Guide to RLHF LLMs in 2024: Benefits & Top Vendors
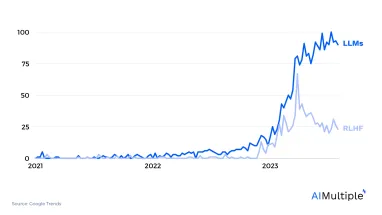
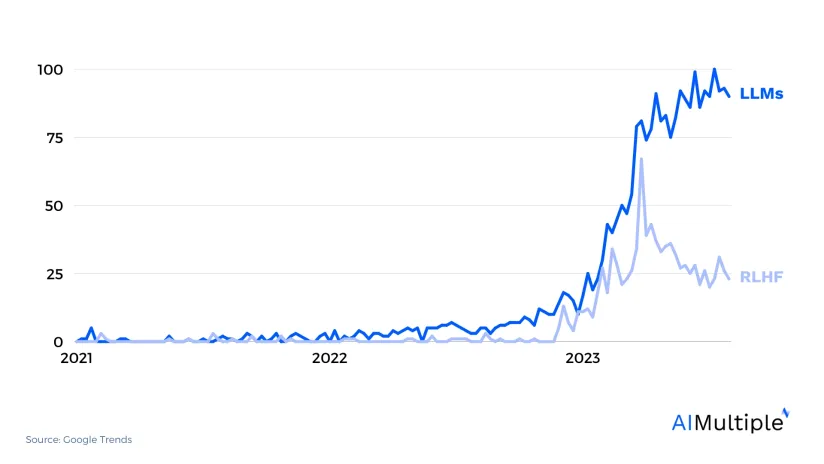
With the outbreak of generative AI and chatbots, the interest in LLMs has rapidly grown in the last couple of years. However, RLHF has seen relatively less growth. Despite its impressive results in the development of AI, generative AI, and LLMs, RLHF is a relatively new approach that many executives still don’t know about.
To fill this knowledge gap, this article explores the relationship between the two abbreviations, how RLHF benefits large language models, and provides a comparison of the top RLHF service providers.
What is RLHF (Reinforcement Learning with Human Feedback)?
Reinforcement learning, or RL, is a machine learning approach where algorithms learn by receiving feedback, typically in the form of a reward function. The traditional method involves training a model to predict the best possible action in a given scenario based on an automated reward system.
RLHF takes this a step further by adding humans to the learning process. It involves the integration of human feedback into the reward system. By incorporating human feedback, the machine learning model gets refined directions, adjusting its behavior based on human preference data.
How does it work?
At the heart of the RLHF training process is the reward model. Instead of relying solely on predefined criteria, it incorporates feedback from humans in the learning process.
A simplified explanation would involve two language models: an initial language model that generates text outputs and a slightly modified version. Human reviewers would then rank the quality of generated text outputs from both models.
This human-generated text comparison aids the automated system in understanding which outputs are more desirable, enabling the reward model to evolve.
It’s a dynamic process, with both human feedback and the reward model evolving together to guide the machine-learning approach.
Figure 1. Reinforcement learning with human feedback process flow
What are LLMs (large language models)?
Large language models, or LLMs, are at the forefront of the AI and machine learning revolution in natural language processing. These machine-learning models are designed to understand and generate text, simulating human-like conversation capabilities.
LLMs are built on vast amounts of text data, undergoing rigorous training processes. Their power is evident in their ability to produce coherent and contextually relevant text based on the initial training data they’ve been provided.
How are they trained?
Training large language models is no small feat. It begins with an initial language model built on a diverse set of training data. This pre-trained language model is then fine-tuned based on specific tasks or domains.
Given the complexity of human language and natural language processing, it’s crucial that such models undergo multiple iterations of refinement. While these models can learn from vast amounts of data, the true challenge lies in ensuring they generate accurate and nuanced responses. That’s where RLHF comes into play.
Sponsored
Clickworker offers RLHF services for LLMs via a crowdsourcing platform. Its global network of over 4.5 million workers serves 4 out of 5 tech giants in the U.S. Clickworker also specializes in preparing training data for LLMs and other AI systems, including:
- Generating and collecting image, audio, video, and text data
- Performing RLHF services
- Processing datasets for machine learning
- Conducting research and surveys
- Conducting sentiment analysis.
How can the RLHF technique benefit LLMs?
The symbiotic relationship between RLHF and LLMs has changed the game in AI-driven language processing. Let’s explore how.
1. More refined LLMs
In the RLHF paradigm, an initial model is trained using traditional methods. This model, while powerful, still has room for improvement. By introducing human feedback integration, the model is refined based on human-provided reward signals.
The process involves training the LLM using reward functions derived from human feedback. This not only refines the model parameters but ensures the model aligns more closely with human conversational norms.
2. Flexible training environment
Instead of a static, pre-defined reward system, the dynamic human-augmented reward model creates a flexible training environment. When the model generates text, the feedback doesn’t just look at the correctness but evaluates nuances, context, and relevance. Such an approach ensures that the generated text outputs are not just technically right but are contextually and emotionally aligned.
