Both data federation and virtualization can be beneficial to a company’s needs; however, knowing when to use which can be difficult.
Explore and compare data federation vs data virtualization approaches, their benefits and limitations, and how they can help companies improve their data integration, storage structure, and analysis.
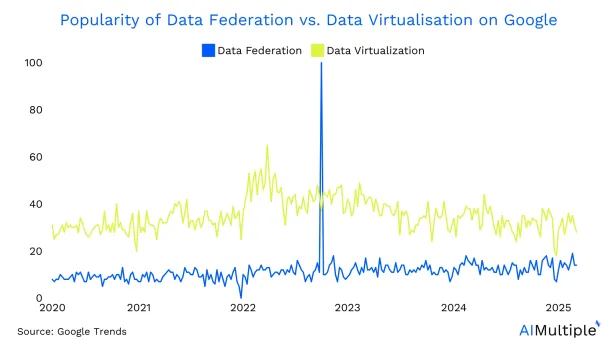
Why does it matter?
Data integration has enabled businesses to rapidly generate significant volumes of data. However, companies are now faced with the challenge of effectively consolidating data from disparate sources to derive meaningful insights and make informed decisions. Hence, effective data management became critical for businesses to stay competitive.
Data federation and data virtualization are two approaches to data integration that have gained popularity since the 2010s for effective data management. Nevertheless, they are less known compared to the traditional data integration approaches like ETL (extract, transform, load) (Figure 2). Unlike ETL, data federation and virtualization can facilitate access to data transformation methods. They can enable businesses to transform data on the fly as it is accessed, eliminate the need for complex ETL processes, and save time.
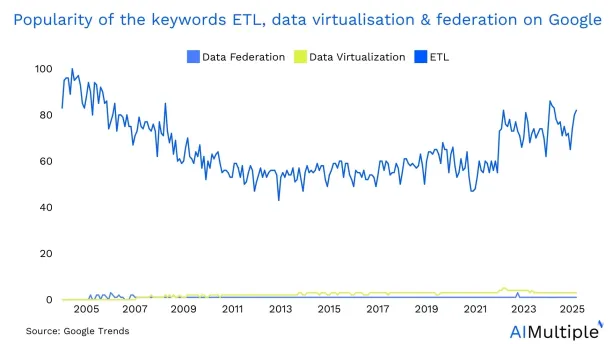
1. Data federation
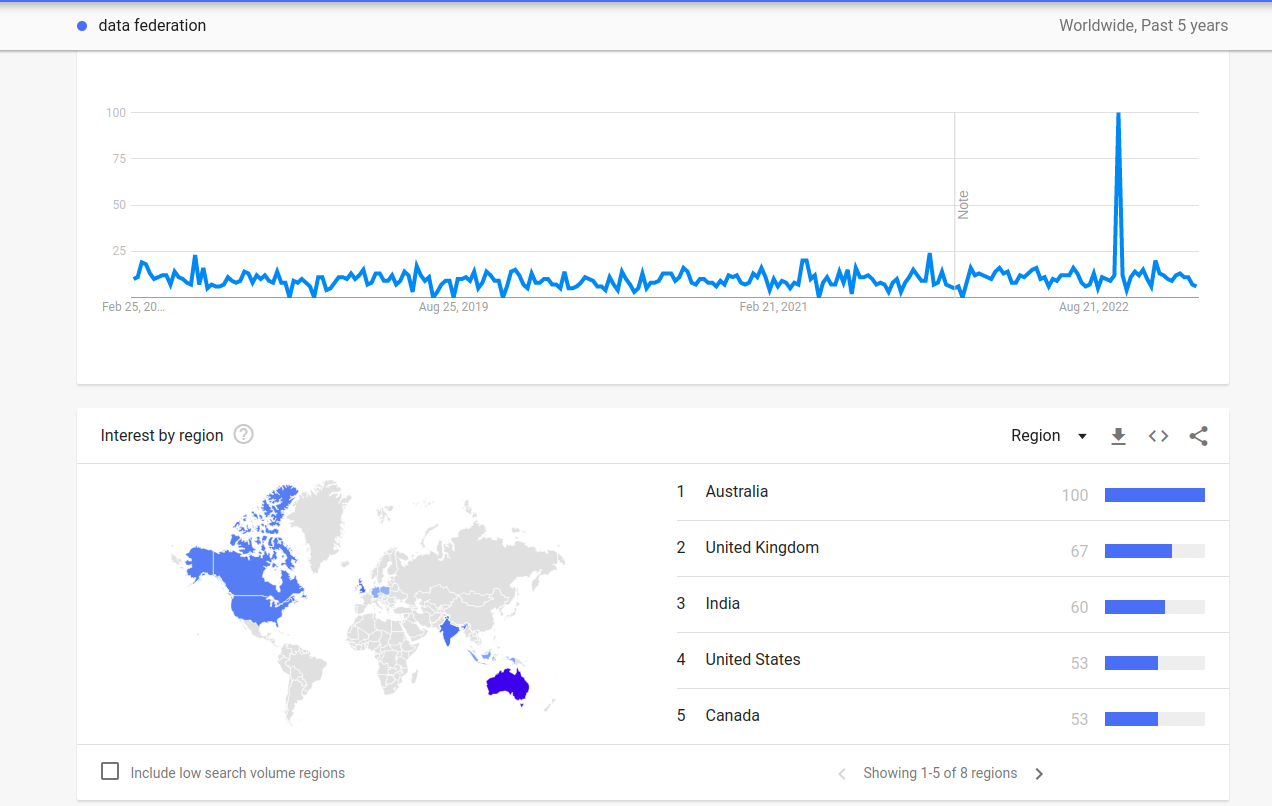
Figure 3. Interest in data federation.
Data federation is a data integration approach that enables businesses to access and query data from multiple sources as if they were a single source. It accomplishes this by using data integration techniques, constructing a layer on top of the disparate data sources.
In this layer, the data is abstracted from its complexities and presented to the data consumer as a unified view. Hence, the virtual data abstraction layer can be compared to a map that assists business users in navigating the various data sources. But, unlike data virtualization, in data federation, underlying data stores continue to operate independently without copying the data.
Advantages of data federation
- Provides a unified view of federated data from disparate sources.
- It simplifies data integration and reduces the need for manual data processing in data warehouses.
- Allows for real-time data access and querying.
Limitations of data federation
- Requires a high level of upfront planning and coordination to ensure the virtual layer accurately reflects the underlying data sources.
- Can be less performant than data virtualization for complex queries involving large data sets to support the virtualization layer.
2. Data virtualization
