If you are flexible about the GPU model, identify the most cost-effective cloud GPU based on our benchmark of 10 GPU models in image and text generation & finetuning scenarios.
If you prefer a specific model (e.g. A100), identify the lowest-cost GPU cloud provider offering it.
If undecided between on-prem and the cloud, explore whether to buy or rent GPUs on the cloud.
Or learn our cloud GPU benchmark methodology to identify the most cost-efficient GPU
Cloud GPU price per throughput
Two common pricing models for GPUs are “on-demand” and “spot” instances. See the most cost effective GPU for your workload based on on-demand prices from the top 3 hyperscalers:
Cloud GPU Throughput & Prices
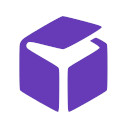
Runpod
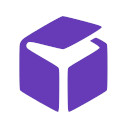
Runpod
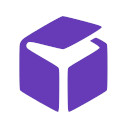
Runpod
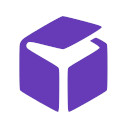
Runpod
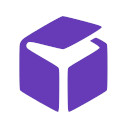
Runpod
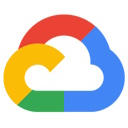
Google Cloud Platform
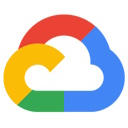
Google Cloud Platform
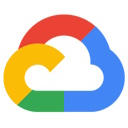
Google Cloud Platform
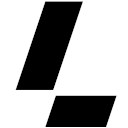
Latitude.sh
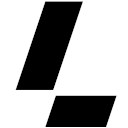
Latitude.sh
See cloud GPU benchmark methodology for details.
On-demand is the most straightforward pricing model where you pay for the compute capacity by the hour or second, depending on what you use with no long-term commitments or upfront payments. These instances are recommended for users who prefer the flexibility of a cloud GPU platform without any up-front payment or long-term commitment. On-demand instances are usually more expensive than spot instances, but they provide guaranteed uninterrupted capacity.
On-demand GPUs from other cloud providers
Cloud | GPU / Memory* | # of GPUs | On-demand $ | Throughput** | Throughput/$*** |
---|---|---|---|---|---|
RunPod | A100 / 80 GB | 8 | 15.12 | 1,362 | 90 |
TensorDock | A100 / 80 GB | 4 | 1.200 | 821 | 684 |
Vast.ai | V100 / 16 GB | 8 | 0.944 | 289 | 306 |
Vast.ai | V100 / 16 GB | 2 | 0.358 | 77 | 215 |
TensorDock | A100 / 80 GB | 1 | 1.200 | 232 | 193 |
TensorDock | V100 / 16 GB | 1 | 0.220 | 42 | 191 |
TensorDock | A100 / 80 GB | 1 | 1.400 | 232 | 165 |
Jarvislabs | A100 / 40 GB | 1 | 1.1 | 179 | 163 |
Lambda | A100 / 40 GB | 1 | 1.1 | 179 | 163 |
Lambda | H100 / 80 GB | 1 | 1.99 | 322 | 162 |
Crusoe Cloud | A100 / 80 GB | 1 | 1.650 | 232 | 140 |
FluidStack | A100 / 40 GB | 1 | 1.290 | 179 | 139 |
FluidStack | A100 / 40 GB | 1 | 1.400 | 179 | 128 |
Vast.ai | A100 / 40 GB | 1 | 1.400 | 179 | 128 |
Datacrunch | A100 / 80 GB | 1 | 1.85 | 232 | 125 |
Crusoe Cloud | A100 / 80 GB | 4 | 6.600 | 821 | 124 |
Crusoe Cloud | A100 / 40 GB | 1 | 1.450 | 179 | 123 |
Crusoe Cloud | A100 / 80 GB | 2 | 3.300 | 406 | 123 |
Seeweb | RTX A6000 / 48 GB | 2 | 1.480 | 179 | 121 |
Vast.ai | A100 / 80 GB | 1 | 2.000 | 232 | 116 |
Lambda | A100 / 80 GB | 8 | 12 | 1,362 | 114 |
Datacrunch | A100 / 80 GB | 4 | 7.4 | 821 | 111 |
Datacrunch | A100 / 80 GB | 2 | 3.7 | 406 | 110 |
CoreWeave | A100 / 80 GB | 1 | 2.210 | 232 | 105 |
CoreWeave | A100 / 80 GB | 1 | 2.210 | 232 | 105 |
