Computer vision (CV) is revolutionizing almost every industry. However, successful computer vision development and implementation depend on high-quality image data. While some work with data collection services, others gather their data to train their computer vision systems.
See what image data collection is and how business leaders and developers can gather relevant image data:
What is image data collection for AI?
Image data collection for AI/ML training involves gathering and preparing images to be added to datasets that will train AI/ML algorithms.
This can include images of people, animals, objects, locations, etc. For instance, a CV-based system for detecting the quality of fruits on a conveyor belt might require training on thousands of images. Such datasets can be large or small, depending on the scope of the project.
Here is a sample dataset for a quality control computer vision system that scans apples.
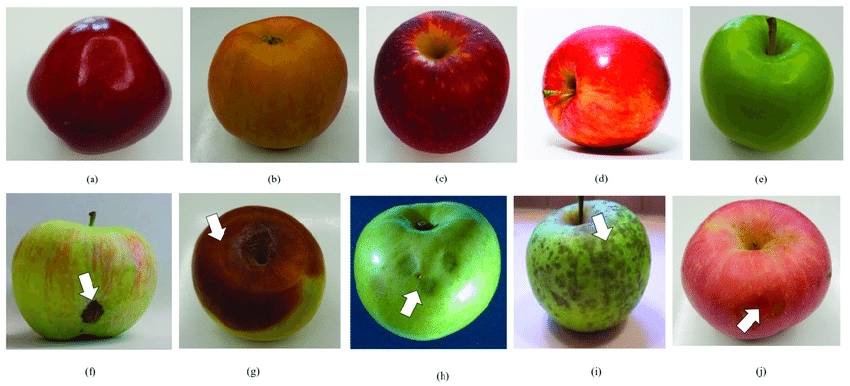
An image dataset for a facial recognition system might look something like this (only larger):
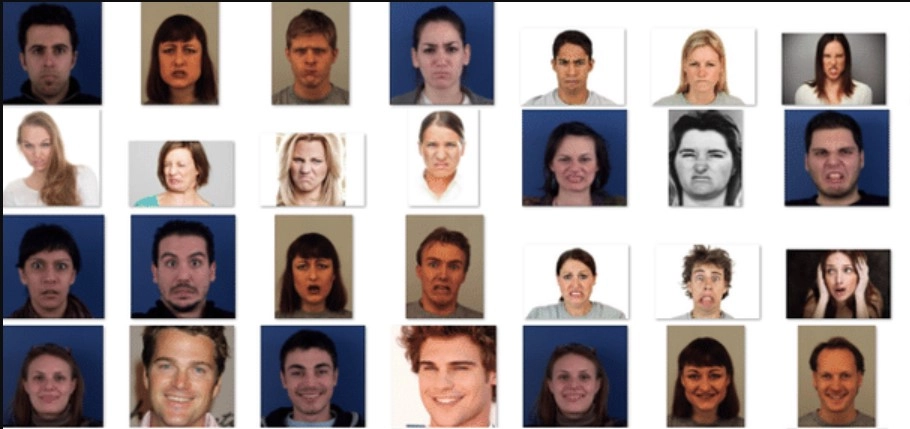
If you wish to work with an image data collection service, click here.
Challenges in image data collection
Gathering image data can be costly
A computer vision system requires a lot of images to be trained; however, this varies with the project’s scope.
According to a study, a facial recognition system with 300K and 400K images will have significantly higher accuracy levels than those trained with 100K and 200K (See Figure 1).
However, collecting datasets of this magnitude, as helpful as they might be, requires expensive cameras and an additional workforce.
Figure 1. Facial recognition accuracy with different dataset sizes
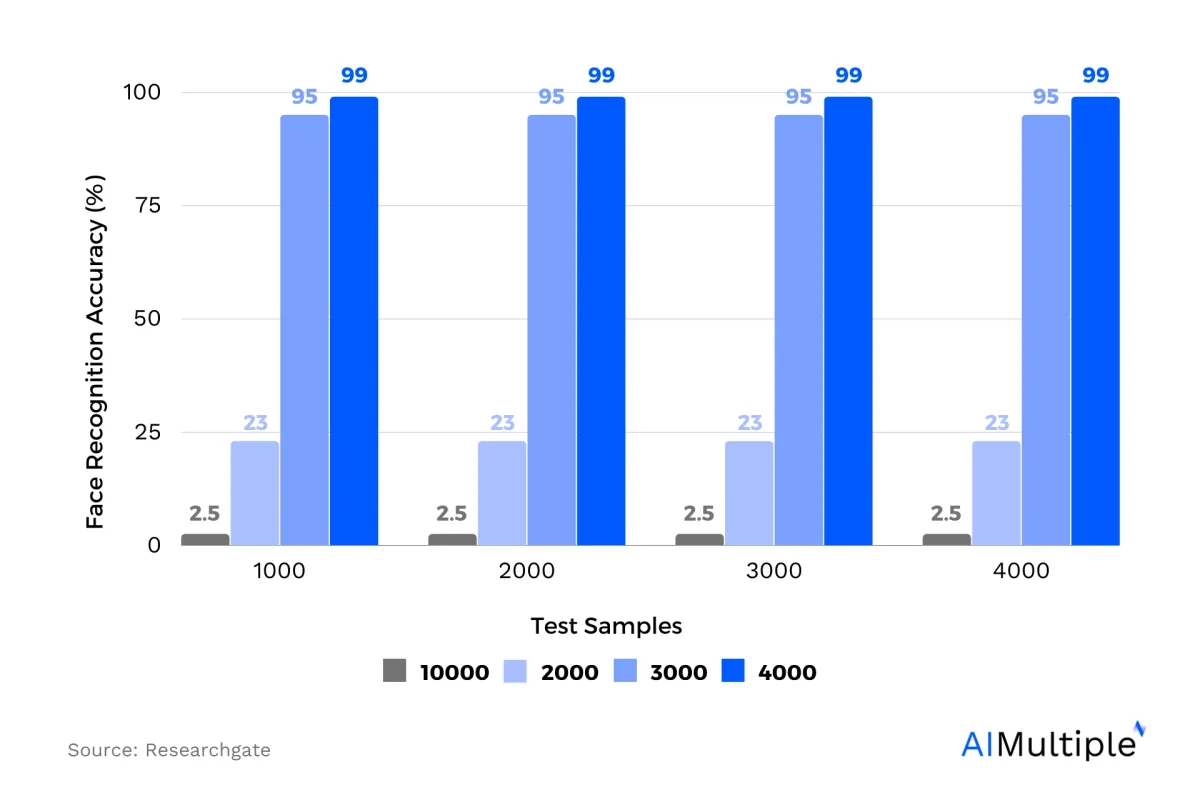
For instance, a facial recognition system being deployed in different countries will require data from the population of those specific countries. If this is done in-house, it can raise the project’s budget to unreasonable heights.
Gathering images can have ethical and legal constraints
Gathering images can sometimes have ethical and legal considerations attached to them. For instance, a facial recognition system might require face data for training., However, since face images are biometric data, they can be difficult to gather and use.
Other biometric image data that CV systems can gather includes fingerprint images, retina scans, etc.. If companies do not follow ethical and legal considerations, they can incur expensive lawsuits.
Watch this video to learn about one of Facebook’s lawsuits on unethically collecting the user’s biometric data:
Gathering data of any sort can be biased
Another issue when collecting data is the risk of the dataset becoming biased. This unconscious bias is transferred from the collector to the dataset and then to the AI/ML model that’s being trained.
Best practices to consider while collecting image data
Leverage automation
Leveraging automation to collect image data can reduce bias because the data that will be collected will be random and with minimal prejudice and discrimination. You can program a bot or leverage an automated data collection application to add data to your existing dataset automatically.
