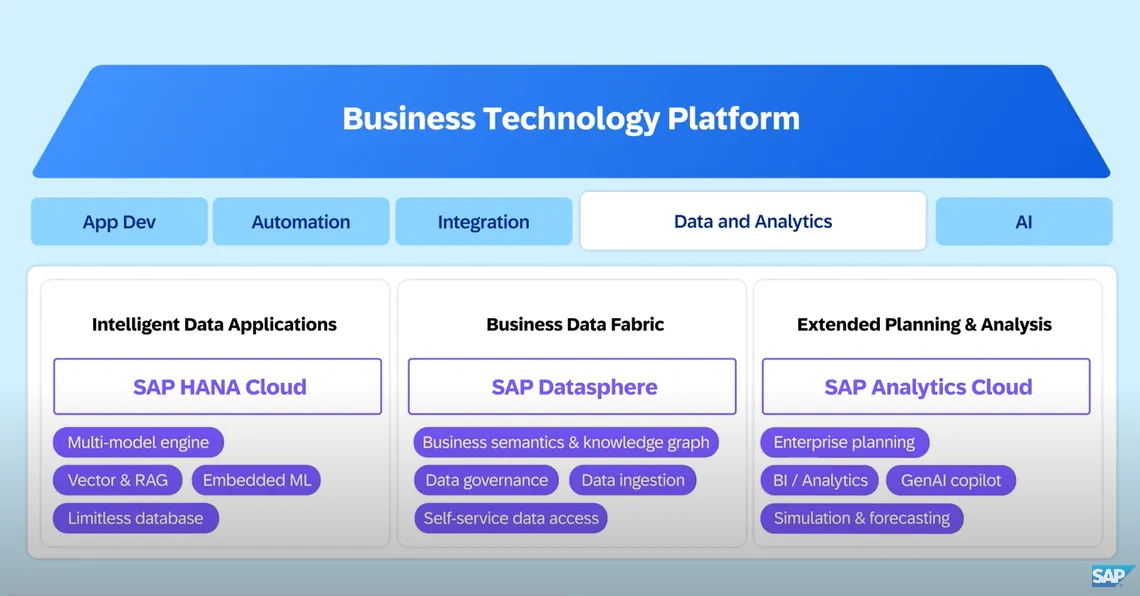
3. SAP Analytics Cloud for data fabric
Data fabric solutions unify and manage data in real time across systems, providing a single, reliable source. They automate access, improve data quality, and enhance security, making analytics and AI easier, even in complex setups. This helps businesses scale and adapt to changing markets. SAP improves data fabric through by:
- Integrating with SAP Analytics Cloud for planning: Planning models from SAP Analytics Cloud can now be deployed into SAP Datasphere. SAP Analytics Cloud utilizes AI to:
- Automate and optimize data transformation and integration processes
- Provide intelligent recommendations and insights based on the integrated actual and planned data. This improves the integration of planned and actual data in real-time decision-making.
allowing for to within SAP Datasphere.
- Reduction in data footprint and modeling efforts: The integration efforts also focus on reducing the data footprint and the effort required for modeling. By leveraging SAP Datasphere’s capabilities, SAP Analytics Cloud can streamline data management processes and improve efficiency.
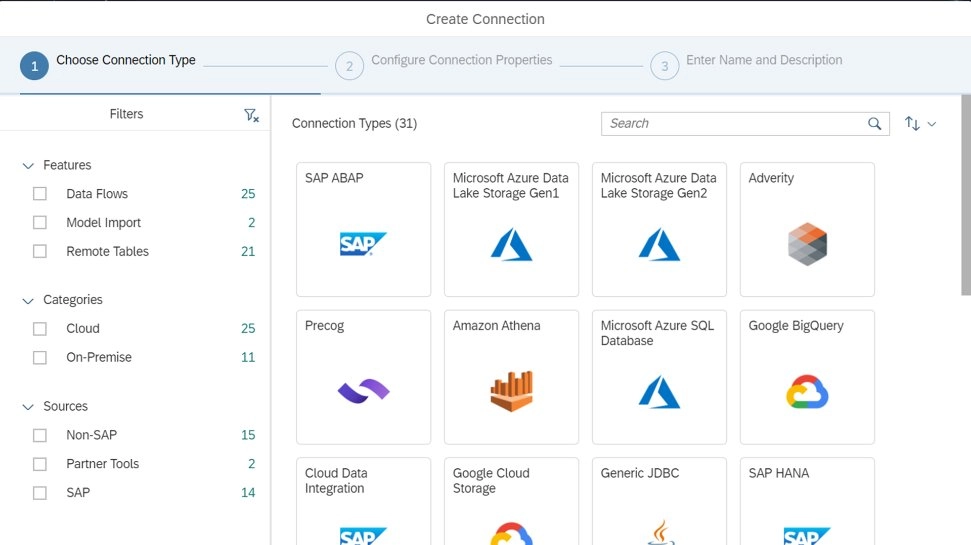
4. Generative AI Co-Pilot
SAP Analytics Cloud Generative AI Co-Pilot empowers SAP customers to leverage generative AI for business planning and simulation. Powered by Joule, it enhances capabilities across data discovery, dashboard creation, planning model maintenance, and more, providing an intuitive user experience.
Using natural language processing (NLP), end users can:
- Run analytical queries
- Simulate impacts of various drivers
- Allocate resources
- Automate dashboards and calculations
For instance, a business user investigating the attrition vs. hiring rate for a specific job over the last 12 months could query Joule to analyze previous plan iterations and uncover underlying reasons (See Figure).
