Generating insights from unstructured data has long been a strategic aim among organizations that
- Have old datasets (e.g. government records).
- Collaborate with multiple stakeholders (e.g. insurers, healthcare providers).
Lazarus AI builds foundation models (e.g. RikAI) to solve complex and urgent problems involving large amounts of unstructured private data.
Lazarus is active in these industries: Government (e.g. defense), insurance (e.g. damage assessment of catastrophic events), healthcare, and banking.
See Lazarus AI’s key extractive AI capabilities and use cases:
Lazarus AI
Unlike most other companies focused on foundation models, Lazarus has flown under the radar until recently because they have initially focused on government work. They have been serving the US government since 2022.
The company uses optical character recognition (OCR) technology and a proprietary language model to contextualize content, assess structures, and capture natural language in documents with minimal human intervention.
The solution can identify both “handwritten” & “typed” language, and classify the outputs as factual/highly probable, possible, and speculative along with relevant citations to facilitate human validation.
Use cases
Lazarus AI provides custom solutions for global insurers, healthcare organizations, business process outsourcers (BPOs), and governments in streamlining data entry and transfer processes for a variety of documents, including:
- claims forms
- new patient intake
- adjuster reports
- lab results
- physician statements
- medical records
- receipts
Users can ask questions to retrieve information from a document such as:
- What is the member ID?
- What medication is being taken by the patient?
- What is this document about?
Video 1: Extracting information on Larurus with RikAI LLM
Source: Loom1
KPIs
Lazarus AI claims that they can achieve the following metrics with the base model without any client-specific fine-tuning or training:
- Document related metrics:
- OCR accuracy: 92% in 140 languages.
- Typed text accuracy: 99.5%
- Handwriting accuracy: 92% on physician handwriting
- Problem-solving capacity: Capable of conducting >1000 steps in solving highly complex problems.
- Hallucination rates:
- Document understanding: 2bn+ context window with less than 1% hallucination rate in 140 languages.
- Multi-modal understanding: 1bn+ context window with less than 5% hallucination rate.
How does Lazarus AI extract data?
1. Capturing documents with OCR
Lazarus AI uses optical character recognition (OCR) technology & text recognition to convert captured documents (e.g. scanned paper documents, PDFs, or images) into editable and searchable data. Lazarus AI can capture data from:
- Emails, reports, and social media posts
- Invoices, receipts, forms, bank statements, letters, and contracts
- Handwritten documents
2. Pre-processing
Pre-processing improves the quality of the retrieved data and prepares it for further data processes. This involves activities such as:
- Text normalization
- Sensitive data identification
- Font irregularities & ink inconsistencies detection
3. Classification & summarization
Lazarus AI classifies and summarizes documents based on their content. For example, it can identify & categorize medical records based on patient numbers or medicine types.
Some examples include:
- Large document summarization (e.g. public and private bond offerings)
- Unified image and text contextual classification for underwriting inspection reports.
- Transaction enrichment for examining payment and transaction data to observe an account holder’s financial behavior fully. This includes insights about preferred merchants, shopping habits, and financial products.
- Examination of syndicated loans, including risk factors.
- Semantic classification of contract terms (International Swaps and Derivatives Association (ISDA) Applicability).
4. Data extraction and structured storage
Based on the document classification and summarization, Lazarus AI leverages a data extraction API to retrieve & store the captured data in a structured format. For a receipt, the classified data includes the transaction date, the business’s name, a description of the goods or services provided, and the amount paid.
