Data augmentation techniques generate different versions of a real dataset artificially to increase its size. Computer vision and natural language processing (NLP) models use data augmentation strategy to handle with data scarcity and insufficient data diversity.
Data-centric AI/ML development practices such as data augmentation can increase accuracy of machine learning models. According to an experiment, a deep learning model after image augmentation performs better in training loss (i.e. penalty for a bad prediction) & accuracy and validation loss & accuracy than a deep learning model without augmentation for image classification task.
Data augmentation techniques in computer vision
There are geometric and color space augmentation methods for images to create image diversity in the model. It is easy to find many coding examples for these augmentation transformations from open-source libraries and articles on the topic.
Adding noise
For blurry images, adding noise to the image can be useful. By “salt and pepper noise”, the image looks like consisting of white and black dots.
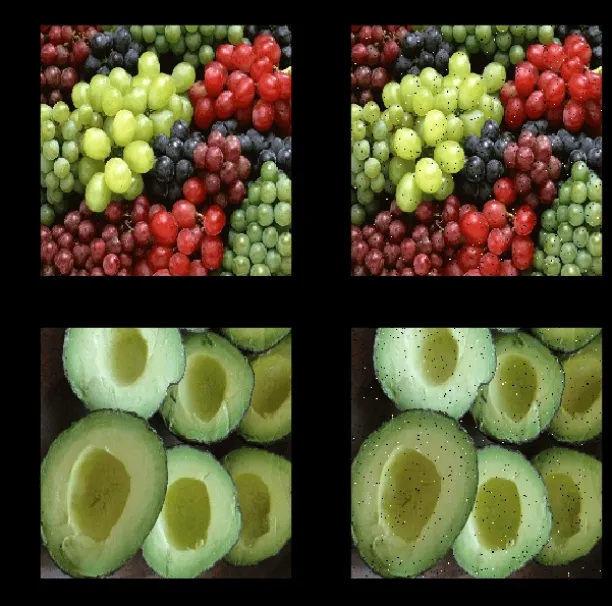
Cropping
A section of the image is selected, cropped, and then resized to the original image size.
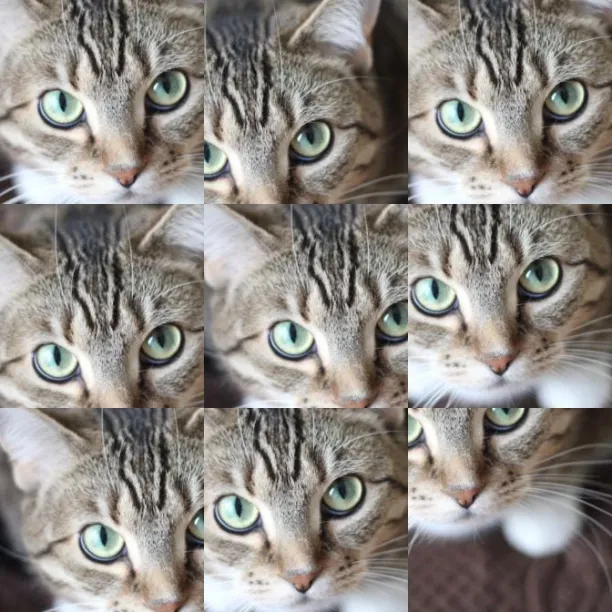
Flipping
The image is flipped horizontally and vertically. Flipping rearranges the pixels while protecting the features of the image. Vertical flipping is not meaningful for some photos, but it can be useful in cosmology or for microscopic photos.
