We analyzed ~20 AI governance tools and ~40 MLOps platforms that deliver AI governance capability to identify the market leaders based on quantifiable metrics. Click the links below to explore their profiles:
AI Governance Tools | Tool type | |
---|---|---|
1. | AI Governance | |
2. | Data Governance | |
3. | MLOps | |
4. | MLOPs | |
5. | LLMOps |
Compare AI governance software
AI governance tools landscape below shows the relevant categories for each tool mentioned in the article. Businesses can select solutions from these categories based on their AI initiatives and governance needs.
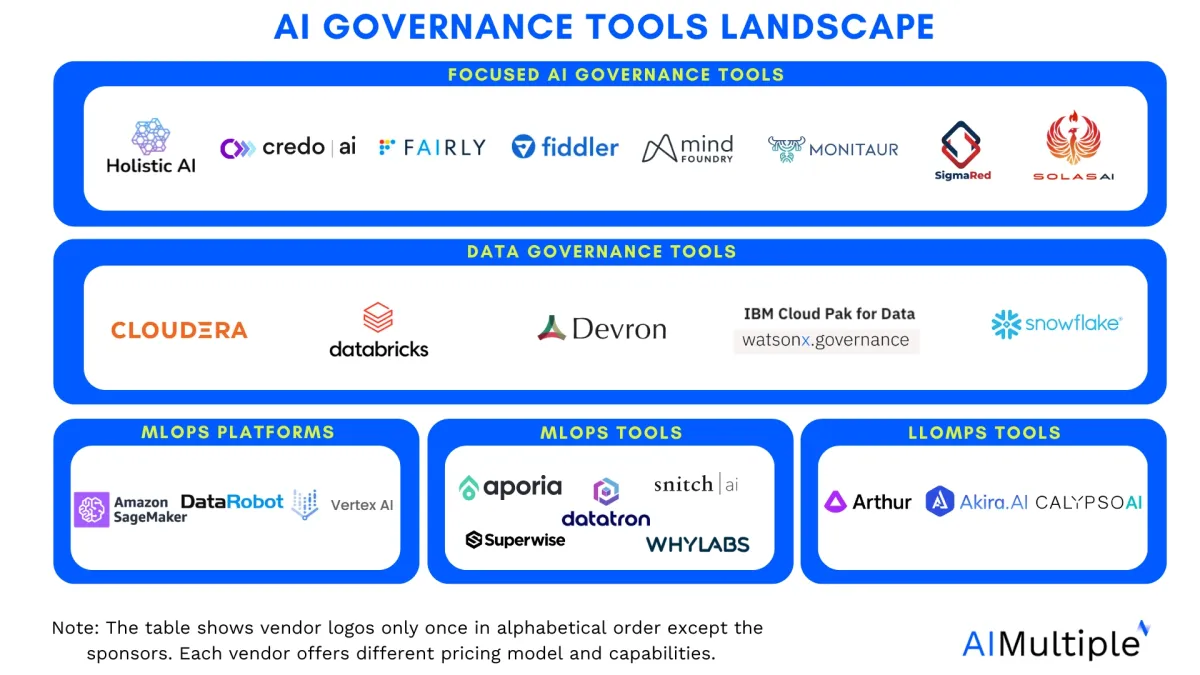
Top AI governance platforms
These tools tend to focus on an aspect of AI governance, unlike platforms that manage the entire AI lifecycle. Such tools can be useful for small-scale projects or best-of-breed approaches.
For example, they can focus on ensuring that AI systems comply with responsible AI best practices, industry regulations and security standards. They help organizations mitigate AI risk by:
- Implementing security measures
- Staying in line with regulatory requirements and laws
- Managing model documentation.
Some of these tools include:
Holistic AI
Holistic AI is a governance platform that helps enterprises manage AI risks, track AI projects and streamline AI inventory management. It can help users assess systems for efficacy and bias and continuously monitor global AI regulations to keep their AI applications, such as LLMs compliant.
