The increasing usage of cloud services and collaboration between companies to monetize data raises concerns over data privacy. Regulations such as the General Data Protection Regulations (GDPR) aim to protect consumers’ privacy, and businesses pay serious fines in case of non-compliance. This creates a tradeoff between data privacy and utility for companies.
Homomorphic encryption is an emerging technology that can help businesses to ensure the privacy of their customers without undermining their ability to gain insights from their data.
What is homomorphic encryption?
Homomorphic encryption (HE) is a type of encryption method that allows computations to be performed on encrypted data without first decrypting it with a secret key. The computation results also remain encrypted and can only be decrypted by the owner of the private key.
How does homomorphic encryption work?
The image below demonstrates how homomorphic encryption works:
- The process starts with data in its decrypted form (i.e., plain text). The owner of the data wants some other party to perform a mathematical operation (e.g., some function, or a machine learning (ML) model) on it without revealing its content.
- The owner encrypts the data with a public key and sends it to the other party.
- The other party receives encrypted data (i.e., cipher text), performs operations on it, and sends the encrypted result to the owner.
- The data owner decrypts it with a private key and reveals the result of the intended mathematical operation, which is identical to the result if the unencrypted data had been used.
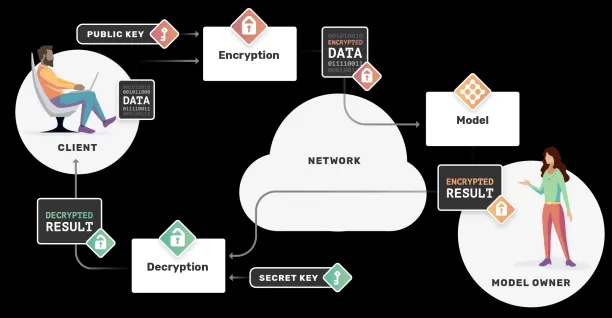
Apple’s implementation of homomorphic encryption:
Apple has integrated homomorphic encryption into its ecosystem to enhance user privacy while delivering enriched on-device experiences. By implementing the Brakerski-Fan-Vercauteren (BFV) HE scheme, Apple enables computations such as dot products or cosine similarity on encrypted data, which are common in machine learning workflows.
This approach allows devices to perform private server lookups without revealing the query content, supporting features like private database lookups and machine learning tasks.
Apple has open-sourced a Swift-based HE library to facilitate broader adoption, encouraging developers to implement similar privacy-preserving techniques in their applications.1
What are the different types of homomorphic encryption?
There are three main types of homomorphic encryption:
- Partially Homomorphic Encryption (PHE): PHE only allows selected mathematical functions to be performed on encrypted data.
- Somewhat Homomorphic Encryption (SHE): SHE allows a limited number of mathematical operations up to a certain complexity to be performed, for a limited number of times.
- Fully Homomorphic Encryption (FHE): FHE allows any kind of mathematical operation to be performed for an unlimited number of times.
These categories are distinguished based on the kind and frequency of mathematical computations that can be executed on the ciphertext.
Why is homomorphic encryption important now?
Sharing private data sets with third parties, such as cloud services or other companies, is challenging due to data privacy regulations such as GDPR and CCPA. Failure to comply with these regulations can lead to serious fines and damage to business reputation.
Traditional encryption methods provide an efficient and secure way to store sensitive data in an encrypted form on cloud environments. However, to perform computations on data encrypted with these methods, businesses either need to decrypt the data on the cloud, which can lead to security problems, or download the data, decrypt it, and perform computations, which can be costly and time-consuming.
Homomorphic encryption (HE) enables businesses to securely share private data with third parties to obtain computational services. With HE, the cloud service provider or outsourcing company has access only to encrypted data and performs computations on it. These services then return the encrypted result to the owner, who can decrypt it with a private key.
What are the benefits of homomorphic encryption?
- Allows secure and efficient cloud use: Homomorphic encryption allows businesses to securely leverage cloud computing and storage services. It eliminates the tradeoff between data security and usability. Businesses don’t have to rely on cloud services regarding the security of their private data while retaining the ability to perform computations on it.
- Enables collaboration: HE enables organizations to share sensitive business data with third parties without revealing the data or the computation results to them. This can accelerate collaboration and innovation without the risk of sensitive information getting compromised.
- Ensures regulatory compliance: HE can allow businesses operating in heavily regulated industries, such as healthcare and finance, to get outsourcing services for research and analytical purposes without the risk of non-compliance.
How to implement homomorphic encryption?
Some popular open-source libraries for implementing homomorphic encryption schemes include:
Check Homomorphic Encryption Standardization’s relevant section for more details on different tools.
What are the challenges to homomorphic encryption?
Partial and somewhat homomorphic encryption systems have existed since the late 70s but a fully homomorphic encryption scheme that allows all mathematical operations on encrypted data was first established in 2009 by Craig Gentry. In its current form, fully homomorphic encryption is impractically slow.
It can be said that FHE schemes are still emerging techniques for data security and utility. But it’s a promising one and we are likely to see faster versions of it that can be applied to a variety of use cases.
In 2017, Researchers from organizations such as Microsoft, IBM, Google, government organizations and academia have formed a an open consortium to standardize homomorphic encryption and to advance secure computation.
What are alternatives to homomorphic encryption?
As businesses can collect large volumes of data that often contain personally identifiable information (PII) of customers, data privacy is likely to remain a hot topic. There are other cryptography algorithms and privacy-preserving technologies that allow businesses to leverage analytical methods without privacy and non-compliance risks.
While homomorphic encryption offers unique advantages, it exists alongside several other privacy-preserving technologies, each with distinct characteristics:
Secure Multi-Party Computation (MPC)
Allows multiple parties to jointly compute a function over their inputs while keeping those inputs private
Advantages: Often faster than FHE; can handle complex computations
Limitations: Requires communication between parties; network latency impacts performance
Ideal for scenarios involving multiple data owners who need to collaborate without revealing individual inputs
Differential Privacy
Adds calibrated noise to data or query results to protect individual records while preserving statistical validity
Advantages: Mathematically provable privacy guarantees; works with plaintext data
Limitations: Involves a privacy-utility tradeoff; each query consumes a “privacy budget”
Ideal for statistical analysis and ML training where approximate answers are acceptable
Zero-Knowledge Proofs
Allows one party to prove to another that a statement is true without revealing any information beyond the validity of the statement
Advantages: Provides verification without disclosure; minimal computational overhead for verifier
Limitations: Typically designed for verification rather than general computation
Ideal for authentication and verification scenarios where proof of knowledge is required
Federated Learning
Trains machine learning models across multiple devices or servers holding local data samples without exchanging the data itself
Advantages: Keeps raw data on local devices; works at scale across distributed systems
Limitations: Limited to machine learning applications; potential vulnerability to inference attacks
Ideal for scenarios involving large user bases and where model training is the primary goal
Each technology serves different needs in the privacy landscape, and they can often be combined for enhanced protection. For instance, homomorphic encryption might be used within a federated learning system to provide additional security guarantees.
For a comprehensive account of privacy-enhancing technologies (PETs), check our article on the topic.
To learn more about cybersecurity best practices, you can read our Top 9 Cybersecurity Practices for Corporations article.
If you need a cybersecurity solution in your business, take a look at our cybersecurity software and services hub.
If you still have questions about homomorphic encryption or other privacy-enhancing technologies, we would like to help:
Comments
Your email address will not be published. All fields are required.