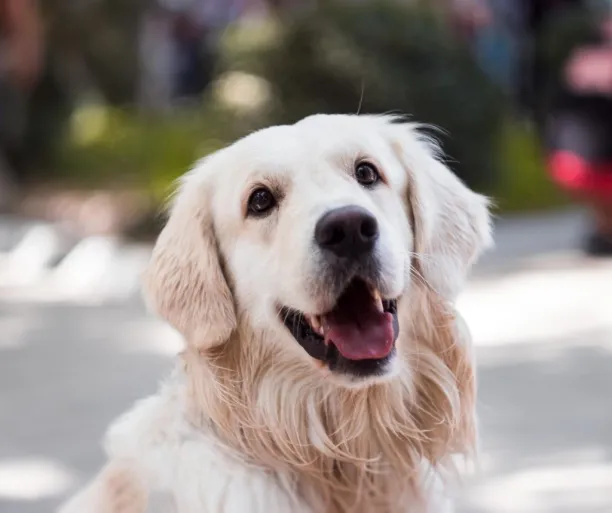
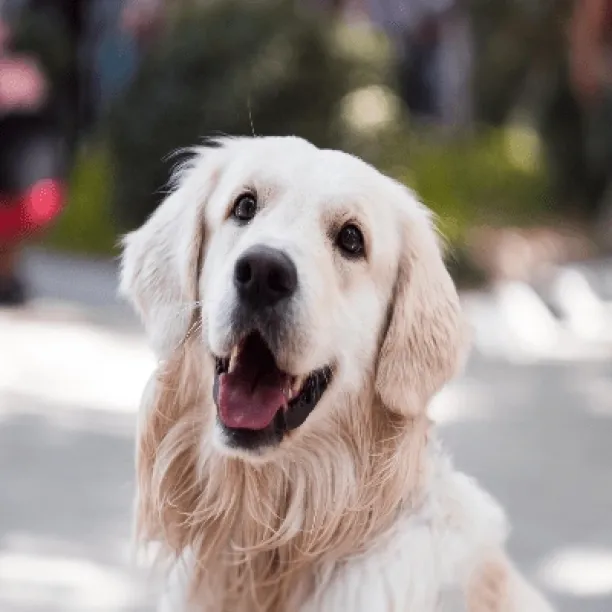
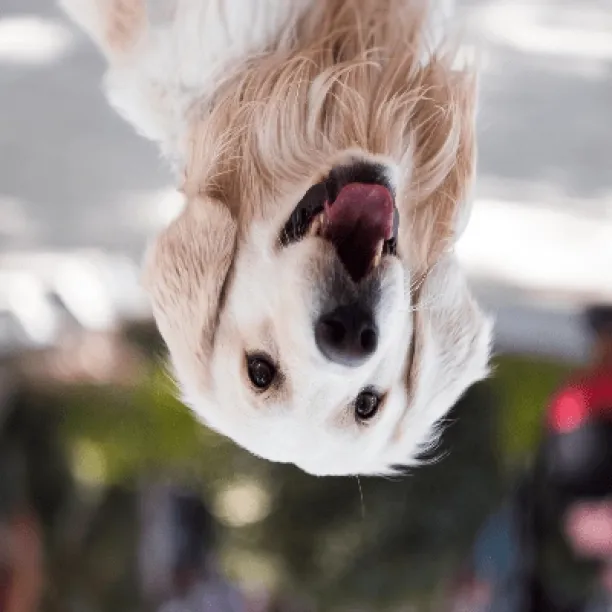
Rotation
The image is rotated by a degree between 0 and 360 degrees. Every rotated image will be unique in the model.
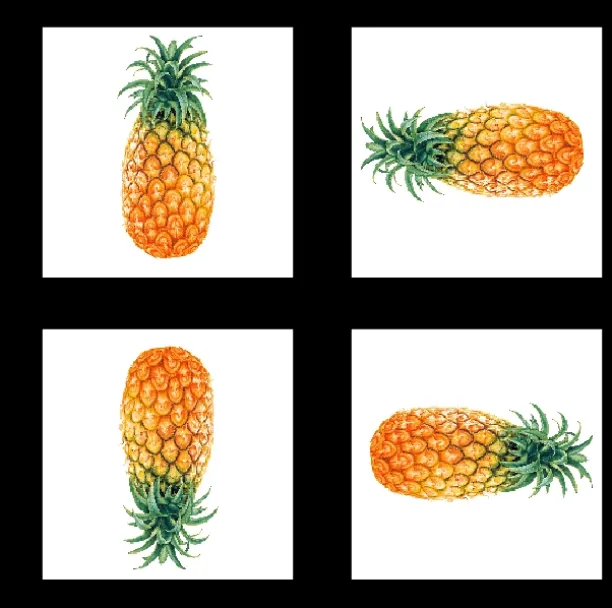
Scaling
The image is scaled outward and inward. An object in a new image can be smaller or bigger than in the original image by scaling.
