Cyber threats are evolving due to developments in AI in network security and generative AI. By leveraging machine learning, advanced analytics, and automation, AI enables businesses to enhance their security posture, identify vulnerabilities, reduce response times, and allocate resources more efficiently.
- AI’s market size reached $305 billion in 2024. 1
- ~1.5 billion cyberattacks on IoT devices have been reported in 2020. (AIMultiple)
- The cost of a data breach rose from ~$3.8M to ~$4.2M in 2020. (AIMultiple)
We examine how AI is transforming the cybersecurity landscape, the challenges and real-life examples, the key technologies, and practical applications.
How is AI transforming the cybersecurity landscape?
AI is revolutionizing cybersecurity by improving threat detection, response times, and overall security strategies. Using machine learning and analytics, AI can identify and mitigate threats faster and more accurately than traditional methods. By analyzing vast amounts of data, AI detects patterns and anomalies, enabling proactive defense against cyberattacks. Consider the following statistics:
- 61% of organizations security analysts reported that they cannot detect breach attempts today without AI technologies, and AI can reduce the time to detect threats by up to 90% 2
- ~75% of cybersecurity teams said they are adopting AI to mitigate cybersecurity risks. (AIMultiple)
- Companies with no security AI and automation averaged a cost of ~$7 million per breach, whereas an organization with a fully deployed system saw costs averaging ~$ 3 million. (AIMultiple)
- Global security and risk management spending is estimated to reach over $150+ billion in 2024. (AIMultiple)
Challenges of AI in Cybersecurity with Real-life Examples
1-AI Washing
AI washing refers to exaggerating or falsely claiming the use of AI to attract customers, investors, or media attention, a practice that has grown as AI and machine learning gain popularity. A key aspect is overstating capabilities, such as presenting basic algorithms (e.g., rule-based systems) or human labor as advanced AI.
For instance, many SIEM and antivirus tools use rule-based methods. Companies also use AI buzzwords like ‘machine learning’ and ‘deep learning’ to appear innovative, even when their AI implementation is minimal or nonexistent. This misleads customers and stakeholders, making it harder to distinguish genuine AI-driven solutions
Real-life examples
While this problem is rife in the cybersecurity industry with even vendors complaining about it,3 most products claiming advanced AI capabilities are closed-source products, sold to a limited audience of audience buyers with contracts that avoid technical details of how these solutions are built. As a result of these conditions, it is unlikely to prove that a cybersecurity company engaged in AI washing. Therefore, we present examples from other industries in this section.
AI Washing Allegations: Coca-Cola’s Y3000 Flavor Controversy
Coca-Cola faced allegations of AI washing for a campaign that claimed a new drink had been created using AI. The company announced that the Y3000 flavor was ‘co-created’ with AI but failed to provide any substantial details on the AI’s role in the process. Critics argued that Coca-Cola appeared to be leveraging the AI label to enhance the product’s perceived innovation.4
AI Washing in Finance: SEC Charges Firms for Misleading AI Claims
Similarly, in the finance and investment sector, two firms were charged by the SEC this year for making ‘false and misleading statements’ about the degree to which AI was used in their investment strategies.5
2-Privacy Concerns and Data Security
As AI systems increasingly rely on vast amounts of personal data, the risk of unauthorized access, data breaches, and misuse of sensitive information grows. Ensuring data security measures and privacy protections is essential to maintain user trust and compliance with regulations.
This involves implementing encryption, secure data storage solutions, and transparent data handling practices. Additionally, organizations must adopt privacy-by-design principles to protect individual’s information from the outset, mitigating potential risks and safeguarding user privacy in the digital landscape.
Increased attack surface
The increased attack surface is another crucial area in the context of privacy concerns and data security. It arises from factors often linked to modern systems’ growing complexity and interconnectedness. One primary reason is the proliferation of devices and endpoints. The widespread use of IoT (Internet of Things) devices, mobile devices, and remote workstations means that there are more potential entry points for attackers. Each device, if not properly secured, can become a gateway for unauthorized access, thereby increasing the overall vulnerability of the network.
Another significant factor contributing to the increased attack surface is the complexity of modern network infrastructures. Today’s networks often comprise a blend of on-premises, cloud-based, and hybrid environments. Managing security across such diverse and intricate networks is challenging, and this complexity often leads to gaps that can be exploited by attackers. Ensuring that every component within this multifaceted environment is secured is a daunting task, and any oversight can provide a potential vulnerability.