With Holistic AI, users can facilitate:
- Policy and risk management for policy implementation, incident control, and operational risk management.
- Auditing and compliance to environmental and disaster recovery standards.
- EU AI Act support to comply with EU AI regulations, allowing businesses to focus on core objectives while the platform handles regulatory complexities.
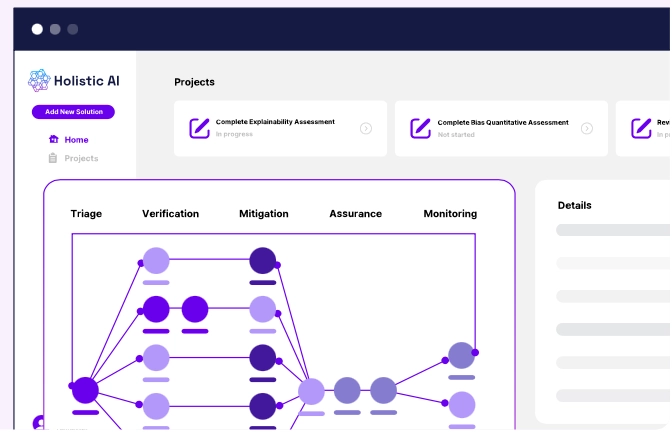
Anch.AI
Anch.AI offers a governance platform designed to help companies manage compliance, assess risks, and adopt ethical AI frameworks. The platform supports various stages of AI development and compliance with capabilities like:
- Risk assessment which refers to continuous monitoring of AI solutions for bias, vulnerabilities, and accountable roles.
- Risk mitigation to address individual risk exposures.
- Auditing and reporting to validate risk reduction and provides reporting on ethical AI performance.
- EU AI Act features, including an EU AI Act HealthCheck for assessing compliance levels and a deep-dive assessment for detailed analysis of regulatory alignment.
Credo AI
Delivers AI model risk management, model governance and compliance assessments with an emphasis on generative AI to facilitate the adoption of AI technology. Credo AI delivers:
- Regulatory compliance to streamline adherence to regulations and enterprise policies, including preparations for new laws like the EU AI Act.
- Risk mitigation to assess AI models for factors such as bias, security, performance, and explainability.
- Governance artifacts to generate AI-related documentation, including audit reports, risk analyses, and impact assessments.
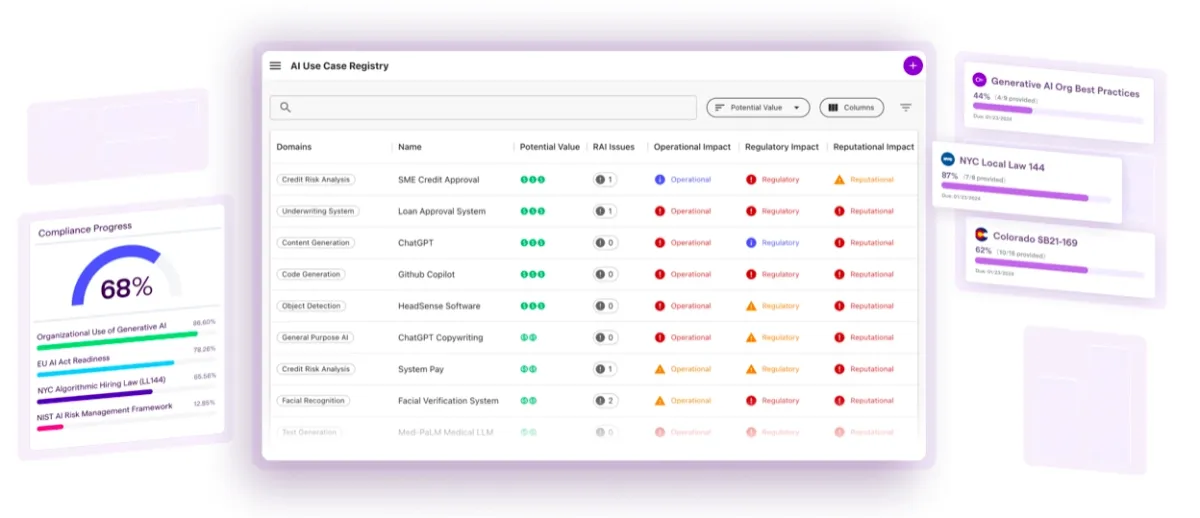
Fairly AI
Fairly AI can monitor, govern, and audit AI models to enhance compliance and reduce risks. It delivers capabilities like:
- Automated compliance to map regulations and policies to AI models for real-time monitoring and auditing.
