Businesses increasingly leverage AI-powered visual data solutions, but confusion between image recognition and classification leads to inefficiencies. Understanding the key differences helps businesses optimize AI deployment in the security, healthcare, and retail fields.
Explore image recognition vs classification, their key differences, and applications with real-life examples.
Image recognition: Turning pixels into meaningful information
What is image recognition?
Image recognition analyzes images or video clips to identify and detect visual features such as objects, people, and places. This is achieved by using sophisticated algorithms and models that analyze and compare the visual data against a database of pre-existing patterns and features.
Image recognition is a complex and multi-disciplinary field that combines computer vision, artificial intelligence, and machine learning techniques to perform tasks such as facial recognition, object detection, and scene analysis.
Image recognition is used across various industries. In healthcare, it aids in medical image analysis and disease diagnosis. Autonomous vehicles rely on object detection models for traffic signs and obstacle detection.
Facial recognition enhances security & surveillance, while augmented reality and image classification improve retail and social media experiences.
Manufacturing benefits from object detection tasks for quality control, and agriculture uses satellite images for crop monitoring. Additionally, financial services, robotics, and education leverage image processing for security, automation, and research.
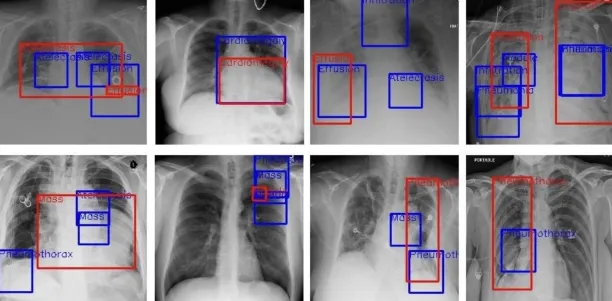
Figure 1: An example of image recognition: different images of chest x-rays with box labels.
Image classification: Sorting images into categories
What is image classification?
Image classification, a key task in computer vision, involves assigning class labels to an entire image using deep learning models like convolutional neural networks (CNNs).
It is widely used in medical image analysis for disease diagnosis, autonomous vehicles for traffic sign recognition, and facial recognition for security.
Retail and eCommerce use image classification models for product categorization, while agriculture applies it to satellite images for crop health monitoring. Financial services, social media, and education also leverage image classification for fraud detection, content moderation, and document digitization.
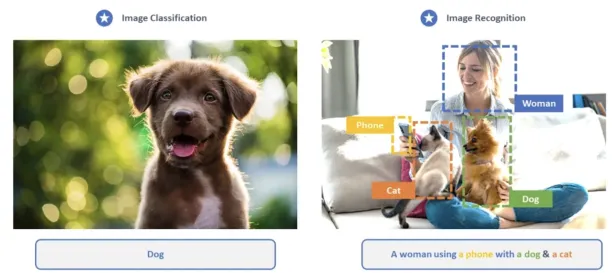
Figure 2: An illustration explaining the difference between image recognition vs classification.
Image recognition vs. classification: Main differences
Context | Image recognition | Image classification |
---|---|---|
Function | Identifying and locating objects in images. | Sorting images into categories. |
Applications | Autonomous vehicles, surveillance systems, facial recognition. | Diagnosing medical conditions, analyzing satellite images. |
Complexity | More computationally intensive due to identifying and localizing multiple objects. | Assigns labels to the entire image, leading to faster processing times. |
While image recognition and classification are related, they have notable differences that make them suitable for distinct applications.
1. Object detection vs. categorization
Image recognition focuses on identifying and locating specific objects or patterns within an image, whereas image classification assigns an image to a category based on its content.
In essence, image recognition is about detecting objects, while image classification is about categorizing images.
2. Use cases and applications
Image recognition is ideal for applications requiring the identification and localization of objects, such as autonomous vehicles, security systems, and facial recognition.
Image classification, however, is more suitable for tasks that involve sorting images into categories, like organizing photos, diagnosing medical conditions from images, or analyzing satellite images.
3. Complexity and processing time
Image recognition is generally more complex than image classification, as it involves detecting multiple objects and their locations within an image. This can lead to increased processing time and computational requirements.