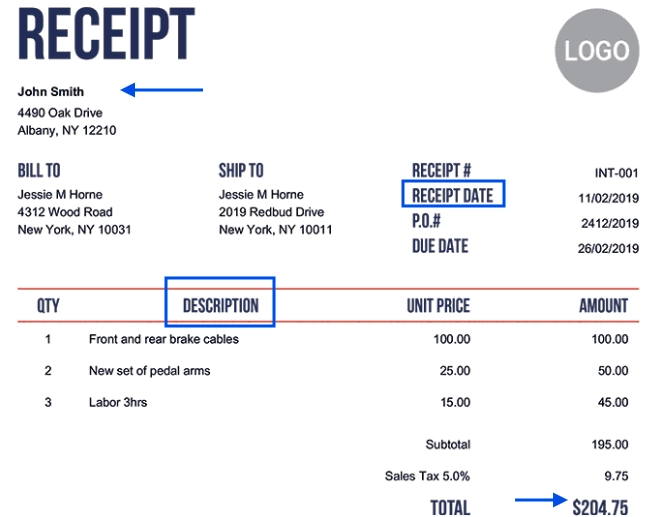
To accomplish this, the solution determines the type of information in the document by using a large language model, called RikAI, and rule-based functions, then stores it as metadata. This helps eliminate the need for human data entry or transfer to predefined query forms.
5. Data validation
Before sending information to a workflow, the data must be reviewed to ensure that the context is correct. Lazarus AI performs a validation step, in which the system compares the extracted information to the relevant documents. For example, Lazarus AI can compare the receipt items to the invoice items to validate data.
Demo & illustrations
Classification example: The user can ask, Lazarus AI: Is this document an “insurance policy”, “reinsurance agreement”, “cooking recipe” or an “instruction manual”? Explain why.
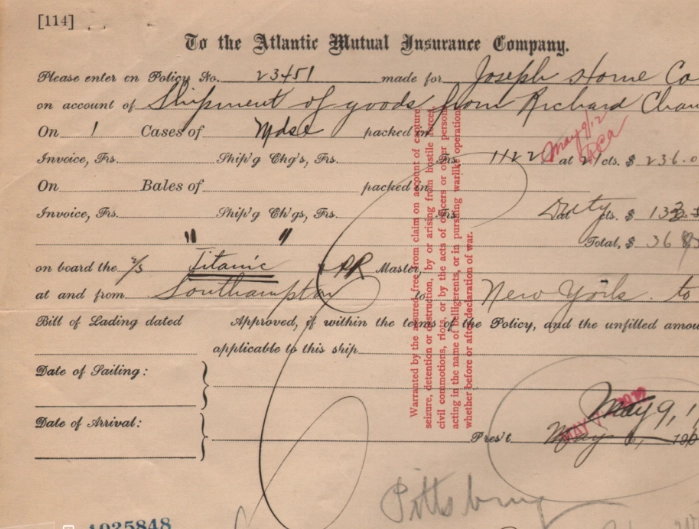
Lazurus AI can reply to the input by classifying the document. In this example, Lazarus would provide an output classifying the above document by stating that, this is an insurance policy document since:
- The direct address includes “Atlantic Mutual Insurance Company” and the mention of a policy number.
- The statement includes the shipment of goods from “Richard Chandler & Co.” to “Joseph Store Co.”, which is a typical subject for an insurance policy.
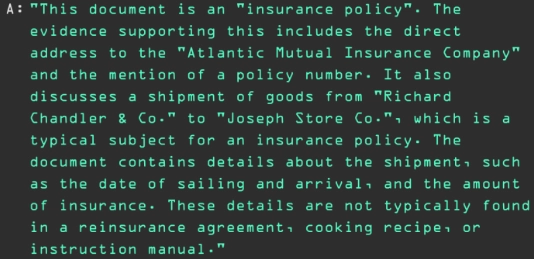
High precision extraction example: Users can upload a handwritten car crash report as a PDF file with advanced document understanding API, and ask Lazarus AI to extract driver demographics as JSON.