FluidStack | A100 / 80 GB | 1 | 2.210 | 232 | 105 |
FluidStack | A100 / 80 GB | 1 | 2.210 | 232 | 105 |
Seeweb | A100 / 80 GB | 1 | 2.220 | 232 | 104 |
Crusoe Cloud | A100 / 80 GB | 8 | 13.200 | 1,362 | 103 |
Vast.ai | A100 / 80 GB | 4 | 8.000 | 821 | 103 |
Vast.ai | A100 / 80 GB | 2 | 4.000 | 406 | 101 |
Datacrunch | A100 / 80 GB | 8 | 14.8 | 1,362 | 92 |
Seeweb | A100 / 80 GB | 4 | 8.880 | 821 | 92 |
Oblivus Cloud | A100 / 80 GB | 1 | 2.55 | 232 | 91 |
Seeweb | A100 / 80 GB | 2 | 4.440 | 406 | 91 |
Vultr | A100 / 80 GB | 1 | 2.604 | 232 | 89 |
CoreWeave | A100 / 40 GB | 1 | 2.060 | 179 | 87 |
CoreWeave | A100 / 40 GB | 1 | 2.060 | 179 | 87 |
Crusoe Cloud | A100 / 80 GB | 8 | 15.600 | 1,362 | 87 |
Oblivus Cloud | A100 / 80 GB | 2 | 5.1 | 406 | 80 |
Oblivus Cloud | A100 / 80 GB | 4 | 10.2 | 821 | 80 |
Vultr | A100 / 80 GB | 4 | 10.417 | 821 | 79 |
Vultr | A100 / 80 GB | 2 | 5.208 | 406 | 78 |
Latitude.sh | H100 (80 GB) | 8 | 35.2 | 2,693 | 77 |
CoreWeave | H100 / 80 GB | 1 | 4.250 | 322 | 76 |
FluidStack | H100 / 80 GB | 1 | 4.250 | 322 | 76 |
Latitude.sh | H100 (80 GB) | 4 | 17.6 | 1,321 | 75 |
Oblivus Cloud | A100 / 40 GB | 1 | 2.39 | 179 | 75 |
ACE Cloud | A100 / 80 GB | 1 | 3.110 | 232 | 74 |
Latitude.sh | H100 (80 GB) | 1 | 4.4 | 322 | 73 |
Paperspace by DigitalOcean | A100 / 80 GB | 1 | 3.18 | 232 | 73 |
FluidStack | H100 / 80 GB | 1 | 4.760 | 322 | 68 |
CoreWeave | H100 / 80 GB | 1 | 4.780 | 322 | 67 |
Oblivus Cloud | A100 / 80 GB | 8 | 20.4 | 1,362 | 67 |
Lambda | V100 / 16 GB | 8 | 4.4 | 289 | 66 |
ACE Cloud | A100 / 80 GB | 2 | 6.200 | 406 | 65 |
Oblivus Cloud | V100 / 16 GB | 1 | 0.65 | 42 | 65 |
Paperspace by DigitalOcean | A100 / 80 GB | 4 | 12.72 | 821 | 65 |
Vultr | A100 / 80 GB | 8 | 20.833 | 1,362 | 65 |
Paperspace by DigitalOcean | A100 / 80 GB | 2 | 6.36 | 406 | 64 |
Latitude.sh | A100 (80 GB) | 8 | 23.2 | 1,362 | 59 |
Oblivus Cloud | V100 / 16 GB | 2 | 1.3 | 77 | 59 |
Oblivus Cloud | V100 / 16 GB | 4 | 2.6 | 153 | 59 |
Paperspace by DigitalOcean | A100 / 40 GB | 1 | 3.09 | 179 | 58 |
Paperspace by DigitalOcean | A100 / 80 GB | 8 | 25.44 | 1,362 | 54 |
CoreWeave | V100 / 16 GB | 1 | 0.800 | 42 | 53 |
Cirrascale | A100 / 80 GB | 8 | 26.030 | 1,362 | 52 |
Exoscale | V100 / 16 GB | 4 | 3.32 | 153 | 46 |
ACE Cloud | A100 / 80 GB | 2 | 9.280 | 406 | 44 |
Vultr | H100 / 80 GB | 1 | 7.5 | 322 | 43 |
Datacrunch | V100 / 16 GB | 1 | 1 | 42 | 42 |
Datacrunch | V100 / 16 GB | 2 | 2 | 77 | 39 |
Datacrunch | V100 / 16 GB | 4 | 4 | 153 | 38 |
Exoscale | V100 / 16 GB | 2 | 2.01 | 77 | 38 |
Cirrascale | A100 / 80 GB | 4 | 22.960 | 821 | 36 |
Datacrunch | V100 / 16 GB | 8 | 8 | 289 | 36 |
Exoscale | V100 / 16 GB | 1 | 1.38 | 42 | 30 |
OVHcloud | V100 / 16 GB | 1 | 1.97 | 42 | 21 |
OVHcloud | V100 / 16 GB | 2 | 3.94 | 77 | 20 |
OVHcloud | V100 / 16 GB | 4 | 7.89 | 153 | 19 |
Paperspace by DigitalOcean | V100 / 16 GB | 1 | 2.3 | 42 | 18 |
* Memory and GPU model are not the only parameters. CPUs and RAM can also be important, however, they are not the primary criteria that shape cloud GPU performance. Therefore, for simplicity, we have not included number of CPUs or RAM in these tables.