- Cross-team collaboration to facilitate collaboration among policy, compliance, risk, and data science teams.
- Governance features to minimize team frictions and ensures automated oversight of AI model development.
Fiddler AI
An AI observability tool that provides ML model monitoring and relevant LLMOps and MLOPs features to build and deploy trustable AI, including generative AI.
Mind Foundry
Monitor and validate AI models, maintain transparency in decision-making, and align AI behavior with ethical and regulatory standards, fostering responsible AI governance.
Monitaur
Monitaur specializes in AI governance with its Monitaur ML Assurance platform, a SaaS solution for monitoring and managing AI models. The platform enables businesses to enhance oversight, improve collaboration, and implement scalable governance frameworks. Its key features include:
- Real-time monitoring: Tracks AI algorithms continuously and records real-time insights.
- Governance framework: Supports the creation of evidence-based, transparent AI governance programs.
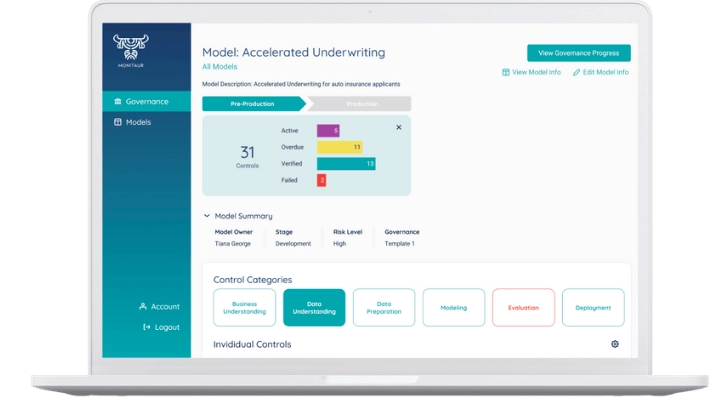
Sigma Red AI
Detects and mitigates biases, ensuring model explainability and facilitating ethical AI practices.
Solas AI
Checks for algorithmic discrimination to increase regulator and legal compliance.
Top data governance platforms
Data governance platforms contain various tools and toolkits primarily focused on data management to ensure the quality, privacy and compliance of data used in AI applications. They contribute to maintaining data integrity, security, and ethical use, which are crucial for responsible AI practices.
Some of these platforms can help check compliance and overall AI lifecycle management. These platforms can be valuable for organizations implementing comprehensive AI governance frameworks. Here are a few examples:
Cloudera
A hybrid data platform that aims to improve the quality of data sets and ML models, focusing on data governance.
Databricks
Combines data lakes and data warehouses in a platform that can also govern their structured and unstructured data, machine learning models, notebooks, dashboards and files on any cloud or platform.
Devron AI
Offers a data science platform to build and train AI models and ensure that models meet governance policies and compliance requirements, including GDPR, CCPA and EU AI Act.
