Data curation is an important part of data management. Data curation is the process of collecting, wrangling and preserving data. It allows companies to store sustainable and accessible data to share and apply self-service analytics. Data-driven insights are crucial as data-driven sales strategies enable companies improve their sales productivity by 20 %. 1
However, companies analyze only 12 % of their data on average. Therefore, data scientists are encouraged to curate more datasets and metadata by familiarizing with data curation approaches.
What is data curation?
Data curation activities (collection, wrangling, preservation) create curated datasets. The main objective is to generate FAIR (Findable, Accessible, Interoperable, and Reusable) and analyzable data. The goal is to maximize data’s business value—an objective increasingly evaluated through the lens of infonomics.
What is an example of data curation?
A hospital collecting thousands of patient X-rays, removing duplicates, standardizing formats, and labeling images based on diagnosis is an example of data curation. This process prepares the dataset for use in research or training machine learning models.
Which are the three main stages of data curation?
The main stages are:
- Collection: Gathering raw data from various sources.
- Organization: Structuring, cleaning, and formatting the data.
- Enrichment: Adding metadata, labels, or context to improve usability
What does data curation include?
Data curation activities include:
- Contextualizing: In contextualizing, metadata (e.g. relevant sources and attributions) is added to the dataset. The purpose is to show the context regarding how and why the data was generated.
- Citing the data: Third-party users use appropriate attributions included in the data while citing the data.
- De-identification: Personally identifiable or protected information is removed or masked.
- Validating and adding metadata: Information about a dataset is structured in a machine-readable form for search and retrieval purposes.
- Validation of data: An expert with similar credentials and subject familiarity as the data creator reviews the dataset. It is performed to confirm the accuracy of the data.
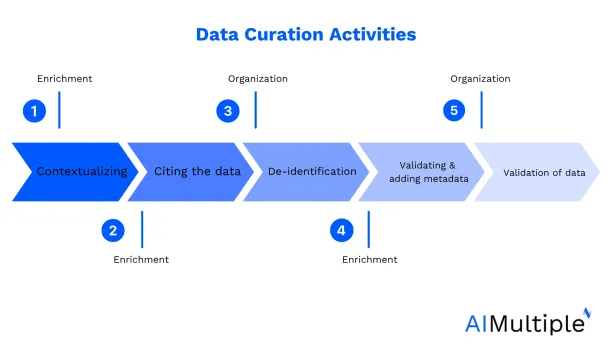
What is the difference between data curation and data governance?
Among data curation tasks, data governance plays a central role by defining how data should be controlled, accessed, and maintained within a business context.
On the other hand, data curation is a repetitive process to optimize data and metadata to ensure valuable use of data. Thus, data curation complements a successful data governance strategy.
What are the modes of data curation?
There are three main modes of data curation:
1. Manual Curation
In this mode, human experts handle the data curation process directly. They review, clean, structure, and label the data manually.
For example, in medical research, radiologists may manually label MRI scans with diagnoses to ensure high accuracy for AI training.
- Pros: High quality and context-aware.
- Cons: Time-consuming and not scalable for large datasets.
- Best for: Ideal when the data is complex, sensitive, or requires domain expertise that automation can’t yet replicate.
2. Automated (AI) Curation
Here, AI tools or algorithms are used to curate data with minimal human involvement. Tasks like deduplication, format standardization, or keyword tagging are handled automatically.
For instance, an e-commerce platform using scripts to clean customer data, remove duplicates, and categorize product descriptions.
- Pros: Fast and scalable.
- Cons: May miss nuances or introduce errors without human review.
- Best for: Useful for handling very large datasets efficiently, especially in business intelligence or web analytics.
3. Semi-Automated Curation
This combines automated tools with human oversight. Machines do the initial heavy lifting, and humans review and refine the output.
In fact, a machine learning pipeline where an algorithm suggests labels for images, and human curators confirm or correct them.
- Pros: Balance of efficiency and quality.
- Cons: Still requires human effort and monitoring.
- Best for: Common in AI/ML training datasets, where both speed and accuracy are important.
Why is data curation important?
Incorrect information, knowledge gaps, wrong guidelines are some examples of inaccurate datasets. These datasets can be:
- Biased: Some AI used for image recognition illustrated gender and racial bias.
- Inaccurate, unreliable, or incorrectly represented
- Error-ridden or ambiguous.
The absence of processed or curated raw datasets reduces feature quality and restricts the development and applications of the data. Therefore, businesses can leverage data curation for:
Improved data quality
Data curation organizes, describes, cleans, and preserves data so business analysts work with proper datasets in the long-term. It would not be easy to access, process, and make sense of data without data curation. This would prevent the creation of data swamps.
Data swamp refers to the situations when storing and accessing data is not managed correctly and gets lost in unusable data. Data curation enables the segregation of data and keeping good data in the lake. Thus, it can be applied to restore data swamps.
Explore data quality assurance with best practices
Machine Learning (ML)
Machine learning (ML) and artificial intelligence (AI) training data are prepared for processing via data curation. The training data becomes adequately labeled and categorized with data curation techniques, making it reliable, unbiased, and machine-readable.
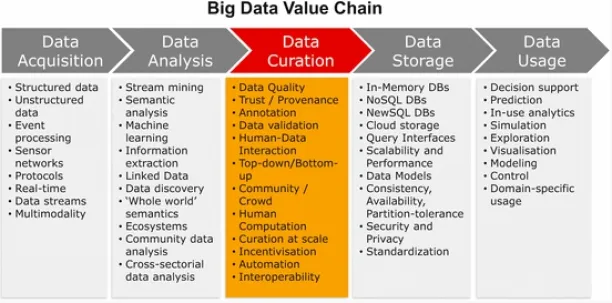
What are the challenges that face data curation?
Data curation can be a costly and challenging process while curating extensive volumes of disorganized data. In such a situation, the data creator considers various data curation approaches and handles high amounts of different data sets.
Furthermore, data has not been accumulated according to its intended usage for decades. Organizations did not know how to implement the data into their strategic decision-making. Companies are expected to leverage their expertise and knowledge concerning the types of data, its value, and why and how to use it before data curation is applied.
What are some of the data curation tools?
Data curation tools optimize the pre-processing steps of data management. These tools assure data integrity and usability. Using AI and ML, these platforms validate metadata and design insights into the accurate repository.
To explore some data-related tools, feel free to scroll down our data-driven lists of data solutions.
What is a data curator’s job?
A data curator manages the quality and usability of datasets. Their tasks include cleaning inconsistent data, organizing it for easy access, labeling it for relevance, and ensuring it meets standards for analysis, sharing, or machine learning use.
And if you believe your business will benefit from a data curation tool, let us guide you to choose one:
External Links
- 1. How data analytics helps sales reps win more deals | McKinsey. McKinsey & Company
Comments
Your email address will not be published. All fields are required.