Data virtualization is a data integration strategy. It can enable businesses to access and query data from multiple sources without physically moving or copying the data. It builds a virtual and logical data layer on top of disparate data sources.
The first virtualized database layer abstracts technical data aspects from their complexities and presents them as a unified view. The data virtualization includes a layer that can be viewed as a window that shows users the various data sources.
Advantages of data virtualization
- Provides a unified view of data from disparate sources.
- It simplifies data integration and reduces the need for manual data processing.
- Can be more performant than data federation for complex queries involving large data sets.
Limitations of data virtualization
- Can be less performant than data federation for simple queries involving small data sets
- May require additional hardware resources to support the virtualization layer
Data virtualization is often used in scenarios where data sources are geographically distributed or where data volumes are large, making it difficult or costly to move data from its source to a central location. By virtualizing autonomous data stores, creating a more virtualized data layer on top of disparate data sources, data virtualization enables businesses to access and query data as if it were all in one place.
Comparison of data federation and data virtualization
Differences between data federation and data virtualization:
Difference | Data federation | Data virtualization |
---|---|---|
Virtual layer | Creates a virtual layer that abstracts the complexities of the underlying data sources | Leverages a virtual layer that abstracts the data sources’ physical location |
Upfront planning | Can necessitate more upfront planning and coordination to ensure that the virtual layer accurately reflects the underlying data sources | Necessitates less upfront planning |
Performance | Can be less performant for complex queries involving large data sets | Can be less performant for simple queries involving small data sets |
Table 1: Difference between data federation and data virtualization.
Advantages and disadvantages of each approach
- Data federation can provide real-time data access and querying capabilities, but it can require more resources to support.
- Data virtualization can be more scalable and easier to maintain, but it can require additional hardware resources to support.
How to choose between data federation and data virtualization
1. Consider the size and complexity of your data sources
It is critical to consider the size and complexity of your enterprise data sources when deciding between data federation and data virtualization. Data federation can be a better option if you have a small number of relatively simple and stable data sources.