IBM Cloud Pak for Data
IBM’s comprehensive data and AI platform, offering end-to-end governance capabilities for AI projects:
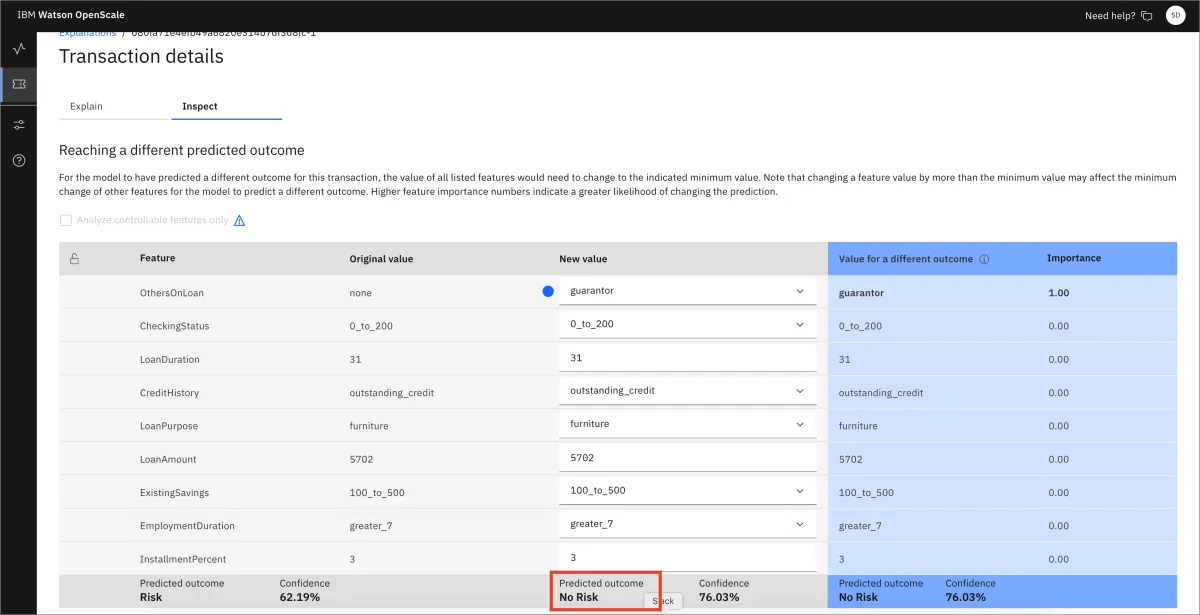
Snowflake
Delivers a data cloud platform that can manage risk and improve operational efficiency through data management and security.
Top MLOps platforms
Leading MLOps platforms provide tools and infrastructure to support end-to-end machine learning workflows, including model management and oversight.
Amazon Sagemaker
Amazon SageMaker is a managed AWS service that enables users to develop, train, and deploy machine learning models at scale. It simplifies the process of building, training, and deploying machine learning models, considering AI governance practices.
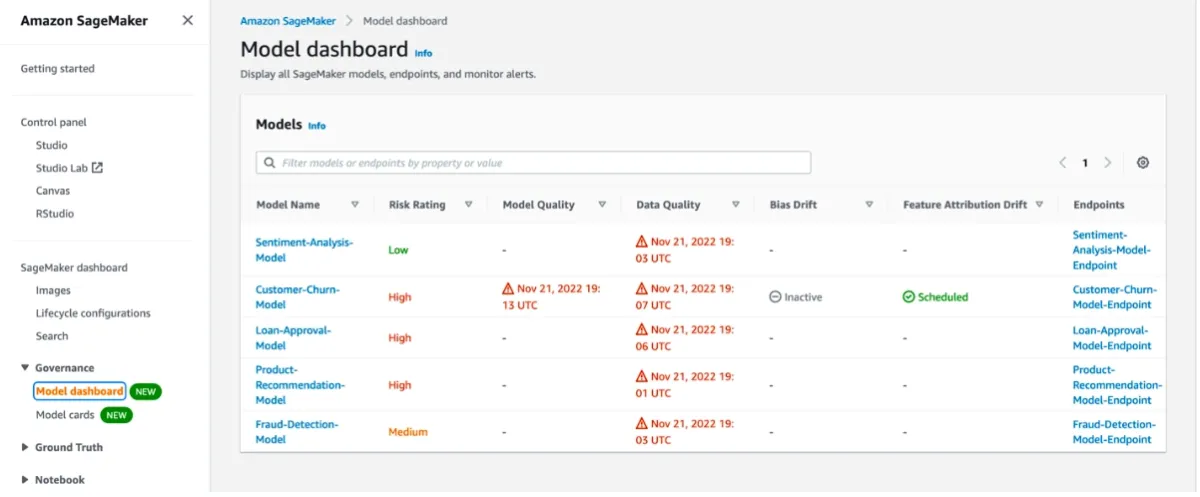
Datarobot
Delivers a single platform to deploy, monitor, manage, and govern all your models in production, including features like trusted AI and ML governance to provide an end-to-end AI lifecycle governance.