3. Continuous improvement
The RLHF approach is not a one-off process. The reward model keeps evolving, taking in more and more nuanced human feedback. This continuous evolution ensures that as language trends change and new linguistic nuances emerge, the large language model remains updated and relevant.
4. Higher level of safety and robustness
Using RLHF allows developers to identify and address unintended model behaviors. By receiving human feedback, potential issues, biases, or inaccuracies in the model’s outputs can be corrected, ensuring the model’s responses are safer and more reliable. This interactive approach ensures a more robust model that’s less prone to errors or controversial outputs.
Why work with an RLHF service provider to develop LLMs?
Developing LLMs can be a resource-heavy and labor-intensive process if done in-house. Working with an RLHF service provider can offer various benefits to your large language model development process.
1. Expertise in human feedback integration
RLHF service providers bring in a deep understanding of how to effectively integrate human feedback into the training process. Their expertise ensures that the feedback generated by human contributors is not just incorporated but is used optimally to guide the AI’s learning.
2. Efficient reward function creation
Given that reward functions play a pivotal role in the RLHF process, an RLHF service provider’s expertise ensures these functions are precise, relevant, and effective. They bridge the gap between the LLM’s understanding of language and human conversational norms.
3. Scalability and continuous refinement
Working with an RLHF partner ensures that the LLM doesn’t just get initial refinement but undergoes continuous improvement. Such partnerships provide an infrastructure where regular human feedback, both positive and negative, is fed into the system, ensuring the model remains top-notch.
4. More diversity
RLHF service providers usually work with a crowdsourcing platform or a large network of workers. This can ensure that the feedback the model receives is varied and encompasses a wide range of human experiences and perspectives.
By tapping into reviewers from different regions and cultures, an outsourced approach can help in training a model that’s more globally aware. This is especially important for LLMs that are meant to serve a global audience, ensuring they don’t reflect just a single regional or cultural perspective.
Comparing the top RLHF service provider on the market
This section compares the top RLHF service providers on the market.
Table 1. Comparison of the market presence category
Company | Crowd size | Share of customers among top 5 buyers | Customer Reviews |
---|---|---|---|
Clickworker | 4.5M+ | 80% | – G2: 3.9 – Trustpilot: 4.4 – Capterra: 4.4 |
Appen | 1M+ | 60% | – G2: 4.3 – Capterra: 4.1 |
Prolific | 130K+ | 40% | – G2: 4.3 – Trustpilot: 2.7 |
Surge AI | N/A | 60% | N/A |
Toloka AI | 245k+ | 20% | – Trustpilot: 2.8 – Capterra: 4.0 |
Table 2: Comparison of the feature set category
Company | Mobile application | API availability | ISO 27001 Certification | Code of Conduct |
---|---|---|---|---|
Clickworker | TRUE | TRUE | TRUE | TRUE |
Appen | TRUE | TRUE | TRUE | TRUE |
Prolific | FALSE | TRUE | FALSE | TRUE |
Surge AI | FALSE | TRUE | TRUE | FALSE |
Toloka AI | TRUE | TRUE | TRUE | TRUE |
Notes & observations from the tables:
- The company selection criteria will be updated as the market, and our understanding of the market evolves.
- The information on the company’s capabilities was not verified. A service provider is assumed to offer a capability if that capability is mentioned in their services page or case studies as of Aug/2023. We may verify companies’ statements in the future.
- The company’s capabilities were not quantitatively measured. We checked if capabilities were offered or not. In a benchmarking exercise with products, quantitative metrics can be introduced in the future.
- All data added to the tables is based on company claims.
- The companies selected in this comparison were based on the relevance of their services.
- All service providers offer API integration capabilities.
How to find the right RLHF service provider for your project?
This section lists the criteria we used to select the RLHF service providers compared in this article. The readers can also use this criterion to find the right fit for their business. The criteria is divided into 2 categories:
- Market presence
- Feature Set
Market presence
1. Share of customers among top 5 buyers
To understand the company’s market footprint and get insight into its relevance and dominance in the market, examine its clientele among these top 5 tech giants:
- Samsung
- Apple
- Microsoft
- Meta
2. User reviews
Check reviews on G2 and Trustpilot for insights into the company’s performance. Ensure reviews match the specific service you’re considering since companies offer varied services.
Feature set
3. Platform Features
Examine the service provider’s platform. We considered if the platform offered a mobile app or API integration.
4. Data protection practices
Given the increase in cyber threats, robust data protection is vital. We looked for ISO 27001 certification.
5. Code of conduct
Your partner’s ethics affect your reputation. Ensure they uphold fair practices and have a code of conduct in place for workers.
Further reading
- 5 AI Training Steps & Best Practices
- Crowdsource Machine Learning: A Complete Guide
- AI Improvement: Recommendations & Top 7 Techniques
If you need help finding a vendor or have any questions, feel free to contact us:

Comments
Your email address will not be published. All fields are required.