What is Affective Computing / Emotion AI (2024)
As computers become more intelligent, they can now handle various manual tasks and understand humans better. Today, AI can recognize human emotions and predict mental states based on facial recognition, voice, and text analysis. In fact, machines could already identify pick up emotions better than humans from speech, according to research conducted in the 2000s. While the emotion AI technology continues to advance, its popularity and the market size are also growing rapidly. However, it comes with ethical concerns and criticism about its effectiveness is increasing.
What is affective computing?
Affective computing, also known as emotion AI, automatically recognizes emotions. Here is Wikipedia’s definition below:
Affective computing is the development of systems that can recognize, interpret, process, and simulate human feelings and emotions.
It may seem strange that machines can do something that is so inherently human. However, growing research supports the point that human emotions are recognizable using facial and verbal clues.
Understanding emotions is critical, especially for companies selling complex products. From those who are not working in customer-facing functions such as sales, marketing, or customer service, it may not be clear how affective computing is valuable for businesses. Emotions, guided by the unconscious mind, are likely to be the decision-makers in complex decisions. Furthermore, emotional, gut-based decisions can be better than conscious decisions when it comes to complex decisions.
Can computers really understand emotions?
People express emotions in surprisingly similar ways across cultures, and machines can pick up the visual and verbal clues of emotions.
A large body of research since the 1970s demonstrates that even pre-literate cultures with minimal exposure to literate cultures can identify basic emotional expressions such as anger, happiness, or surprise. There is also new, contradicting evidence supporting the theory that emotions are expressed individually in different ways, and this is an ongoing debate. However, despite the recent challenges, the theory that emotions are expressed in similar ways by different people is still widely accepted.
Machines are better than humans in identifying emotions from speech. Even in research conducted during 2003 and 2006, software achieved an accuracy of 70 to 80%. Human accuracy is ~60%.
Machines are already at acceptable levels in identifying emotions from facial expressions. In a 2017 study cited >30 times, researchers achieved a classification accuracy of 73% for seven emotional states with a relatively simple model using the Facial Action Coding System developed by Ekman, one of the pioneers in the field of facial expressions and emotions. However, this was achieved under strictly defined conditions using 3D Microsoft Kinect cameras. Additionally, experiment participants posed to create facial expressions; they did not naturally generate them. Despite these caveats, ~70% is a significant achievement.
Is the interest in emotion AI increasing?
Popularity
We have observed the last five years’ trend of both affective computing and emotion AI terms to analyze the popularity of the technology. As it has been a long time since this technology was first born, we observe a slightly decreasing popularity in affective computing. However, we observe an increasing trend for emotion AI, and the popularity of the term caught affective computing in 2020, with AI becoming a more popular term.
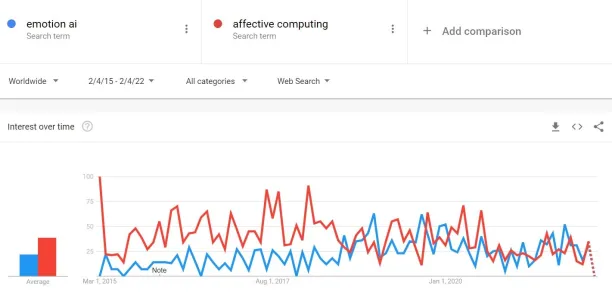
Market growth
According to market research conducted after coronavirus outbreak, the global affective computing market size will grow from $29 billion to $140 billion by 2025, at a compound annual growth rate (CAGR) of 37.4% during the forecast period. The increasing market growth is mostly due to the opportunities for measuring customer satisfaction. There are also other use cases like software testing, employee workload arrangement, and candidate emotion analysis during interviews, which are becoming popular. Feel free to read more about emotion AI use cases in our article.
What are the challenges with affective computing?
1. Limitations in algorithm design and hardware
The accuracy of affective computing is rising with the developments in algorithm designs. With the advances in technology, AI can identify emotions with new sources like blood volume pulse and facial electrography. However, it still requires further improvements in the algorithm design and more advanced hardware to be more widespread in real-life.
2. Ethical issues about increased surveillance
As more use cases of affective computing emerge, some use cases require video surveillance or social media monitoring to identify human emotions. While the main goal is to understand users’ mental states for better services, some people might not want to be monitored and their voices, images, or social media posts to be analyzed by affective computing software. The advances in emotion AI can bring some controversial ethical issues especially in some use cases like tracking employees during work, job interviews etc. Political campaigns’ use if sentiment analysis already proved deeply unpopular after the 2016 US presidential elections.
For more details, see our article about AI ethics.
3. Ethical issues about bias
Even if people are ok with estimates about their emotions being used for analytics, the results may have bias which is especially concerning in cases like job interviews. There has been research claiming that other AI techniques (e.g. facial recognition) have significant bias against underrepresented groups.
For more details, see our article about AI bias.
4. Challenges regarding results
Emotion AI using facial expressions relies on research about microexpressions by Paul Ekman and others which has recently come under more scrutiny. However, microexpressions are still accepted as valid signals for emotions by most scientists.
You can read more about affective computing in our related articles:
- Affective Computing: In-Depth Guide to Emotion AI
- Top 10 Emotional AI Examples & Reasons for Their Success
If you have questions about affective computing, don’t hesitate to contact us:

Cem has been the principal analyst at AIMultiple since 2017. AIMultiple informs hundreds of thousands of businesses (as per similarWeb) including 60% of Fortune 500 every month.
Cem's work has been cited by leading global publications including Business Insider, Forbes, Washington Post, global firms like Deloitte, HPE, NGOs like World Economic Forum and supranational organizations like European Commission. You can see more reputable companies and media that referenced AIMultiple.
Throughout his career, Cem served as a tech consultant, tech buyer and tech entrepreneur. He advised businesses on their enterprise software, automation, cloud, AI / ML and other technology related decisions at McKinsey & Company and Altman Solon for more than a decade. He also published a McKinsey report on digitalization.
He led technology strategy and procurement of a telco while reporting to the CEO. He has also led commercial growth of deep tech company Hypatos that reached a 7 digit annual recurring revenue and a 9 digit valuation from 0 within 2 years. Cem's work in Hypatos was covered by leading technology publications like TechCrunch and Business Insider.
Cem regularly speaks at international technology conferences. He graduated from Bogazici University as a computer engineer and holds an MBA from Columbia Business School.
To stay up-to-date on B2B tech & accelerate your enterprise:
Follow on
Comments
Your email address will not be published. All fields are required.