Vertex AI
Offers a range of tools and services for building, training, and deploying machine learning models with AI governance techniques, such as model monitoring, fairness, and explainability features.
Compare more MLOPs platforms in our data-driven and comprehensive vendor list.
Top MLOps tools
MLOps tools are individual software tools that serve specific purposes within the entire machine learning process. For example, MLOps tools can focus on ML model development, monitoring or model deployment. A data science team can deliver responsible AI products by applying these tools to machine learning algorithms to:
- Monitor and detect biasses
- Check for availability and transparency
- Ensure ethical compliance and data privacy.
Some of these tools include:
Aporia AI
Specialized in ML observability and monitoring to maintain the reliability and fairness of their machine learning models in production. It employs model performance tracking, bias detection, and data quality assurance.
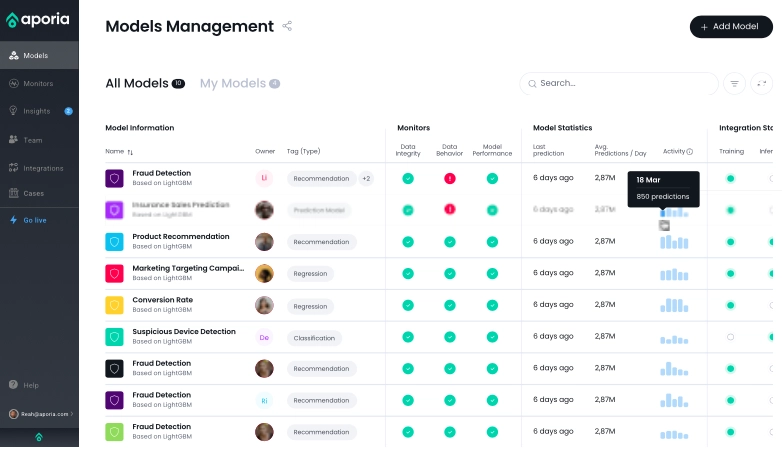
Datatron
Provides visibility into model performance, Enables real-time monitoring, and Ensures compliance with ethical and regulatory standards, thus promoting responsible and accountable AI practices.
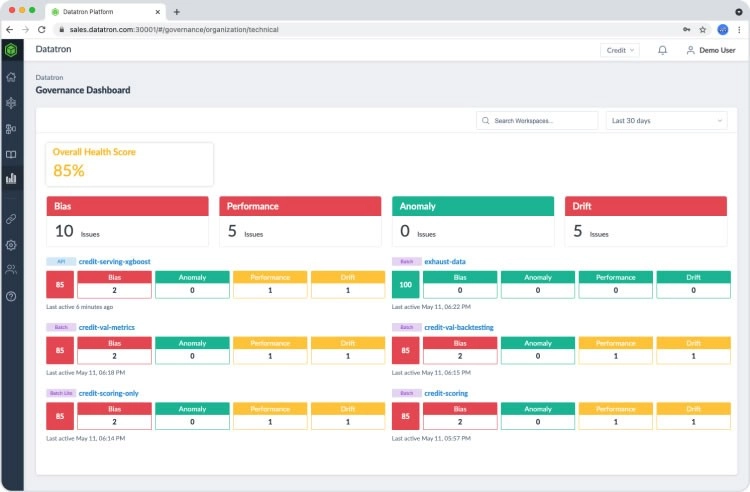
Snitch AI
An ML observability and model validator which can track model performance, troubleshoot and continuously monitor.