** Training throughput is a good metric to measure relative GPU effectiveness. It measures the number of tokens processed per second by the GPU for a language model (i.e. bert_base_squad).1 Please note that these throughput values should serve as high level guidelines. The same hardware would have a significantly different throughput for your workload since there is significant throughput difference even between LLMs running on the same hardware.2
*** Excludes cost of storage, network performance, ingress/egress etc. This is only the GPU cost.3
Spot GPUs
Cloud | GPU / Memory* | # of GPUs | Spot | Throughput** | Throughput/$*** |
---|---|---|---|---|---|
Azure | A100 / 80 GB | 1 | 0.76 | 232 | 303 |
Azure | A100 / 80 GB | 4 | 3.05 | 821 | 269 |
Azure | A100 / 80 GB | 2 | 1.53 | 406 | 266 |
Jarvislabs | A100 / 40 GB | 1 | 0.79 | 179 | 227 |
GCP | A100 / 40 GB | 1 | 1.62 | 179 | 111 |
AWS | V100 / 16 GB | 1 | 0.92 | 42 | 46 |
AWS | V100 / 16 GB | 4 | 3.67 | 153 | 42 |
Azure | V100 / 16 GB | 1 | 1.04 | 42 | 40 |
AWS | V100 / 16 GB | 8 | 7.34 | 289 | 39 |
Azure | V100 / 16 GB | 2 | 2.08 | 77 | 37 |
Azure | V100 / 16 GB | 4 | 4.16 | 153 | 37 |
In all these throughput per dollar tables:
- Not all possible configurations are listed, more commonly used, deep learning focused configurations are included.
- West or Central US regions were used where possible
- These are the list prices for each category, high volume buyers can possibly get better pricing
Finally, it is good to clarify what “spot” means. Spot resources are:
– Interruptible so users need to keep on recording their progress. For example, Amazon EC2 P3, which provides V100 32 GB, is one of the most frequently interrupted Amazon spot services.4
– Offered on a dynamic, market-driven basis. The price for these GPU resources can fluctuate based on supply and demand, and users typically bid on the available spot capacity. If a user’s bid is higher than the current spot price, their requested instances will run.
Cloud GPU costs & availability
Lowest prices for most popular GPUs
GPU | Lowest price (USD/hr) | Vendor with the lowest price |
---|---|---|
Nvidia L4 | $0.38 | Seeweb |
Nvidia RTX4000 | $0.38 | Hetzner, Paperspace by DigitalOcean |
AMD 7900XTX | $0.39 | DataCrunch |
Nvidia T4G | $0.42 | AWS |
Nvidia M4000 | $0.45 | Paperspace by DigitalOcean |
Nvidia RTX6000 | $0.50 | Lambda Labs |
Nvidia T4 | $0.53 | Azure |
Nvidia V100 | $0.62 | DataCrunch |
Nvidia H100 | $2.49 | Lambda Labs |
Nvidia A100 | $1.29 | DataCrunch |
Sorting by lowest price. For other low cost options, you can check out cloud GPU marketplaces.