However, this can create storage issues since the dataset will increase every time someone uses the program. In such a scenario, setting parameters to regulate the collection can be helpful.
Automation in itself can be another issue. As Jo and Gebru mention in their paper, “Taking data in masses without critiquing its origin, motivation, platform, and potential impact results in minimally supervised data collection.”
Leverage Crowdsourcing
This can be another solution to overcoming the challenges mentioned earlier. Since the crowdsourcing model works with data collectors worldwide through a micro-job mechanism, the collected data can be diverse.
Crowdsourcing to collect data is a way to bypass AI bias.
Respect the ethical and legal considerations
In our data collection ethics article, we list the following best practices:
- Providing ethical training to all data collection staff and obtaining the consent of the person providing the image data
- Ensuring that the consent given is explicit, clear, and understandable
- Ensuring that the ethical considerations are consistent with all the data providers
- The data provider has control over its usage of the images
Ensure consistency
Image data consistency is essential in determining the model’s performance level. If 500 images need to be in front of a green background, then that should be the case for all of them.
Ensure quality
The quality of the overall image dataset is evaluated through its:
- Relevance to the scope of the project
- Comprehensiveness so that it can cover all requirements of the AI/ML model
- Authenticity and validity
To learn more about data collection quality assurance, check out this quick read.
You can also check our data-driven list of data collection/harvesting services to find the option that best suits your needs.
Image data collection use cases
1. Healthcare
Hospitals use federated learning to train diagnostic AI models on decentralized MRI/X-ray datasets without sharing sensitive patient data. Synthetic datasets (e.g., AI-generated tumor scans) supplement rare disease cases.
- Tools:
NVIDIA Clara, Owkin.
2. Retail
Virtual try-on systems require hyper-diverse datasets covering 50+ body types, skin tones, and cultural apparel. Retailers use 3D body scans from in-store kiosks and crowdsourced selfies (with consent).
- Tools:
Vue.ai is used for personalized styling datasets, and Zeekit (acquired by Walmart) is used for real-time AR try-ons.
3. Agriculture
Drones equipped with multispectral cameras capture crop health data (RGB + infrared), fused with soil moisture sensors for precision farming. Farmers share anonymized data via agricultural data cooperatives to train community AI models.
4. Traffic management system
Traffic management systems collect anonymized CCTV feeds and scans from autonomous vehicles to optimize routes. Privacy is maintained via edge processing, data anonymized on-device before transmission.
- Tools:
NVIDIA Metropolis for video analytics.
5. Manufacturing
Quality control systems use ultra-high-resolution thermal cameras to detect micro-cracks in materials. Synthetic defect data simulates rare production-line failures.
- Tools:
Cognex VisionPro and Siemens Synthetic Defect Generator.
Comments
Your email address will not be published. All fields are required.