The $28 billion healthcare analytics market1 is transforming how providers, payers, and life sciences organizations compete, and companies that move now can seize the advantage.
By delivering solutions that drive predictive care, reduce costs, and optimize operations, analytics unlocks new revenue streams and strengthens customer loyalty in a healthcare industry racing toward data-driven performance.
Learn the top 10 operational, financial, and clinical healthcare analytics use cases, plus the main integration challenges healthcare managers face.
1. Detecting fraud risk
Healthcare fraud (HCF) can occur through deceitful practitioners, organized schemes, or honest billing mistakes.
Fraud in the healthcare industry, whether through billing schemes or pharmacy-related issues such as forged prescriptions, poses significant financial risks. Healthcare analytics tools can detect anomalies across billing patterns, prescription usage, and other high-risk areas, helping to prevent significant financial losses.
Identity theft prevention through predictive analytics
Identity theft and patient fraud can pose important risks to both healthcare providers and patients. By integrating health informatics with biometric verification systems, healthcare organizations can analyze patient data for inconsistencies, such as mismatched addresses or service histories, and flag suspicious claims.
Leveraging healthcare data analytics supports healthcare organizations to detect and mitigate fraud by:
- Analyzing claim patterns across different insurance policies or insurers,
- Detecting upcoding (e.g., services that are unnecessary in light of the diagnosis),
- Discovering duplicate and phantom billing: A phantom bill is a claim submitted by a physician to the government to reimburse them for services they did not provide. Unveiling phantom billing is done by comparing patient claims with prior medical history.
This approach helps empower healthcare providers to ensure financial integrity while enhancing operational efficiency through insights derived from data analysis.
Real-life example:
The LexisNexis Intelligent Investigator provided a health plan used to detect fraud in a neurologist’s excessive prescribing of a drug for a rare neurological disorder.
By analyzing large datasets and identifying suspicious patterns in the doctor’s prescribing practices, the health plan was able to uncover fraudulent activities, tighten authorization rules, and prevent further misuse of funds.2
2. Ensuring patient data security
In healthcare analytics, ensuring data security is critical due to the sensitive nature of patient data and compliance requirements like HIPAA (Health Insurance Portability and Accountability Act). Leveraging analytics can help organizations proactively defend against security threats in the following ways:
Monitoring network traffic changes and detecting suspicious cyber-attack behavior: By analyzing real-time network traffic and user behavior, healthcare analytics can quickly detect abnormal patterns that may signal cyberattacks. These insights help identify unauthorized access, unusual data transfers, and suspicious system interactions, enabling timely intervention.
Calculating real-time risk scores for specific transactions: In healthcare, not all transactions carry the same level of risk. Analytics can help assign real-time risk scores to specific transactions, such as data access or financial transactions, based on various factors such as the sensitivity of the data, the user’s location and device, and historical transaction patterns.
By assigning dynamic risk scores, healthcare systems can implement adaptive security protocols. For example, high-risk transactions might trigger additional authentication steps, or in some cases, restrict access altogether.
Check out AI cybersecurity to learn more.
3. Forecasting patient loads
Healthcare analytics tools are also helps organizations to forecast patient loads. This involves using predictive analytics to estimate how many patients will visit each department at specific times, based on historical clinical data, population health trends, and seasonal patterns.
Here’s how this approach supports healthcare providers in enhancing both patient care and operational efficiency:
Managing resources (physicians and supporting staff)
By using healthcare data analytics to predict patient volumes, hospitals can ensure that the right number of physicians, nurses, and supporting staff are scheduled during peak times. This helps:
Improving patient outcomes
By ensuring that the right resources are available when needed, patient wait times can be reduced, which can lead to better patient outcomes. Faster access to care also improves the quality of treatment plans and enhances the overall patient experience.
Additionally, forecasting patient loads aids in disease prevention by ensuring that adequate staff and resources are allocated during public health crises, such as flu outbreaks. This can have a significant impact on population health.