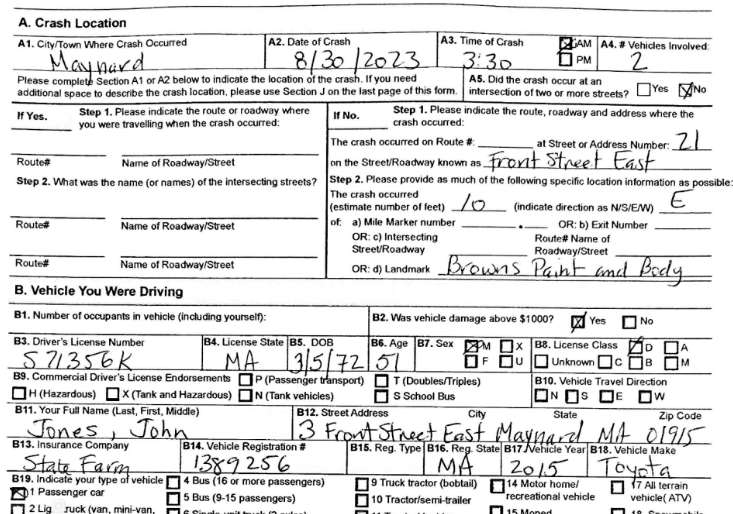
Lazarus AI captures and translates visuals into structured data, providing detailed file information including the driver’s full name, address, city, state, etc.
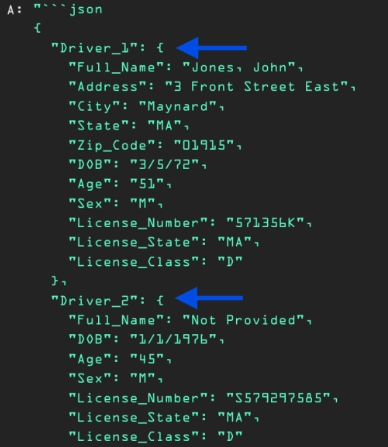
High precision extraction & summarization: Users can use Lazarus AI for extracting & summarizing any unstructured notes (e.g. clinical notes). For example, users can ask the solution to create a breakdown of the patient’s history including vitals, blood pressure, and medications.
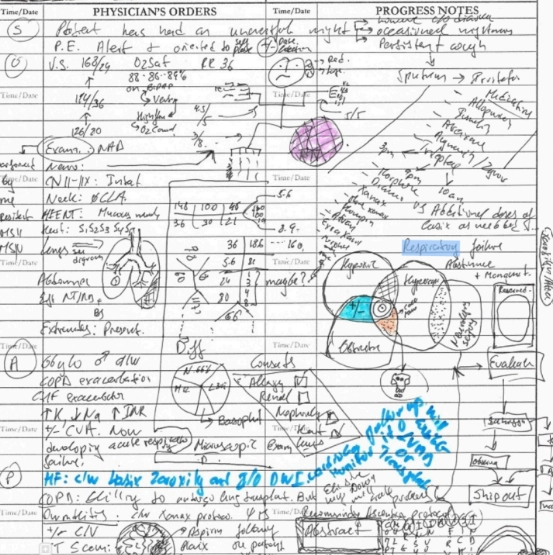
Lazarus AI will provide the patient’s history based on the clinical notes. The output will include the patient’s vital signs and medications.
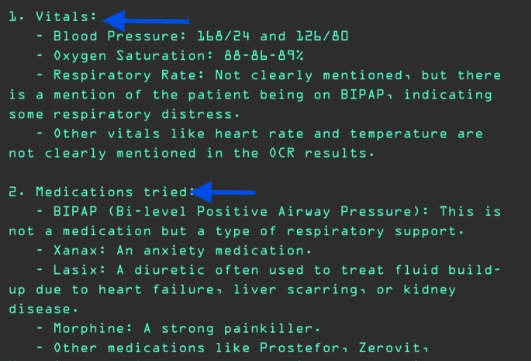
Reasoning temporal dimensionality: Consider a compliance analyst reviewing transaction-related documentation. The compliance analyst could ask Lazarus AI to identify potential red flags and inconsistencies in a service argument. The analyst can also classify the answers as factual/highly probable, possible, and speculative.