GPU availability
Input the model that you want in the search box to identify all cloud providers that offer it:
Provider | GPU | Multi-GPU | $/hour*** |
---|---|---|---|
AWS | M60 8 GB | 1, 2, 4x | 1.14 |
AWS | T4 16 GB | 1, 2, 4, 8x | 1.20 |
AWS | A10G 24 GB | 1, 4, 8x | 1.62 |
AWS | V100 16 GB | 1, 4, 8x | 3.06 |
AWS | V100 32 GB | 8x | 3.90**** |
AWS | A100 40 GB | 8x | 4.10**** |
AWS | A100 80 GB | 8x | 5.12**** |
Azure | K80 12 GB | 1, 2, 4x | 0.90 |
Azure | T4 16 GB | 1, 4x | 1.20 |
Azure | P40 24 GB | 1, 2, 4x | 2.07 |
Azure | P100 16 GB | 1, 2, 4x | 2.07 |
Azure | V100 32 GB | 8x | 2.75 |
Azure | V100 16 GB | 1, 2, 4x | 3.06 |
Azure | A100 40 GB | 8x | 3.40**** |
Azure | A100 80 GB | 1, 2, 4x | 3.67 |
Azure | A100 80 GB | 8x | 4.10**** |
GCP | T4 16 GB | 1, 2, 4x | 0.75 |
GCP | K80 12 GB | 1, 2, 4, 8x | 0.85 |
GCP | P4 8 GB | 1, 2, 4x | 1.00 |
GCP | P100 16 GB | 1, 2, 4x | 1.86 |
GCP | V100 16 GB | 1, 2, 4, 8x | 2.88 |
GCP | A100 40 GB | 1, 2, 4, 8, 16x | 3.67 |
OCI | A100 40 GB | 8x | 4.00 |
OCI | A100 80 GB | 8x | 3.05 |
OCI | A10 24 GB | 1,2,4x | 2.00 |
OCI | V100 16 GB | 1,2,4,8x | 2.95 |
OCI | P100 16 GB | 1,2x | 1.275 |
ACE Cloud | A2 (16 GB) | 1, 2x | 0.59 |
ACE Cloud | A30 (32 GB) | 1, 2x | 0.95 |
ACE Cloud | A100 (80 GB) | 1, 2x | 3.11 |
Alibaba Cloud | A100 80 GB | 8x | |
Cirrascale | A100 (80 GB) | 4, 8x | 5.74 |
Cirrascale | RTX A6000 (48 GB) | 8x | 1.12 |
Cirrascale | RTX A5000 (24 GB) | 8x | 0.51 |
Cirrascale | RTX A4000 (16 GB) | 8x | 0.34 |
Cirrascale | A40 (48 GB) | 8x | 1.44 |
Cirrascale | A30 (24 GB) | 8x | |
Cirrascale | V100 (32 GB) | 4, 8x | 1.92 |
Cirrascale | RTX 6000 (48GB) | 8x | 1.18 |
CoreWeave | H100 (80 GB) | 1x | 4.25 |
CoreWeave | A100 (80 GB) | 1x | 2.21 |
CoreWeave | A100 (40 GB) | 1x | 2.06 |
CoreWeave | V100 (16 GB) | 1x | 0.80 |
CoreWeave | A40 (48 GB) | 1x | 1.28 |
CoreWeave | RTX 6000 (48 GB) | 1x | 1.28 |
CoreWeave | RTX 5000 (24 GB) | 1x | 0.77 |
CoreWeave | RTX 4000 (16 GB) | 1x | 0.61 |
CoreWeave | Quadro RTX 5000 (16 GB) | 1x | 0.57 |
CoreWeave | Quadro RTX 4000 (8 GB) | 1x | 0.24 |
Crusoe Cloud | A6000 (48 GB) | 1, 2, 4, 8x | 0.92 |
Crusoe Cloud | A40 (48 GB) | 1, 2, 4, 8x | 1.10 |
Crusoe Cloud | A100 (80 GB) | 1, 2, 4, 8x | 1.45 |
Crusoe Cloud | H100 (80 GB) | 8x | |
FluidStack | H100 (80 GB) | 1x | 4.25 |
FluidStack | A100 (80 GB) | 1x | 2.21 |
Jarvis Labs | Quadro RTX 5000 16 GB | 1x | 0.49 |