Reducing unnecessary labor costs
Predicting patient volumes helps hospitals optimize staffing by ensuring that medical staff are available during peak times while avoiding overstaffing during slower periods. This balance improves both patient care and financial sustainability.
Real-life example:
The public university hospital in Paris utilized Intel’s analytics platform to predict patient visits to the emergency department. The analytics platform allowed the users to view 15-day predictions of ER visits for optimizing staffing availability based on anticipated needs.3
4. Monitoring real-time healthcare data
By using healthcare analytics tools, organizations can continuously monitor patient conditions and resources to improve patient outcomes and operational efficiency. Here’s how real-time monitoring can enhance healthcare:
Preventing adverse events
Real-time data analysis helps prevent adverse events by continuously monitoring patient data both in the hospital and at home. With health informatics, healthcare providers can detect critical changes in patient conditions which would allow for faster intervention.
Constantly analyzing data from in-hospital and in-home patient devices
In modern healthcare, many patients use wearables or other devices to track vital signs, whether they are in the hospital or at home. By continuously analyzing this data, healthcare providers can:
- Monitor patient health remotely, detecting issues such as irregular heartbeats, oxygen level drops, or other critical changes that may require immediate attention.
- Prevent critical events by alerting staff to anomalies in patient data that may indicate emergencies, such as a sudden drop in oxygen levels.
- Optimize staff response times by delivering insights to healthcare professionals, enabling them to prioritize care based on real-time patient data.
- Improve patient outcomes by catching early warning signs of complications or deterioration in real time.
Real-life example:
Enghouse’s VirtualSitter solution addresses nursing shortages and patient safety, particularly in geriatric, ICU, and behavioral health settings. By enabling remote observation of up to 16 patients from a single screen, it helps prevent falls, reduce burnout, and improve patient care.
The solution also allows staff to categorize patients by risk level and monitor them continuously. It supports cost savings by reducing the need for 1:1 sitters and integrating with existing hospital systems.
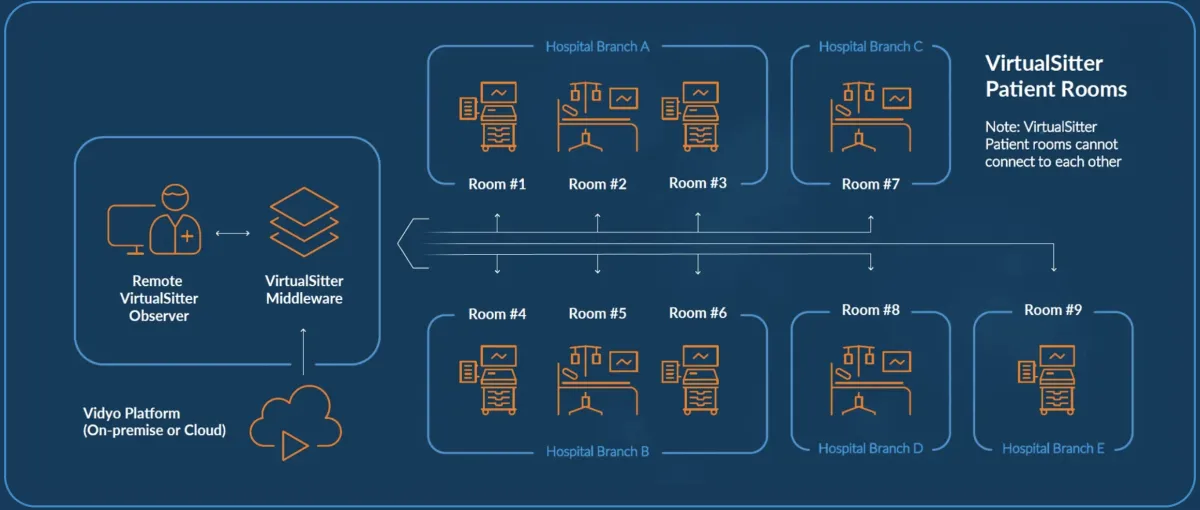
Figure 1: Enghouse’s VirtualSitter diagram for patient rooms.4
Allowing better resource planning
Real-time data monitoring provides crucial information for hospital administrators to optimize resource planning. By tracking the number of patients, staff availability, and the capacity of medical facilities in real time, healthcare organizations can ensure smooth operations and high-quality care.