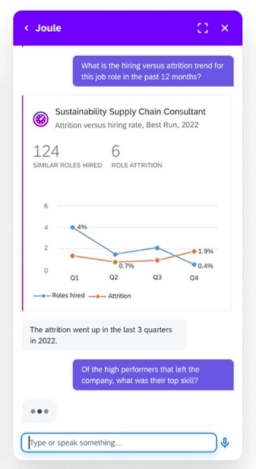
5. SAP Analytics Cloud Compass
SAP Analytics Cloud Compass offers Monte Carlo simulation capabilities with a user-friendly interface, enabling non-technical users to conduct real-time multi-variate analysis without advanced statistical skills. Here are the key benefits of SAP Analytics Cloud Compass:
- Real-time multi-variate analysis to analyze multiple variables simultaneously in real time, providing a comprehensive view of potential outcomes.
- Business simulations for quick and efficient scenario modeling, helping users compare assumptions and report on impact variances.
- Sensitivity analysis to discover real-time insights into sensitivity drivers, helping users understand data dynamics and how changes in variables affect outcomes.
- Continuous agile simulations to adapt to changing business conditions and make informed decisions.
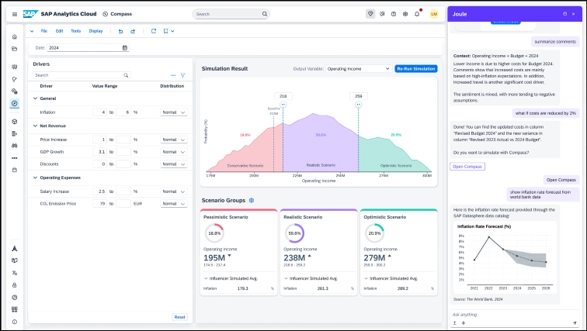
6. SAP HANA Cloud Vector Engine
SAP HANA Cloud introduces a vector capability to its existing multi-model capabilities, enabling customers to leverage all types of business data using a single database. The vector engine enhances the ability to build intelligent data applications that combine human intuition with machine learning and multi-model processing capabilities. Key features and benefits of SAP HANA Cloud Vector Engine include:
- Store and compare vectors using SQL, enabling use cases such as retrieval-augmented generation (RAG), recommendations, classifications, and clustering.
- Simplify data management architecture and enhance with a single multi-model database with SQL interaction.
- Gain new insights by combining spatial, graph, JSON, and relational data with vector queries.
- Easily incorporate vector use cases into solutions within the HANA Cloud ecosystem, including clients, Python libraries, and CAP (Cloud Application Programming Model).
- Integrate with open-source community tools like LangChain via plug-ins.
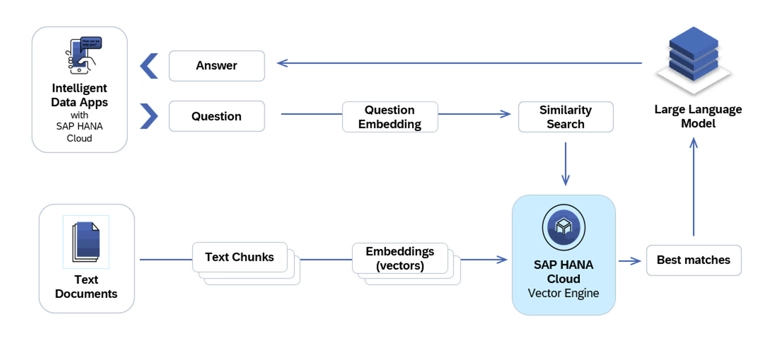
FAQ
What is SAP Datasphere?
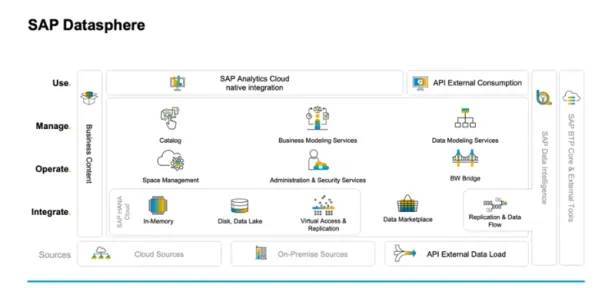
SAP Datasphere is a comprehensive data service built on the SAP Business Technology Platform (SAP BTP). It provides data professionals with seamless and scalable access to critical business data. The platform offers a unified experience for various data-related activities, including:
Data integration
Data cataloging
Semantic modeling
Data warehousing
Data federation
Data virtualization
SAP Datasphere ensures that data professionals can easily distribute essential business data while preserving business context and logic across different data environments.
Further reading
Explore more on SAP related news and applications by checking out our comprehensive and data-driven articles:
- Discover 7 SAP BTP Generative AI Tools, 4 Use Cases & Benefits
- Top 5 SAP BTP Automation Software: Analysis over 500+ Reviews
If you have more questions, do not hesitate contacting us:
External sources
- 1. Business data fabric: Demo video from SAP Data Unleashed in 2024.
- 2. SAP Datasphere on Teched 2023: What has been achieved and where are we going? • Interdobs.
- 3. Set SAP Data in Motion with Confluent Cloud, an SA... - SAP Community .
- 4. Business data fabric: Demo video from SAP Data Unleashed in 2024.
- 5. Business data fabric: Demo video from SAP Data Unleashed in 2024.
- 6. Generative AI with SAP – Part 3: Joule, SAP’s Gene... - SAP Community .
- 7. SAP Analytics Cloud compass - SAP Danmark Nyheder.
- 8. Vectorize your Data : SAP HANA Cloud's Vector Engi... - SAP Community .
Comments
Your email address will not be published. All fields are required.