Superwise AI
Monitor AI models in real-time, detect biases, and explain model decisions, thereby promoting transparency, fairness, and accountability in AI systems.
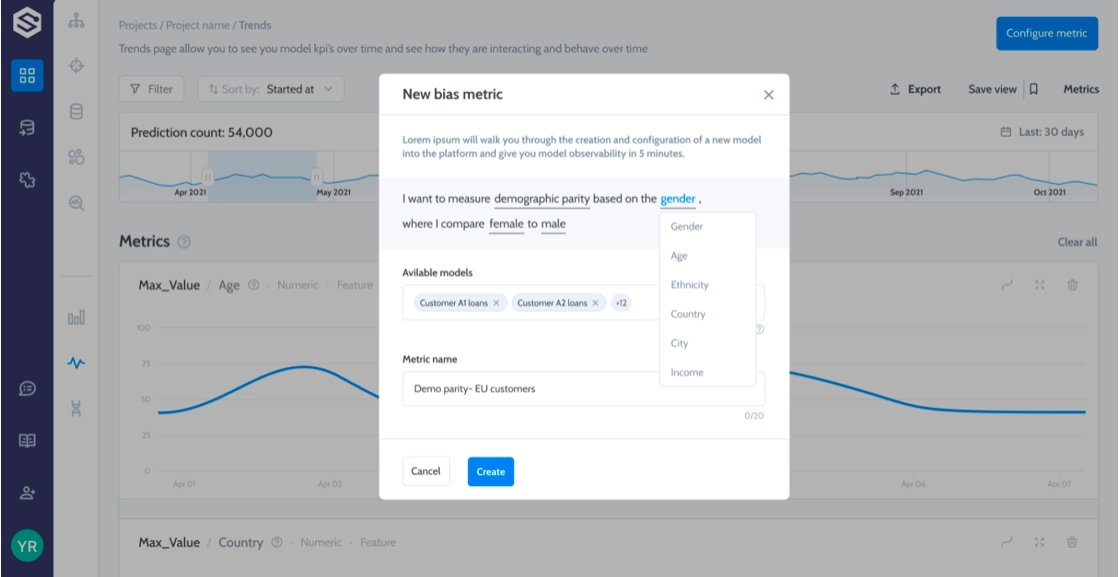
Why Labs
An LLMOps tool that monitors LLMs data and mode to identify issues.
Top LLMOps tools
LLMOps tools include LLM monitoring solutions and tools that assist some aspects of LLM operations. These tools can deploy AI governance practices in LLMs by monitoring multiple models and detecting biases and unethical behavior in the model. Some of them include:
Akira AI
Runs quality assurance to detect unethical behavior, bias or lack of robustness.
Calypso AI
Delivers monitoring considering control, security and governance over generative AI models.
Arthur AI
It tests LLMs, computer vision and NLPs (natural language processing) against established metrics.
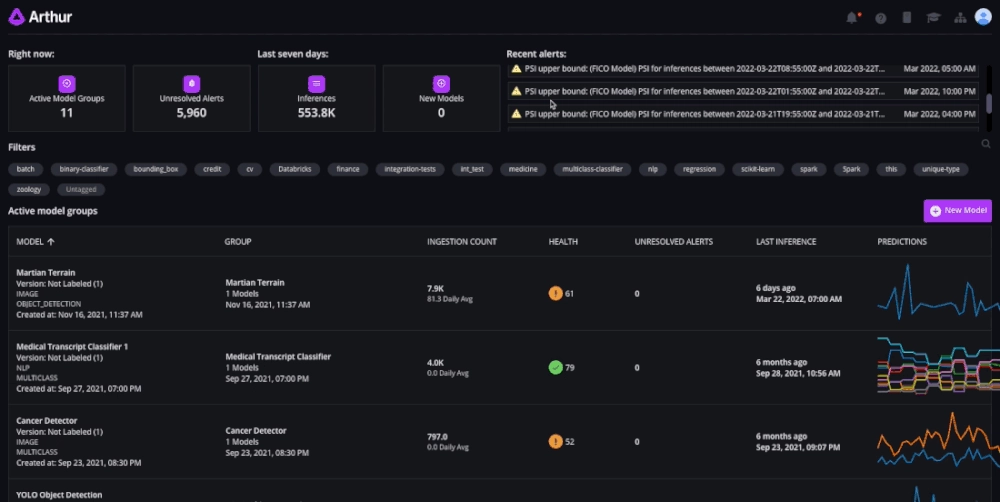
Compare more LLMOps tools in our data-driven and comprehensive vendor list.