If you have a large number of geographically distributed relational data stores or need to combine data from many different types of data sources, data virtualization can be a better option. In that case, data virtualization can be a better option in integrating relational data stores with web services.
2. Consider the level of performance required for your queries
The level of performance required for your queries on large data stores is another important factor to consider when deciding between data federation and data virtualization.
If you need to perform complex queries on large data sets, data federation can be a better option because it can often provide better performance for these types of queries. If you need to perform simple queries on small data sets, data virtualization can be a better option because it often provides better performance for these types of queries.
Data virtualization can be a better choice for simple queries on small data sets because it lets users access and query data from multiple sources without having to move or copy the data. This virtual data access means that data in virtual databases can be accessed and queried in real-time, without the delay that is often needed when moving data.
3. Consider the resources available to support your chosen approach
Finally, consider the resources available to support your chosen approach when deciding between data federation and data virtualization. Data federation can necessitate more hardware resources because it frequently involves consolidating data from multiple sources.
Data virtualization frequently involves creating a virtual layer on top of multiple data sources. Hence, it can necessitate more software resources. It can be critical to assess your organization’s resources and select an approach that can be supported within your budget and staffing constraints
4. Consider data integration approaches
The following table summarizes the factors to consider when choosing data federation or data virtualization.
Factors to consider | Data federation | Data virtualization |
---|---|---|
Size and Complexity of Data Sources | Better for a small number of relatively simple and stable data sources | Better for large, geographically distributed relational data stores or combining data from many different types of data sources |
Level of Performance Required for Queries | Better for complex queries on large data sets | Better for simple queries on small data sets |
Resources Required to Support Approach | May necessitate more hardware resources due to consolidating data from multiple sources | May necessitate more software resources due to creating a virtual layer on top of multiple data sources |
Table 2. Factors to consider when choosing data federation or data virtualization.
Use Cases
1. Use cases of data federation
Real-time monitoring
Suppose a utility company wants to monitor the performance of its power generation systems in real-time. They can use a data federation system to combine data from multiple sources, including Internet of Things (IoT) data like sensors and control systems, into a unified view. This can enable operators to monitor the performance of the systems and identify issues in real-time.
Risk management
Suppose a financial services company wants to assess its risk exposure across multiple portfolios. They can use data federation tools to combine data from multiple sources into one particular data store for data consumers.
This data context can include market data feeds and trading systems in a unified view. The unified view can enable risk managers to assess risk exposure and make informed decisions about portfolio management.
2. Use cases of data virtualization
Some of the data virtualization use cases include:
Sales reporting
Suppose a company with multiple sales systems desires a unified view of sales data across all systems. They can use data federation tools to build a virtual layer on top of sales systems. This can provide business users with a unified view of the data across multiple systems and allow them to generate reports to gain insights into overall sales performance.
Customer analytics
Suppose a retail company wants to gain insights into customer behavior and preferences by analyzing data from multiple sources. This can include point-of-sale systems, customer loyalty programs, and website analytics.
They can use data virtualization and data science tools to create a virtual layer on top of disparate data sources. The above virtualized data layer can enable them to easily access and analyze customer data.
Challenges and considerations
When implementing data federation or data virtualization technologies, businesses must take into account a range of challenges and considerations. These include:
1. Security and data governance considerations:
Since the 2020s, there has been a greater interest in data security (Figure 5). Last year, the average cost of a data breach in the U.S. was ~$9.44 million.1 Specifically, data integration can pose security risks if not properly managed.
Businesses must ensure that their data integration solutions are secure, compliant with regulations, and adhere to data governance policies. The implementation of privacy-enhancing technologies and data masking methods can also help protect sensitive information during data integration.
The use of synthetic data is also beneficial in reducing risks by providing anonymized datasets for testing and analysis, as shown by various synthetic data use cases.
2. Performance and scalability considerations
Data integration solutions must be designed and optimized to provide the optimal performance and scalability that the business requires. This includes ensuring that the solution can handle large amounts of data as well as complex queries. Utilizing data quality tools and data cleaning processes can improve the accuracy and reliability of integrated data.
3. Cost considerations
Data integration can be costly, particularly when dealing with large or complex data sets. Businesses must evaluate the costs of implementing and maintaining a data integration solution and ensure that it aligns with their budget and ROI expectations.
For more technical details on data virtualization and data federation, please contact us at:
Comments
Your email address will not be published. All fields are required.