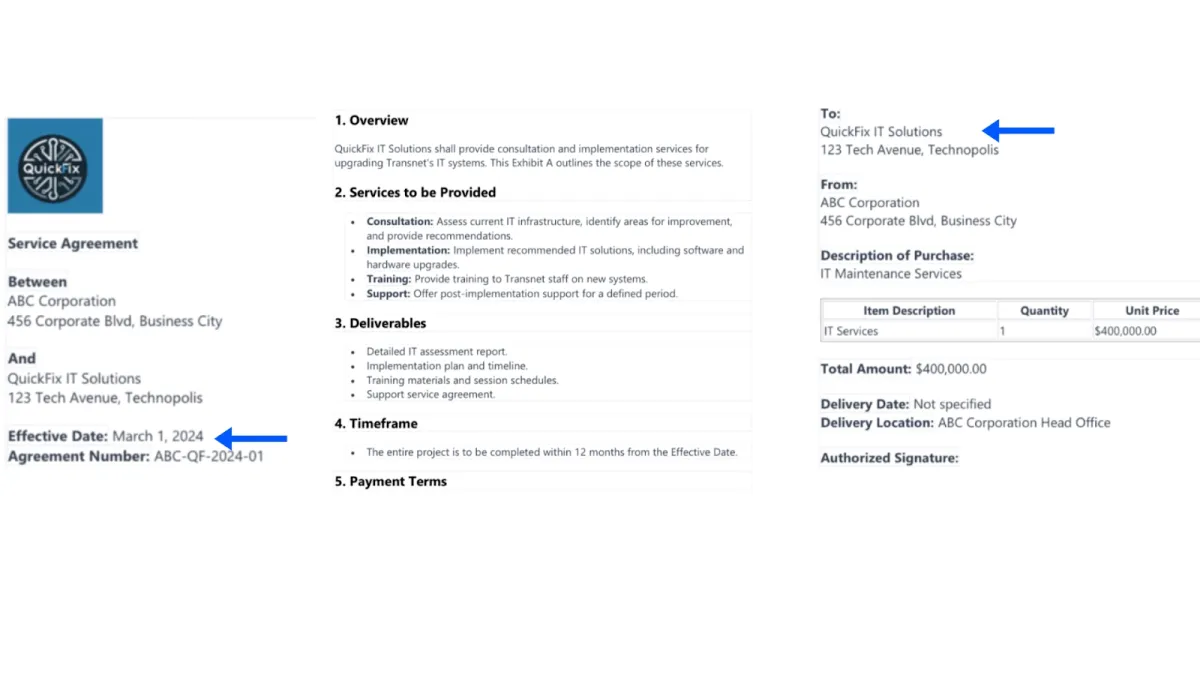
Upon reviewing the provided documentation, Lazarus AI would provide the potential red flags and inconsistencies.
Factual/highly probable findings:
- The invoice from QuickFix IT Solutions is dated March 3, 2023, which is before the effective date of the service agreement (March 1, 2024).
- The service agreement (exhibit B: Payment Terms) states that the entire payment amount will be due upon completion of the services and acceptance by Transnet. However, the invoice states that the payment is due upon receipt.
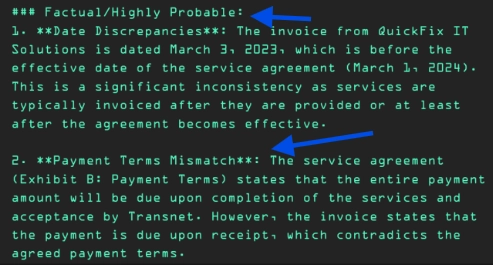
Possible findings:
- Third-party involvement
- Early invoice
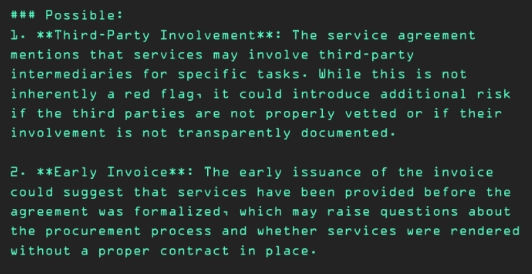
Image comprehension – Example 1: Consider a case in which a user needs to analyze layouts in satellite images, before and after the hurricane. The user can ask Lazarus AI to describe step by step the analysis of signs and damage done by the flooding and strong winds expected with the hurricane. The users can also classify the findings into the following classes: factual/highly probable, possible, and speculative.