What is AI governance & why is it important?
AI governance refers to establishing rules, policies, and frameworks that guide the development, deployment, and use of artificial intelligence technologies. It aims to ensure ethical behavior, transparency, accountability, and societal benefit while mitigating potential risks and biases associated with AI systems.
Ethical AI needs to be a priority for enterprises:
- EU AI Act came into force in August 2024. Some of its provisions are already enforced and all of them be enforced by 2026.
- AI is projected to power 90% of commercial applications by the close of 2025 (Source: AI stats).
These factors led to an increased interest in AI governance:
Data and algorithm biases can harm an enterprises’ reputation and finances, which can be prevented by adopting AI governance platforms. These tools help companies developing and implementing AI by improving:
- Ethical and responsible AI: Ensures AI systems are designed, trained, and used ethically, preventing biased or harmful outcomes. Learn more on ethical AI and generative AI ethics.
- Transparency and accountability: Promotes transparency in AI algorithms and decisions, making developers and organizations accountable for actions that AI systems take.
- Data privacy and compliance: Helps organizations comply with data privacy regulations like GDPR and HIPAA, ensuring that data is collected and used legally and ethically.
- Risk assessment and mitigation: Identifies and mitigates various risks associated with AI, including legal, financial, and reputational risks, before they lead to negative consequences.
- Fairness and equity: Identifies and addresses AI bias in AI models to promote equal treatment across diverse users and groups.
- Model performance and reliability: Continuously monitors AI models to maintain reliability by detecting model drift and performing model retraining as needed, reducing errors and improving user satisfaction.
- Public trust: Builds public trust in AI technologies by emphasizing ethical behavior and transparency.
- Alignment with organizational values: Allows organizations to align AI practices with their mission and values, demonstrating a commitment to ethics and responsibility.
- Discover more on AI compliance solutions.
- Competitive advantage: Ethical AI and responsible governance can provide a competitive edge by attracting customers, partners, and investors who value ethical AI solutions.
FAQ
Key AI governance techniques & features
AI governance software employs common techniques to streamline building and deploying AI/ML models, such as:
Explainability and interpretability: AI governance software employs visualizations and explanations for AI model outputs to provide insights into how AI models make decisions. These tools allow users to understand and predict complex model behavior.
Transparency and accountability: AI governance provides clear documentation of model training data and processes, which enables monitoring of model decisions for accountability.
Fairness and bias detection: AI governance practices mainly focus on identifying and quantifying biases in AI models and data. For example, AI governance tools can monitor model performance across different demographic groups, allowing to mitigate biases in real-time or during training. Two main ways to detect bias in the model is to ensure compliance with ethics and law:
Ethical AI compliance: AI governance primarily aligns AI behavior with ethics by implementing guidelines and constraints. As a result, a data scientist can customize AI behavior to avoid harmful and offensive outputs of AI systems.
Regulatory compliance: A major AI governance practice is to ensure adherence to legal and regulatory requirements, meet data privacy and security standards and help business users comply with industry-specific regulations.
Model lifecycle management: Once a model is ready, AI governance techniques can manage the deployment of the model in the production environment by monitoring models for drift, degradation, or unexpected behavior. Two features that can facilitate AI deployment include:
Model validation and testing: Some AI governance tools can contain model validator features to test and verify models against benchmark datasets. Deploy these tools before production to detect potential issues.
