Enterprise search has been a game-changing technology in terms of increasing the productivity of organizations. Enterprise search engines combined with the power of a modern search engine with the company’s internal data.
With the advancements in personalization, machine learning, and Natural Language Processing (NLP), search engines of today are able to understand the context and respond to complex queries. In addition, search engine approaches are being combined with users’ actions to surface useful data for users even before they search for it. Such approaches will further increase the productivity of organizations.
Insights engines aim to bring modern knowledge discovery approaches to companies’ private data using the latest advances in technology. They aim to improve the knowledge discovery experience of end-users and the accuracy of data analysis by identifying the relevant data for each user. For more details:
What is an insight engine?
An insight engine, is also called cognitive search or enterprise knowledge discovery and management, is an enterprise platform that makes key enterprise insights accessible to the users when they need them. It combines search with machine learning capabilities to provide information for users and data for machines. The goal of an insight engine is to provide timely data that delivers actionable insights.
The term was first suggested by Gartner and defined as “Insight engines apply relevancy methods to describe, discover, organize and analyze data. This allows the existing or synthesized information to be delivered proactively or interactively, and, in the context of digital workers, customers or constituents, at timely business moments.”
Why is it important now?
Analysts expect insights engines to grow in importance
Experience shows that analyst expectations are anything but accurate. And most analyst estimates are impossible to be verified since they are either not specific, not measurable or impossible to validate with public data. However, they still include information about market expectations which point to increased interest in insight engines:
- Verified Market Research highlights that the Global Insight Engines Market was valued at USD 757.20 Million in 2018 and is projected to reach USD 4.14 Billion by 2026.
- Gartner stated that ”By 2020, 50% of analytical queries either will be generated via search, natural language processing or voice, or will be automatically generated.”
Drivers of interest in insight engines
Insights engines enable organizations to generate insights automatically from existing databases. Data-driven decision-making is increasing in popularity among managers and insights engines enable companies to base their decisions on insights extracted from data.
How does it work?
The insight engine proactively searches databases to deliver information when needed rather than start searching on-demand. Insight engines extract needed information from large volumes of complex (structured/unstructured) and diverse (internal/external) data sources.
Insights engines use a combination of technologies:
To extract information from the companies data and generate insights that will enhance decision making:
- Data integration: Insight engines needs to access companies’ data in various data sources and data integration technologies facilitate that.
- Natural Language Processing (NLP): NLP technology enables engines to understand natural language in unstructured data (text, video, image, etc.). This enables insights to be identified within the company’s data.
- Machine learning (ML): Data can be analyzed using machine learning algorithms to identify trends, make predictions and suggest recommendation.
To match insights with users’ search queries:
- ML Algorithms: With machine learning algorithms such as collaborative filtering, clusterization and similarity calculation, insight engines can identify relevance between search results and match insights to search queries.
- Semantically enhanced Logical Data Warehouse (LDW): Logical data warehouses store semantic information (information about the meaning of the stored data) along with the data itself. For example, this enables LDW systems to identify synonyms and deal with user search queries involving synonyms.
To enable alternative forms of query:
- Conversational UI: Queries that are formed as sentences can be processed by insight engines and responses can be served in a conversational way, facilitating human understanding of insights.
What are the use cases?
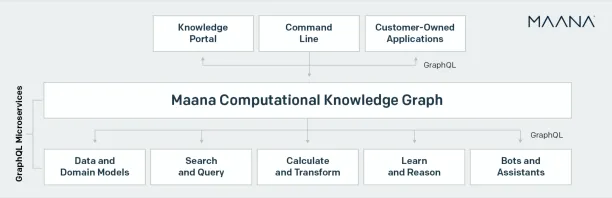
Maana, one of the vendors in the space, outlines above how their platform can serve insights to various interfaces (portal, command line, customer applications).
Internal Search
This is the most common use case of insight engines. Employees use these internal search engines to quickly access insights. Insight engines enable internal search to be more personalized and accurate thanks to their machine learning capabilities.
Internal search can also power analytics. Some vendors provide the capability of transforming unstructured data into structured form so that data scientists can easily perform analysis on data.
External Search
Insight engines can also enable companies to offer search capabilities to their users/customers. For example, content-heavy websites, e-commerce sites, and social media sites benefit from search functionality.
Insight applications
These insights can be embedded in different applications enabling smart, timely recommendations to users. Search is still the dominant form for individuals to find information. However, with the advance of machine learning algorithms and by continuously learning from user behavior, insight engines are becoming better at predicting when users will need insights and serving those insights in a timely manner.
What are the factors to consider while choosing a vendor?
Security
Insight engines give employees access to a wide range of enterprise data. Insight engines should have access authorization features (especially for vulnerable content) so that organizations can prevent data breaches.
Integration
Since insight engines’ goal is extracting information from various sources, not being able to integrate with all available data sources will decrease the effectiveness of insight engines. Therefore any insight engine must be connected to all data sources.
Scalability
Organizations seek valuable insights hidden in large volumes of data, a challenge that grows as data production increases. Modern insight engine solutions must operate quickly and at scale, offering access to all organizational insights within milliseconds. While this may seem difficult, it’s achievable—just as Google can index the entire web, similar solutions can be implemented to efficiently analyze and retrieve insights from a company’s specific data.
What are some insights engine case studies?
Challenge: With 10 million monthly posts, 3 million daily comments, and 330 million daily users, Reddit needed a search solution that can scale and quickly find relevant content for 47 million searches per day.
Solution: Reddit implemented Apache Solr (open-source search technology) by using Lucidwork’s Fusion insight engine to enhance the user-generated query capabilities of the website.
Results: Search cluster reduced from 200 to 30 nodes. Search errors were almost fully eliminated. The image below compares the number of errors between the legacy search app (yellow) and Fusion (Green). Green spikes indicate the incidents when nodes were taken down for updates.
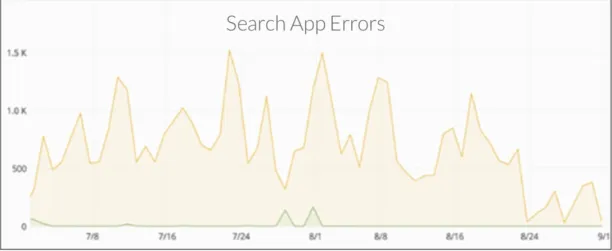
What are the best practices?
- Ensure awareness: If insights engines are not embedded in applications, employees need to proactively use them. Therefore awareness of the tool is important. And once employees get used to working with insight engines, they can easily adapt to them since they are already used to working with search engines like google that act as insight engines for the web.
- Invest in proven technology: Find an organization that had similar goals and projects so that you can see whether a particular vendor offered a successful solution for that organization. Your solution should provide enterprise-grade security, integrated machine learning with strong NLP capabilities and an easy-to-use UX.
What are the leading tools?
- Attivio Cognitive Search and Insight Platform
- Cloud Search by Google
- Cogito Intelligence Platform
- Coveo Platform
- EXALEAD
- Funnelback
- Fusion by Lucidworks
- Goldfire
- IDOL by Microfocus
- iFinder elastic
- InSpire
- InfoNgen
- Microsoft Search
- Sinequa ES
- Watson Discovery
You can compare insight engines through our data-driven list of tools.
Insight engines are the next step in enterprise search. If you want to remember what it is, why is it important, and how does it work, we recommend you to read our article.
If you still have questions about insight engines, don’t hesitate to contact us:
Comments
Your email address will not be published. All fields are required.