Real-life examples
The Cambridge Analytica scandal
The Cambridge Analytica scandal was a 2018 controversy involving the misuse of personal data from millions of Facebook users without their consent. A third-party app, “This Is Your Digital Life,” collected data from users who installed it and their friends, ultimately affecting an estimated 87 million people. Cambridge Analytica, a British political consulting firm, used this data to build psychological profiles for targeted political advertising, influencing major events like the 2016 U.S. presidential election and the Brexit referendum.
The scandal exposed Facebook’s lax data privacy policies and sparked global outrage over how personal data was being exploited for political purposes. It led to significant legal and financial repercussions, including a $5 billion fine for Facebook from the U.S. Federal Trade Commission (FTC). This incident also fueled global calls for stronger data privacy regulations, such as GDPR, and raised awareness about the ethical implications of data-driven political campaigns. 6
Samsung’s leak of sensitive data via ChatGPT
Samsung initially endorsed the use of ChatGPT among its employees, appreciating its potential to enhance productivity. However, after allowing engineers in its semiconductor division to use the AI tool, several instances of sensitive data leaks occurred. Engineers input confidential information, such as source code and internal meeting notes, into ChatGPT, leading to unintended data breaches.
In response to these breaches, Samsung issued warnings about the risks associated with using AI tools like ChatGPT, emphasizing that once data is inputted, it cannot be retrieved from OpenAI’s servers. This led Samsung to ban the use of generative AI tools temporarily and to explore developing its own in-house AI solutions to mitigate such risks. 7
3-Technical Limitations and the Need for Human Oversight
While powerful, AI systems often struggle to understand context, handle ambiguous situations, and make ethical decisions. These limitations necessitate human oversight to ensure AI applications function correctly, make appropriate decisions, and do not cause unintended harm. Human intervention is crucial for validating AI outputs, addressing unexpected issues, and guiding the ethical use of AI technologies.
In cybersecurity specifically, AI’s sole use is unsuitable for security configurations without human oversight. First, AI systems can misinterpret data or fail to recognize nuanced threats, especially those that deviate from known patterns. Second, AI can be susceptible to sophisticated attacks designed to exploit its algorithms. Third, AI lacks the contextual understanding and adaptive reasoning that human experts bring, which are crucial for responding to dynamic and complex security challenges.
Real-life examples
AI and Human Oversight in Facebook’s 2017 Content Moderation
In 2017, Facebook implemented AI systems to detect and remove content that violated its community standards, such as hate speech and violent images. However, the AI systems faced significant challenges in accurately interpreting context and nuances in the content. This led to several instances where the AI flagged harmless posts as harmful and failed to detect genuinely harmful content.
Facebook employed a large team of human moderators to review the content flagged by AI. The human moderators provided the necessary context and understanding that the AI lacked, ensuring that the content removal process was accurate and fair. For example, while AI might misinterpret a sarcastic comment as hate speech, human moderators could correctly identify the intent behind the comment and make the appropriate decision. This combination of AI and human oversight helped Facebook improve its content moderation efforts, reducing the number of false positives and ensuring that harmful content was more effectively identified and removed.8
4-Lack of Transparency
The “black box” nature of many AI systems creates challenges for cybersecurity due to their lack of transparency. This opacity limits security experts’ ability to understand decision-making processes, identify vulnerabilities, and predict behavior under different scenarios. It also complicates detecting and mitigating threats like adversarial attacks, where minor input changes lead to incorrect outputs.
The lack of transparency further impairs auditing and verifying AI systems for compliance with security standards and regulations. This results in blind spots in security protocols, leaving vulnerabilities open to exploitation. Incident response and forensic investigations are also hindered, as understanding the cause and extent of AI-related breaches often requires inaccessible knowledge of the system’s functioning..
5 Cybersecurity Challenges Addressed by AI With Real-Life Examples
1-Alert Overload
Alert overload is a significant challenge in cybersecurity and other fields where monitoring systems generate a vast number of alerts. These alerts can overwhelm operators, making it difficult to discern genuine threats from false positives. This issue strains resources and risks critical alerts being overlooked, ultimately reducing the efficiency and effectiveness of threat response.
Example: IBM Security QRadar SIEM Implementation for a Gulf-Based Bank
A notable example of AI addressing alert overload in cybersecurity is IBM Security QRadar SIEM’s deployment at a major Gulf-based bank. This institution faced significant challenges with its previous Security Information and Event Management (SIEM) system. The legacy system generated an overwhelming number of alerts, many of which were false positives, leading to alert fatigue among security analysts and delayed response times.