Real-life example:
Netsmart’s predictive analytics tool supports skilled nursing facilities in improving outcomes related to MDS (Minimum Data Set) and quality measures. It offers real-time insights to enhance MDS accuracy, predict quality ratings, and Five-Star outcomes.
The tool also optimizes PDPM (Patient-Driven Payment Model) and allows facilities to benchmark data, spot negative trends early, and automate MDS workflows. This supports better decision-making and enhances regulatory compliance.5
Real-life example:
Origami Risk’s Patient Safety & Quality solution helps healthcare organizations manage incidents, conduct root cause analysis, and improve patient safety through features such as automated reporting, safety huddles, and patient experience tracking.
The solution supports proactive risk assessments and regulatory compliance for healthcare operations. It also includes tools for conducting surveys, peer reviews, and monitoring policy adherence, to enable continuous improvement in patient safety.6
Other than operational and financial use cases, there are several clinical use cases. Most clinical use cases rely on patient data, including family history, smoking habits, health conditions, and other patients’ outcomes and data:
5. Predicting disease outcomes & 6. Treatment plans
Predictive analytics leverages patient history, genetics, and past treatment outcomes to forecast disease progression and recommend personalized care plans, improving both disease management and treatment effectiveness. This approach allows:
- Identify high-risk patients: Flagging those with conditions like heart disease for closer monitoring.
- Forecast disease trajectories: Predicting complications in conditions like diabetes to enable early interventions.
- Personalized care: Tailors treatment to individual needs for better outcomes.
- Improved treatment effectiveness: Predicts which therapies are most likely to succeed.
7. Predicting the benefits of certain drugs
Healthcare analytics predicts how patients will respond to medications by analyzing clinical data and outcomes. It helps:
- Determine the best drug options: Recommending drugs based on patient demographics and conditions.
- Monitor drug effectiveness: Analyzing real-time feedback to assess if a medication works.
8. Prescription auditing
Data analytics ensures medication safety by auditing prescriptions. It can analyze prescribing patterns to ensure that medications follow clinical guidelines.
9. Tracking patient prescriptions and refills
Healthcare analytics tracks prescriptions and refills to ensure medication adherence. It can help:
- Monitor compliance: Identifying patients who miss refills for timely intervention.
- Improve patient care: Ensuring adherence reduces complications and hospital readmissions.
10. Identifying the patient’s risk of substance abuse
Predictive healthcare analytics also identifies patients at risk of substance abuse by analyzing prescription history and lifestyle factors. It can:
- Flag high-risk patients: Identifying those with multiple prescriptions or a history of abuse.
- Support decision-making: Providing insights for alternative treatments to prevent dependency.
Common data sources for healthcare analytics
Analytics in healthcare is only as valuable as the data it draws from. To generate valuable insights, healthcare organizations rely on a variety of healthcare data sources that fuel data analytics and enable data-driven decision making. Below, we break down the key sources that shape the healthcare analytics landscape.
Electronic Health Records (EHRs)
At the core of modern healthcare delivery are Electronic Health Records (EHRs), which serve as digital repositories of patient data. EHRs capture a patient’s medical history, including diagnoses, allergies, medications, prescriptions, laboratory results, radiology images, treatment plans, and test outcomes. This clinical data enables healthcare providers and medical professionals to track and improve patient care, contributing to better health outcomes.
EHRs are vital for health data analytics because they provide historical and real-time updates. Predictive and prescriptive analytics models often leverage EHR data to anticipate disease risks, optimize treatment strategies, and reduce hospital readmissions.
Healthcare data analysts apply advanced analytics, such as machine learning, to EHR datasets to surface actionable insights that enhance clinical decision support systems and inform population health management strategies.
Administrative and operational data
Beyond medical records, administrative data and operational data from healthcare facilities are essential in data analysis. This includes appointment scheduling, billing, insurance claims, resource utilization, staffing, and supply chain management. These data streams help healthcare management teams and healthcare leaders improve operational efficiency, lower costs, and manage clinical workflows.