Model risk management: AI governance techniques provide insights to assess and mitigate risks for AI systems.
Continual monitoring and auditing: Another common practice is tracking the model performance in production and behavior to ensure compliance and reliability in AI systems.
How to select the right AI governance software ?
1. Identify your objective and scale: Consider the scale of your AI initiatives and the types of AI models and applications you are developing.
2. Research and evaluate available tools in the market:
– Look for vendors that specialize in the areas most relevant to your needs.
– Create a shortlist of promising tools based on their features, capabilities, and user reviews.
3. Benchmark the shortlisted tools based on the following:
– Each tool’s features: Assess its ability to detect bias, ensure data privacy, provide transparency, and monitor compliance.
– Ease of integration: Assess how well the AI governance tool integrates with your existing AI development and deployment pipeline.
– Compatibility with your organization: Check for compatibility with the programming languages, frameworks, and platforms you use for AI development. Ensure the tool can work seamlessly with your data sources, storage solutions, and cloud providers.
– User-friendly interface: How intuitive the tool is for seamless interaction.
– Customization and flexibility: The extent to which the tool can be customized to match your requirements, allowing you to adjust settings and configurations.
– Scalability: Consider the tool’s scalability to accommodate your organization’s growth in AI initiatives, such as increasing data volumes and workloads as your projects grow.
– Quality of vendor support: Investigate the level of customer support, response time and assistance provided.
– Training and resources: Review how comprehensive is the documentation, tutorials, user guides, online sources and training materials. Remember that adequate resources to help your team learn how to use the tool effectively.
– Cost and budget: Evaluate the cost structure of the AI governance tool, including licensing fees, subscription costs, and implementation expenses. Calculate the long-term costs and benefits of the tool to ensure it provides value over time based on your financial resources.
– Data security and privacy: Check compliance with data protection regulations, including encryption and access controls. Ensure the security and confidentiality of sensitive information.
3. Seek free trial and proof of concept (if applicable): Conduct a trial or proof of concept (PoC) with the selected AI governance software. You may use real or simulated AI projects to assess how well the tool addresses your governance needs. Involve key stakeholders, data scientists, and AI developers in the PoC to gather feedback on usability and effectiveness.
Disclaimers
This is an emerging domain, and most of these tools are embedded in platforms offering other services like MLOps. Therefore, AIMultiple has not had a chance to examine these tools in detail and relied on public vendor statements in this categorization. AIMultiple will improve our categorization as the market matures.
Products, except the products of sponsors, are sorted alphabetically on this page since AIMultiple doesn’t currently have access to more relevant metrics to rank these companies.
The vendor lists are not comprehensive.
Further reading
Explore more on AIOps, MLOps, ITOPs and LLMOps by checking out our comprehensive articles:
- Comparing 10+ LLMOps Tools: A Comprehensive Vendor Benchmark
- What is LLMOps, Why It Matters & 7 Best Practices
- Understanding ITOps: Benefits, use cases & best practices
- What is AIOPS, Top 3 Use Cases & Best Tools?
- MLOps Tools & Platforms Landscape: In-Depth Guide
Check out our data-driven vendor lists for more LLMOps tools and MLOps platforms.
If you still have questions and doubts, we would like to help:
External sources
- 1. Holistic AI - End to End AI Governance Platform.
- 2. Credo AI - The Trusted Leader in AI Governance.
- 3. AI Governance software that goes beyond good intentions | Monitaur.
- 4. IBM Developer.
- 5. Improve governance of your machine learning models with Amazon SageMaker | AWS Machine Learning Blog.
- 6. Aporia's Platform | Delivering Secure and Reliable AI. Aporia
- 7. MLOps Platform with Scale and Security | Datatron.
- 8. Model Observability Platform | Observe, Monitor & Improve ML. Superwise.ai
- 9. “Product dashboard.” Arthur AI. Revisited September 7, 2023.
Comments
Your email address will not be published. All fields are required.