To overcome these challenges, the bank implemented IBM Security QRadar SIEM, leveraging its advanced AI and machine learning capabilities. The implementation of IBM Security QRadar SIEM resulted in a reduction in alert volume and false positives. 9
2-Phishing Attacks
Phishing attacks present a persistent cybersecurity challenge as they exploit human vulnerabilities to gain unauthorized access to sensitive information. Attackers use deceptive emails, messages, or websites that appear legitimate to trick individuals into revealing personal data, such as passwords, credit card numbers, or social security information. The sophistication of these attacks is increasing, with perpetrators using social engineering techniques and personalized tactics to enhance their effectiveness.
Example: Google’s Gmail Phishing Detection
Google’s Gmail service handles over 1.5 billion users’ emails worldwide. The platform faces millions of phishing attempts daily, where attackers send emails that appear legitimate but are designed to steal sensitive information from users.
Google uses machine learning models to enhance its phishing detection capabilities. These models are trained on datasets of email characteristics, including the email’s content, sender information, and metadata. 10
3-Malware, Ransomware, and advanced persistent threats (APT)
Malware can infiltrate systems to steal data or cause damage, while ransomware encrypts files, demanding payment for their release. APTs are particularly insidious, involving prolonged, targeted attacks that aim to establish a persistent presence within a network to extract valuable information. Traditional security measures often fail to detect these sophisticated attacks in real time, leading to significant breaches and data losses.
Example: Darktrace Deep Learning Threat Detection
Darktrace, a cybersecurity firm, leverages deep learning to enhance its threat detection capabilities. The company’s platform uses advanced machine learning algorithms to identify and respond to threats autonomously in real time. 11
4-Financial Data Security
Financial data security is a paramount cybersecurity challenge as it involves protecting sensitive information such as bank accounts, credit card numbers, and transaction records from unauthorized access and fraud. The financial sector is a prime target for cybercriminals due to the high value of the data and the potential for significant financial gain. Organizations must employ robust encryption, access controls, and intrusion detection systems to safeguard this data.
Example: Capital One Enhances Cybersecurity with AI-Driven Threat Detection and Response
Capital One, a major financial institution, needed to enhance its cybersecurity defenses to protect sensitive financial data from increasingly sophisticated cyber attacks. Traditional network security and measures were not sufficient to manage the extensive amount of data and detect threats in real-time.
Capital One integrated AI-driven security tools into its cybersecurity framework. They deployed AWS Macie, an AI-powered data security service, to automatically discover, classify, and protect sensitive data. Macie continuously monitors data activity, identifying anomalies and potential threats.
Additionally, Capital One utilized automated threat detection and response tools leveraging machine learning to detect unusual patterns and behaviors across their network. Upon identifying a threat, the system automatically isolates affected systems, blocks suspicious activities, and notifies the security team. This automation reduced incident response times, minimized damages, and allowed the security team to focus on complex threats.12
5-Content Moderation in User-Generated Social Media Platforms
While it could be argued that content moderation is not directly related to cybersecurity, it involves aspects of cybersecurity practices, from detecting and mitigating threats to ensuring real-time responses and protecting user rights. These platforms must identify and mitigate harmful content, including misinformation, hate speech, and illegal activities while balancing user privacy and freedom of expression.
Malicious actors often use sophisticated techniques to evade detection. The cybersecurity challenge here is to stay ahead of these evasion techniques to prevent harmful content from spreading.
Example: Facebook Social Media Monitoring for Threat Detection
Facebook uses Natural Language Processing (NLP) to scan and analyze posts, comments, and messages for harmful content. NLP algorithms detect keywords and phrases associated with threats and extremist content, while sentiment analysis gauges the emotional tone, flagging posts with extreme negativity or aggression.
Advanced models identify and then analyze data and the context around specific entities to understand broader implications. Topic modeling groups similar posts to identify trends or coordinated campaigns. The system recognizes deviations in language and behavior patterns, signaling potential threats. Continuous updates and machine learning on large datasets of harmful content enhance detection accuracy and reduce false positives. 13
Key AI Technologies in Cybersecurity
Machine Learning (ML) and Deep Learning
Machine Learning (ML) is a subset of AI that enables systems to learn and improve from experience without being explicitly programmed. In cybersecurity, ML is used in:
- Anomaly Detection: ML algorithms can identify unusual patterns in network traffic, user behavior, and system activities that may indicate a security threat.
- Spam and Phishing Detection: By analyzing large datasets of email attributes and content, ML models can classify and filter out spam and phishing attempts with high accuracy.
- Malware Detection: ML can help identify malicious software by analyzing file characteristics and behaviors and distinguishing between legitimate and malicious files.