By applying descriptive analytics and diagnostic analytics to administrative datasets, healthcare organizations gain a deep understanding of bottlenecks such as patient wait times, resource shortages, and system inefficiencies. This enables professionals and healthcare data analytics teams to implement solutions that enhance operational efficiency and improve the patient experience.
Research outcomes and clinical trials
Another valuable source of health data comes from published research outcomes, clinical trials, and academic studies. These sources provide insights into the efficacy of new treatments, emerging therapies, and best practices in disease prevention and healthcare services.
Integrating this third-party data into internal analytics pipelines helps healthcare professionals and medical services teams stay at the forefront of innovations and apply evidence-based interventions.
When combined with proprietary data from the healthcare ecosystem, research findings offer valuable insights that guide health education, improve patient satisfaction, and strengthen health informatics systems. Healthcare industry leaders often use these insights to craft data-driven insights for improving health outcomes across populations.
Population and environmental health data
Population health efforts require the inclusion of social determinants of health, environmental exposures, and demographic data. These factors, such as income, education, housing, and local environmental conditions, significantly impact patient and health outcomes. Including environmental factors in analytics allows healthcare systems to address health disparities, design targeted interventions, and support disease prevention programs.
By analyzing this unstructured data alongside clinical records, healthcare data analysts can deliver business insights and public health recommendations that improve population health management strategies.
Wearables, remote monitoring, and patient-generated data
With the rise of digital health tools, healthcare organizations increasingly tap into data from wearables, fitness trackers, and remote monitoring devices. These sources provide continuous raw data streams on heart rate, blood pressure, glucose levels, physical activity, and sleep patterns.
By integrating this healthcare data into data warehousing systems, healthcare providers can apply big data analytics and data visualization techniques to monitor chronic conditions, tailor treatment strategies, and deliver predictive analytics that help improve patient outcomes.
Claims and financial data
Lastly, payer data, including insurance claims, cost records, and reimbursement data, offers crucial insights into healthcare utilization and spending patterns. Analyzing this information enables business analytics teams to uncover cost-saving opportunities, optimize billing, and enhance healthcare services delivery.
By combining clinical and financial datasets, healthcare analytics teams can conduct systematic analysis to identify gaps, reduce hospital readmissions, and generate data-driven insights that strengthen the healthcare system’s financial sustainability.
Challenges in integrating healthcare analytics
The key challenges in data analytics in healthcare include:
Diversity in data formats
Typically, different medical facilities such as hospitals, pharmacies, and private clinics use different medical software and data formats, which makes data comparison, analysis, transfer, and sharing more difficult.
Data storage
The massive size of healthcare data, as well as the different types of structured and unstructured data, increases the cost of storage.
Data technologies and staff
Medical organizations need to purchase analytics products and solutions. Alternatively, to handle healthcare data, specialized tech teams, including data scientists and analysts, need to be hired, which can be quite costly depending on the size of the medical facility.
However, choosing the right analytics technology in healthcare is critical due to the uniqueness of healthcare data and its measurement difficulty.
Further reading:
- Top 10 Healthcare CRM Software
- Generative AI Healthcare: 15 Use Cases, Challenges & Outlook
- Healthcare APIs: Top 7 Use Cases & Challenges with Examples
- 17 Computer Vision in Healthcare Use Cases & Examples
External Links
- 1. Health big data analytics services market by application 2025 global forecast| Statista. Statista
- 2. Healthcare Billing Fraud Detection. LexisNexis Risk Solutions
- 3. https://www.intel.com/content/dam/www/public/us/en/documents/white-papers/french-hospital-analytics-predict-admissions-paper.pdf
- 4. Remote Patient Observation Solution | VirtualSitter | Enghouse Video. Enghouse Video
- 5. Predictive Analytics | Netsmart.
- 6. Patient safety & quality | Origami Risk. Origami Risk
Comments
Your email address will not be published. All fields are required.