Image classification, on the other hand, focuses solely on assigning images to categories, making it simpler and often faster.
How are image recognition and image classification related?
Despite their differences, image recognition and image classification share some common ground:
1. Techniques and technologies
Both processes use similar techniques and technologies, such as machine learning algorithms and deep learning models like convolutional neural networks (CNNs). These methods can be adapted for image recognition or classification tasks depending on the specific application.
2. Interdependence in applications
In some applications, image recognition and image classification are combined to achieve more sophisticated results.
For instance, an autonomous vehicle may use image recognition to detect and locate pedestrians, traffic signs, and other vehicles and then use image classification to categorize these detected objects. This combination of techniques allows for a more comprehensive understanding of the vehicle’s surroundings, enhancing its ability to navigate safely.
3. Feature extraction and analysis
Image recognition and image classification involve extracting and analyzing image features. These features, such as edges, textures, and colors, help the algorithms differentiate between objects and categories.
In both cases, the images’ quality and the extracted features’ relevance are crucial for accurate results.
Real-world applications of image recognition and classification
To further clarify the differences and relationships between image recognition and image classification, let’s explore some real-world applications.
1. Medical imaging
Medical imaging is a popular field where both image recognition and classification have significant applications. Image recognition is used to detect and localize specific structures, abnormalities, or features within medical images, such as X-rays, MRIs, or CT scans. This helps medical professionals diagnose and treat various conditions.
Image classification, on the other hand, can be used to categorize medical images based on the presence or absence of specific features or conditions, aiding in the screening and diagnosis process. For instance, an automated image classification system can separate medical images with cancerous matter from ones without any.
Real-life example:
The UK’s National Health Service (NHS) has initiated a landmark trial involving nearly 700,000 women to evaluate the use of artificial intelligence in breast cancer screening. The AI technology analyzes mammograms to detect tumors earlier and more efficiently, potentially doubling the workload capacity of radiologists and improving early-stage cancer identification.1
Check out computer vision healthcare for more examples.
2. Security
Image recognition and classification are critical tools in the security industry that enable the detection and tracking of potential threats. Automated image recognition solutions match real-time surveillance images with pre-existing data to identify individuals of interest, while image classification solutions categorize and tag objects in surveillance footage.
Facial recognition is a specific form of image recognition that helps identify individuals in public areas and secure areas. These tools provide improved situational awareness and enable fast responses to security incidents.
Real-life example:
New York Police Department (NYPD) utilizes advanced facial recognition software to analyze surveillance footage, aiding in the identification and apprehension of suspects. In a recent case, the NYPD leveraged this technology, alongside other tools like drones and DNA analysis, in the investigation of a high-profile assassination.2
3. Environmental monitoring
Environmental monitoring and analysis often involve the use of satellite imagery, where both image recognition and classification can provide valuable insights. Image recognition can be used to detect and locate specific features, such as deforestation, water bodies, or urban development.
Image classification, meanwhile, can be employed to categorize land cover types or identify areas affected by natural disasters or climate change. This information is crucial for decision-making, resource management, and environmental conservation efforts.
4. Retail and eCommerce
Leveraging image recognition and classification in retail and eCommerce can enhance customer experience, inventory management, fraud detection, and marketing.
AI-powered visual search, personalized recommendations, and AR shopping improve engagement, while automated inventory tracking and shelf monitoring support operations. Retailers use deep learning models like CNNs, Vision Transformers, and multi-modal AI for shoppable images, counterfeit detection, and defect analysis.
Real-life example:
Pinterest Lens is a visual discovery tool integrated into the Pinterest app that allows users to search for ideas and products using their device’s camera.
By pointing the camera at an object and capturing its image, Lens analyzes the visual elements to suggest related items, ideas, or inspirations available on Pinterest. This feature is particularly useful for exploring fashion styles, home décor, and recipes based on real-world objects.3
Conclusion
While image recognition and image classification are related and often use similar techniques, they serve different purposes and have distinct applications.
Understanding the differences between these two processes is essential for harnessing their potential in various areas. By leveraging the capabilities of image recognition and classification, businesses and organizations can gain valuable insights, improve efficiency, and make more informed decisions.
Comments
Your email address will not be published. All fields are required.