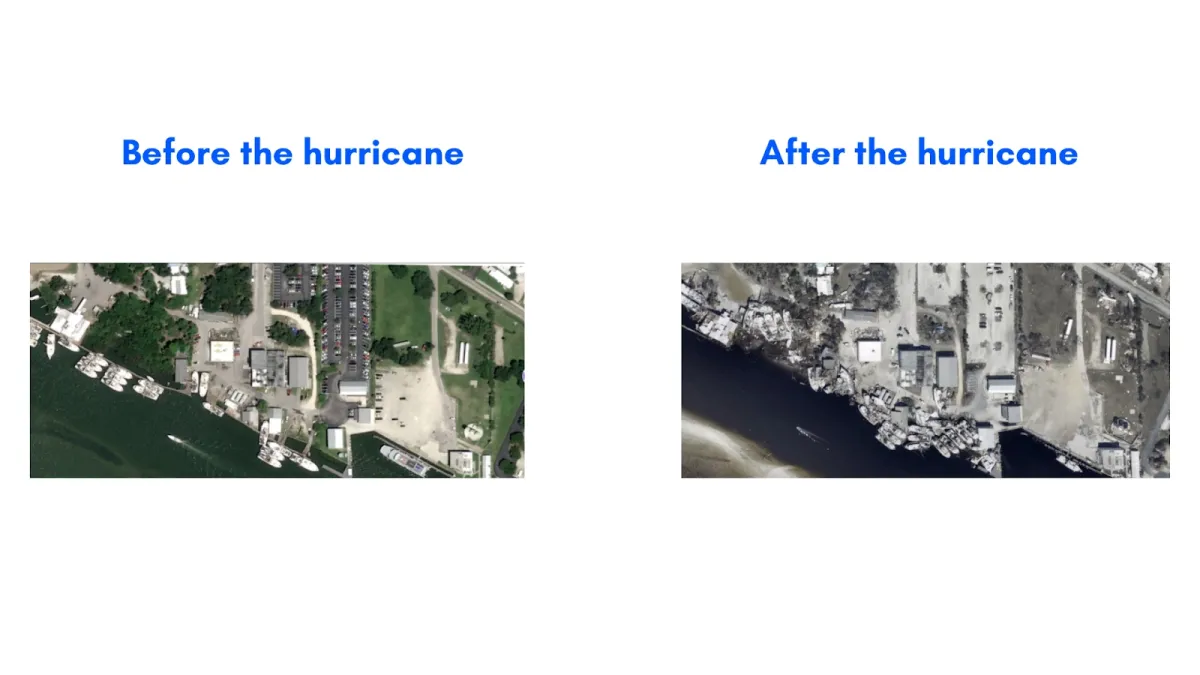
Lazurus AI will compare the before and after images and classify the findings.
Factual/highly probable findings:
- Structural damage to buildings
- Boat displacement
- Vegetation damage
- Changes in the ground surface
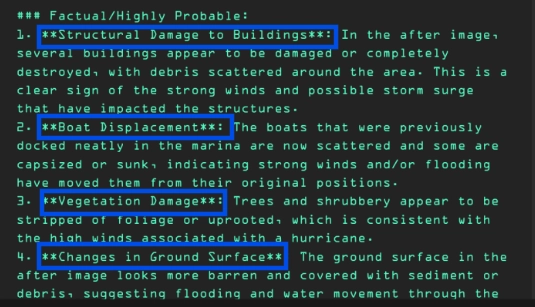
Possible findings:
- Road damage
- Infrastructure damage
- Erosion
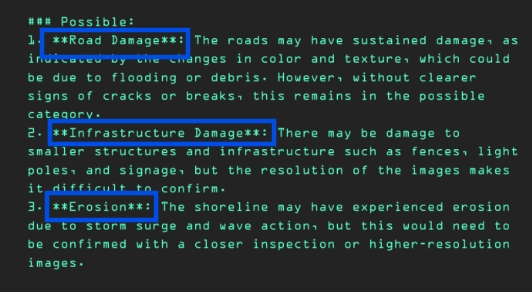
Image comprehension – Example 2: Users can analyze checks for detecting aspects of potential fraud.