- Predictive Analysis: ML can predict potential security breaches by analyzing historical data and identifying trends and vulnerabilities.
- Explainable AI (XAI): XAI provides transparency in ML models by making their decisions understandable to humans, enhancing model accuracy, accountability, and compliance.
Deep Learning is a more advanced subset of ML that uses neural networks with many layers (deep neural networks) to model complex patterns in large datasets. In cybersecurity, deep learning is used for:
- Intrusion Detection Systems (IDS): Deep learning models can analyze vast amounts of network traffic data to detect sophisticated intrusions that traditional methods might miss.
- Advanced Threat Detection: Deep learning can identify complex and evolving threats, such as zero-day exploits, by learning from vast amounts of cybersecurity data.
- Behavioral Analysis: Deep learning models can analyze user and entity behaviors to detect deviations that may indicate insider threats or compromised accounts.
Natural Language Processing (NLP) for Threat Detection
Natural Language Processing (NLP) is a branch of AI that deals with the interaction between computers and human languages. In cybersecurity, NLP is employed in:
- Threat Intelligence: NLP can process and analyze large volumes of text from various sources, such as security reports, forums, and dark web data, to extract valuable threat intelligence.
- Phishing Detection: By analyzing the content of emails, messages, and websites, NLP can identify linguistic patterns and cues that indicate phishing attempts.
- Automated Incident Response: NLP can help automate the analysis of security logs, incident reports, and alert messages, making it easier for security teams to prioritize and respond to threats.
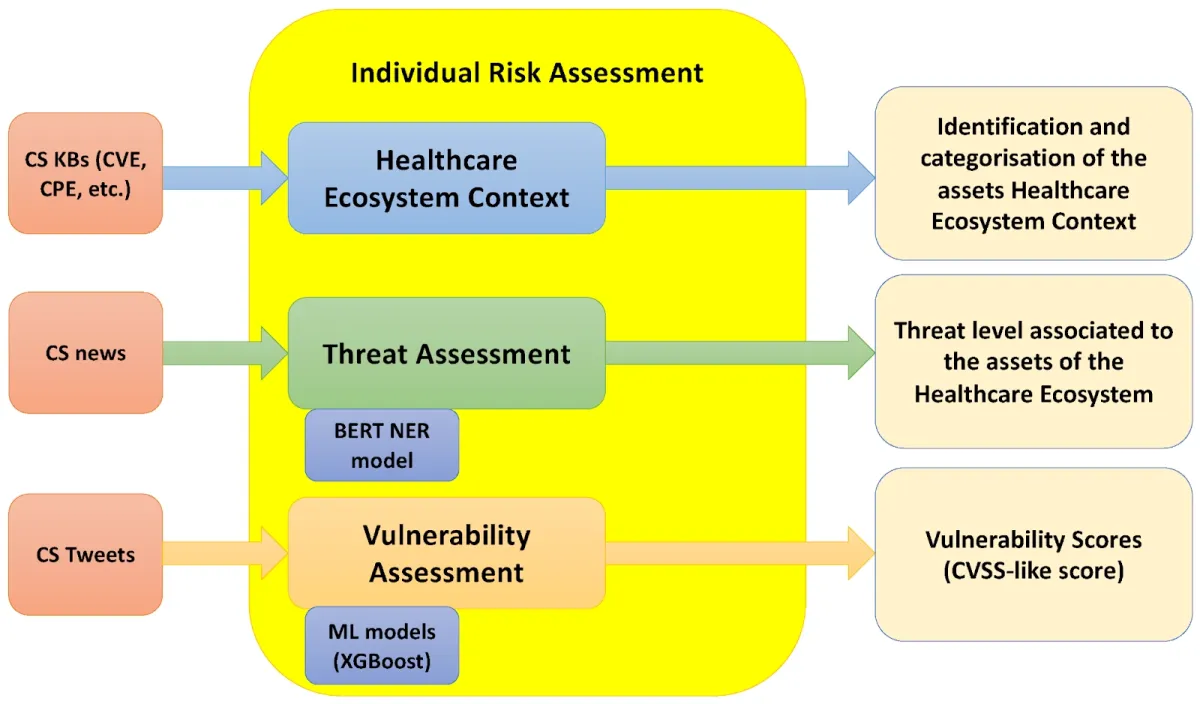
Figure 1. A Machine Learning Approach for the NLP-Based Analysis of Cyber Threats, Vulnerabilities of the Healthcare Ecosystem Example. 14
Automation via AI
Automation in cybersecurity involves the use of AI and other technologies to perform tasks that would otherwise require human intervention. Its impacts include:
- Faster Incident Response: Automation can significantly reduce the time taken to detect, analyze, and respond to security incidents, minimizing potential damage and helping cybersecurity professionals.