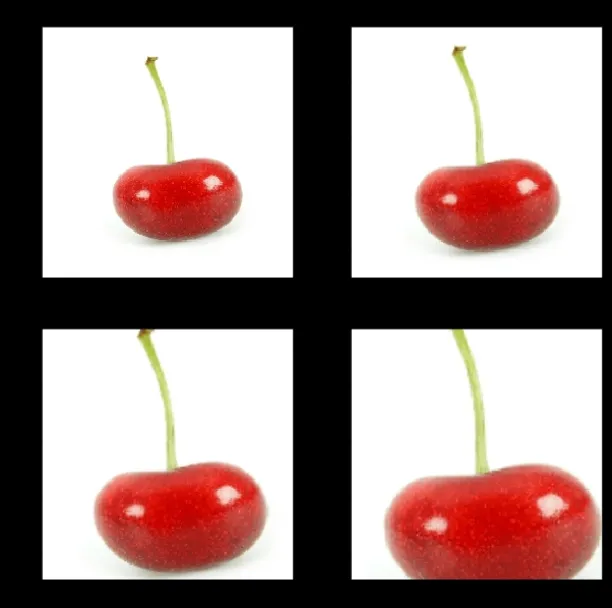
Translation
The image is shifted into various areas along the x-axis or y-axis, so the neural network looks everywhere in the image to capture it.
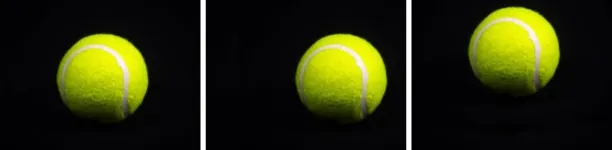
Brightness
The brightness of the image is changed and the new image will be darker or lighter. This technique allows the model to recognize images in different lighting levels.
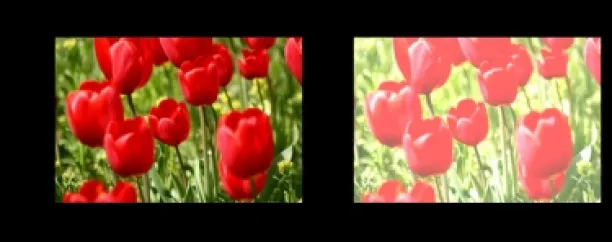
Contrast
The contrast of the image is changed and the new image will be different from luminance and color aspects. The following image’s contrast is changed randomly.
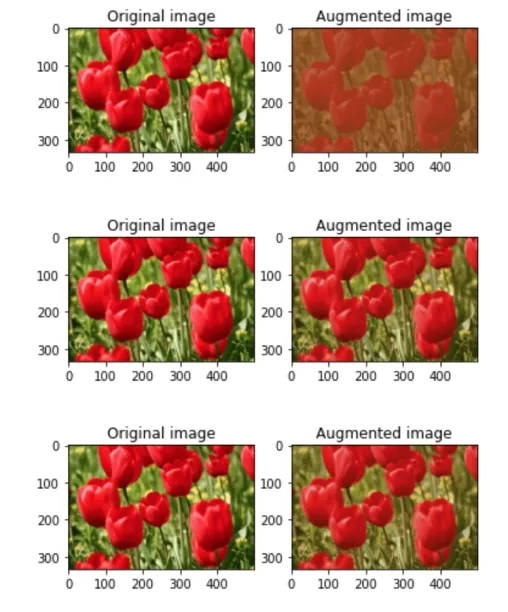
Color Augmentation
The color of the image is changed by new pixel values. There is an example image which is grayscale.
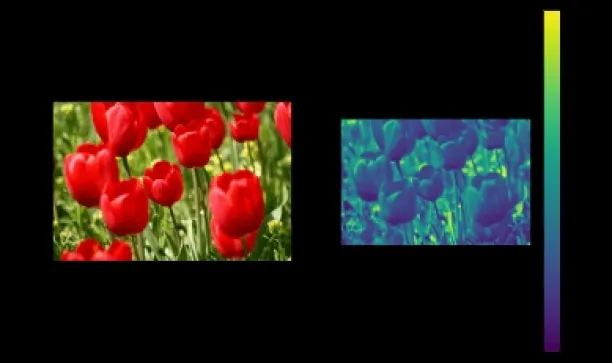
Saturation
Saturation is the depth or intensity of color in an image. The following image is saturated with the data augmentation method.