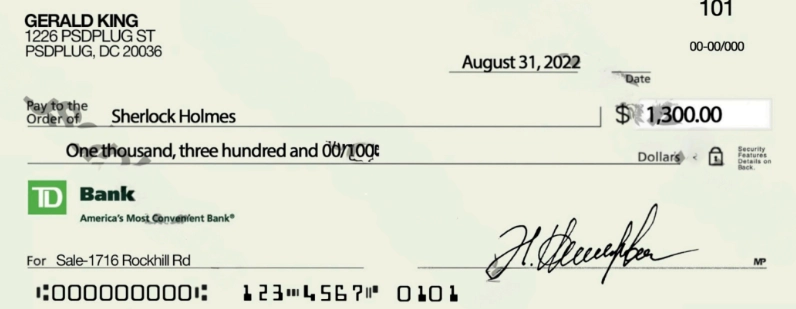
Upon examining the provided image of the check, Lazarus AI can make several observations regarding potential fraud indicators:
- Mismatched numerical and written amounts
- Inconsistency in font types
- Signature appearance
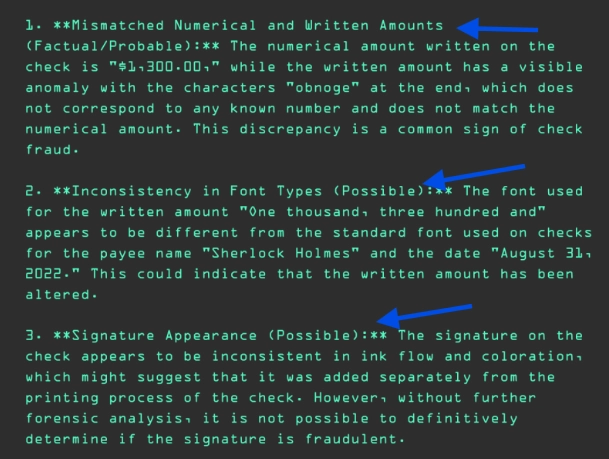
Why is Lazarus relevant?
Governments, regulated organizations, and industrial businesses have millions of unstructured files (e.g. PDF reports, PPTX presentations) and previously they could not unlock insights from these files at scale. With extractive AI, these organizations have a chance to better operationalize their unstructured data.
Generative AI vs. extractive AI
Though both technologies are built with machine learning techniques, generative AI technology is more suited for creative tasks and extractive AI is better for information retrieval and analysis. Here’s a comparison of their key aspects:
Aspect | Generative AI | Extractive AI |
---|---|---|
Focus | Creates new content | Retrieves and summarizes existing content |
Accuracy | Can be subjective, prone to hallucinations and biases | High, relies on factual data |
Transparency | Difficult to understand reasoning | Clear source of information |
Applications | Content creation, marketing, creative tasks | Search, summarization, information retrieval, knowledge management |
Extractive AI models:
- Concentrate on data extraction summarization classification of significant information from existing data sources.
- Train to extract important phrases, sentences, or sections that provide the basic semantic meaning.
- More manageable since they are confined to existing knowledge bases and written documents. This guarantees that the outputs remain on-topic.
- Promotes auditability and can explain which data they summarized and why. This is crucial in regulated sectors.
Generative AI models:
- Aim to create entirely new material. Models such as GPT-3 are trained on large datasets to generate human-like writing.
- Have an unpredictable nature raises issues about transparency. It’s difficult to audit how or why the model processes data.
- Open to errors, biases, and hallucinations. Outputs are unpredictable.
- For example, ask ChatGPT the following: “Create one short sentence about insurance underwriting.”
ChatGPT will produce two different responses at a certain time, ChatGPT (a generative language model) produced the following response:
The first response and second response have resulted in different results. Generative AI is not built for consistency.
- For example, ask ChatGPT the following: “Create one short sentence about insurance underwriting.”
Comments
Your email address will not be published. All fields are required.