- Scalability: Automated systems can handle large volumes of security data and activities, making it easier to manage cybersecurity at scale.
- Consistency and Accuracy: Automation reduces the risk of human error and ensures that security protocols and responses are consistently applied.
- Resource Optimization: By automating routine and repetitive tasks, security teams can focus on more strategic and complex issues, improving overall efficiency.
Examples of Traditional Cybersecurity Measures
- Antivirus Software:
- McAfee or Norton Antivirus: Detects malware based on predefined signature databases.
- Firewalls:
- Cisco ASA or Fortinet FortiGate: Implements rule-based filtering of network traffic.
- Intrusion Detection Systems (IDS):
- Snort: Monitors network traffic for known attack patterns using predefined rules.
- Manual Threat Hunting:
- Security analysts manually inspect logs and alerts for signs of compromise.
- Password Policies:
- Enforcement of strong password rules and periodic changes to protect against unauthorized access.
Protecting AI-powered applications
Protecting AI-powered applications is crucial because these systems often handle sensitive data, make critical decisions, and are integral to various industries, including finance, healthcare, and security. A breach or malfunction can lead to significant financial losses, privacy violations, and even threats to human safety. Ensuring robust protection mechanisms is essential to maintain trust, compliance, and operational integrity. The table below highlights key aspects and examples of measures necessary for safeguarding AI-powered applications.
Aspect | Description | Examples |
---|---|---|
Data Integrity | Ensuring the accuracy and consistency of data used by AI systems to prevent malicious alterations. | Implementing robust data validation processes and using blockchain for immutable data records. |
Model Security | Protecting AI models from adversarial attacks and theft. | Using techniques like adversarial training and differential privacy to secure models. |
Access Control | Restricting access to AI systems and sensitive data to authorized personnel only. | Implementing role-based access control (RBAC) and multi-factor authentication (MFA). |
Monitoring & Auditing | Continuously monitoring AI system activities and auditing logs to detect and respond to suspicious behavior. | Using anomaly detection systems and regular security audits to identify potential threats. |
Data Privacy | Protecting the privacy of data used and generated by AI applications. | Implementing data anonymization and encryption techniques to secure user data. |
Robustness Testing | Ensuring AI systems can handle unexpected inputs and attacks gracefully. | Conducting stress testing and deploying fallback mechanisms to maintain system stability. |
Compliance | Adhering to legal and regulatory requirements related to AI and data protection. | Ensuring compliance with GDPR, CCPA, and industry-specific regulations like HIPAA for healthcare. |
Incident Response | Preparing and executing a plan to respond to security breaches involving AI systems. | Developing a comprehensive incident response plan and conducting regular drills. |
Explainability | Making AI decision-making processes transparent and understandable. | Implementing explainable AI (XAI) techniques to provide insights into AI model decisions. |
Supply Chain Security | Securing the supply chain of AI components and services to prevent tampering and ensure reliability. | Vetting third-party vendors and implementing security standards for AI hardware and software. |
Further Reading:
If you have further questions, reach us
External Links
- 1. Global AI market size 2030| Statista. Statista
- 2. Research library - Capgemini.
- 3. Was RSA Conference AI-washed or is AI in cybersecurity real? | Sumo Logic.
- 4. Spotting AI Washing: How Companies Overhype Artificial Intelligence.
- 5. Spotting AI Washing: How Companies Overhype Artificial Intelligence.
- 6. nytimes.com. The New York Times
- 7. Samsung Bans ChatGPT Among Employees After Sensitive Code Leak. Forbes
- 8. Update on Our Progress on AI and Hate Speech Detection | Meta.
- 9. IBM Security QRadar SIEM Design and Implementation for a Gulf-Based Bank - Case Study.
- 10. spam detection: Google develops AI-powered spam detection to make Gmail safer - The Economic Times.
- 11. The AI Arsenal: Understanding the Tools Shaping Cybersecurity | Resources | Darktrace.
- 12. AWS Technology Enables Capital One's Move to Machine Learning | Video | AWS.
- 13. Natürliche Sprachverarbeitung - Messenger-Plattform - Dokumentation - Meta for Developers.
- 14. A Machine Learning Approach for the NLP-Based Analysis of Cyber Threats and Vulnerabilities of the Healthcare Ecosystem.
Comments
Your email address will not be published. All fields are required.