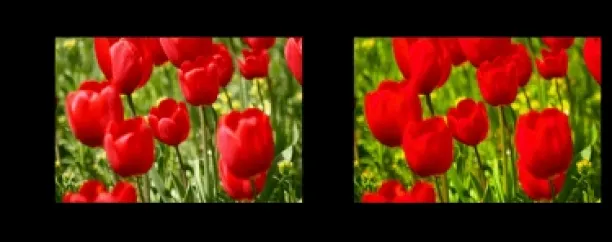
You can also check check our article on synthetic data for computer vision.
Data augmentation techniques in natural language models
Data augmentation techniques are applied on character, word, and text levels.
Easy Data Augmentation (EDA) Methods
EDA methods include easy text transformations, for example, a word is chosen randomly from the sentence and replaced with one of this word synonyms, or two words are chosen and swapped in the sentence. EDA techniques examples in NLP processing are
- Synonym replacement
- Text Substitution (rule-based, ML-based, mask-based and etc.)
- Random insertion
- Random swap
- Random deletion
- Word & sentence shuffling
Back Translation
A sentence is translated into one language and then a new sentence is translated again in the original language. So, different sentences are created.
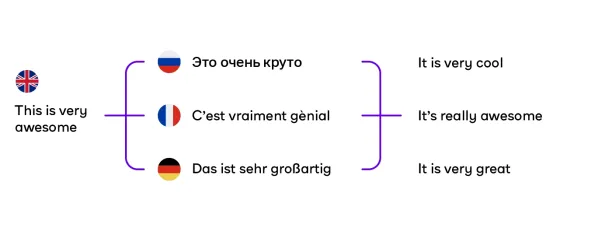
Text Generation
A generative adversarial network (GAN) is trained to generate text with a few words.
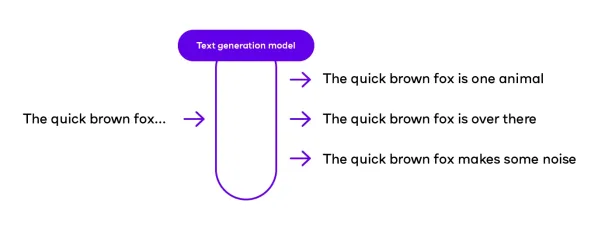
Developers can optimize natural language models by training them on web data that contains large volumes of human speech, languages, syntaxes, and sentiments.
Data augmentation techniques for audio data
Audio data augmentation methods include cropping out a portion of data, noise injection, shifting time, speed tuning changing pitch, mixing background noise, and masking frequency.
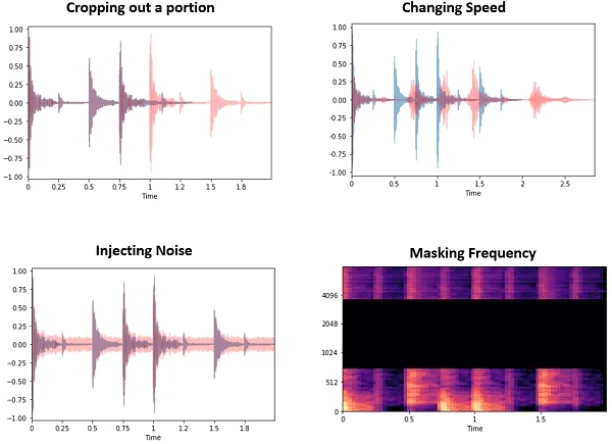
Advanced data augmentation techniques
Advanced data augmentation methods are commonly used in the deep learning domain. Some of these techniques are
- Adversarial training
- Neural style transfer
- Generative adversarial networks (GANs) based augmentation
For more, feel free to read our articles on deep learning data augmentation and GANs for synthetic data.
Data augmentation libraries
There are libraries for developers, such as Albumentations, Augmentor, Imgaug, nlpaug, NLTK, and spaCy. These libraries include geometric transformation & color space transformation functions, Kernel filters (i.e. image processing function for sharpening and blurring), and other text transformations.
Data augmentation libraries use different deep learning frameworks, for example, Keras, MxNet, PyTorc,h, and TensorFlow.
If you are ready to use data augmentation in your firm, we prepared data-driven lists of companies. However, we don’t yet have a list exclusively for data augmentation libraries yet. Most of the time, this functionality is provided as part of more comprehensive software packages (i.e. deep learning software):
If you need help in choosing vendors who can help you get started, let us know:
This article was drafted by former AIMultiple industry analyst Ayşegül Takımoğlu.
Comments
Your email address will not be published. All fields are required.