Jarvis Labs | Quadro RTX 6000 24 GB | 1x | 0.99 |
Jarvis Labs | RTX A5000 24 GB | 1x | 1.29 |
Jarvis Labs | RTX A6000 48 GB | 1x | 1.79 |
Jarvis Labs | A100 40 GB | 1x | 2.39 |
Lambda Labs | Quadro RTX 6000 24 GB | 1, 2, 4x | 1.25 |
Lambda Labs | RTX A6000 48 GB | 1, 2, 4x | 1.45 |
Lambda Labs | V100 16 GB | 8x | 6.8 |
Latitude.sh | H100 (80 GB) | 1, 4, 8x | 4.40 |
Latitude.sh | A100 (80 GB) | 8x | 23.2 |
LeaderGPU | A100 (40 GB) | ||
LeaderGPU | A10 (24 GB) | ||
LeaderGPU | V100 (32 GB) | ||
Linode | Quadro RTX 6000 24 GB | 1, 2, 4x | 1.50 |
OVH | V100 32 GB | 1, 2, 4x | 1.99 |
OVH | V100 16 GB | 1, 2, 4x | 1.79 |
Paperspace | Quadro M4000 8 GB | 1x | 0.45 |
Paperspace | Quadro P4000 8 GB | 1, 2, 4x | 0.51 |
Paperspace | Quadro RTX 4000 8 GB | 1, 2, 4x | 0.56 |
Paperspace | RTX A4000 16 GB | 1, 2, 4x | 0.76 |
Paperspace | Quadro P5000 16 GB | 1, 2, 4x | 0.78 |
Paperspace | Quadro RTX 5000 16 GB | 1, 2, 4x | 0.82 |
Paperspace | Quadro P6000 24 GB | 1, 2, 4x | 1.10 |
Paperspace | RTX A5000 24 GB | 1, 2, 4x | 1.38 |
Paperspace | RTX A6000 48 GB | 1, 2, 4x | 1.89 |
Paperspace | V100 32 GB | 1, 2, 4x | 2.30 |
Paperspace | V100 16 GB | 1x | 2.30 |
Paperspace | A100 40 GB | 1x | 3.09 |
Paperspace | A100 80 GB | 1, 2, 4, 8x | 3.19 |
Seeweb | RTXA6000 (48 GB) | 1, 2, 3, 4, 5x | 0.74 |
Seeweb | RTXA6000 (24 GB) | 1, 2, 3, 4, 5x | 0.64 |
Seeweb | A30 (24 GB) | 1, 2, 3, 4, 5x | 0.64 |
Seeweb | L4 (24 GB) | 1, 2, 3, 4, 5x | 0.38 |
Seeweb | A100 (80 GB) | 1, 2, 3, 4, 5x | 2.22 |
TensorDock | A100 (80 GB) | 1x | 1.40 |
TensorDock | L40 (40 GB) | 1x | 1.05 |
TensorDock | V100 (16 GB) | 1x | 0.22 |
TensorDock | A6000 (48 GB) | 1x | 0.47 |
TensorDock | A40 (48 GB) | 1x | 0.47 |
TensorDock | A5000 (24 GB) | 1x | 0.21 |
TensorDock | A4000 (16 GB) | 1x | 0.13 |
TensorDock | RTX 4090 (24 GB) | 1x | 0.37 |
TensorDock | RTX 3090 (24 GB) | 1x | 0.22 |
TensorDock | RTX 3080 Ti (12 GB) | 1x | 0.17 |
TensorDock | RTX 3080 (10 GB) | 1x | 0.17 |
TensorDock | RTX 3070 Ti (8 GB) | 1x | 0.14 |
TensorDock | RTX 3060 Ti (8 GB) | 1x | 0.10 |
TensorDock | RTX 3060 (12 GB) | 1x | 0.10 |
Vast.ai | L40 (45 GB) | 1, 2, 4x | 1.10 |
Vast.ai | A100 (40 GB) | 1, 2, 4x | 1.40 |
Vast.ai | A40 (48 GB) | 1, 2x | 0.40 |
Vast.ai | A6000 (24 GB) | 1, 2, 4, 8x | 0.44 |
Vast.ai | A5000 (24 GB) | 1, 2, 4, 8x | 0.20 |
Vast.ai | A4000 (16 GB) | 1, 2, 4, 5, 8x | 0.15 |
Vast.ai | V100 (16 GB) | 2, 5x | 0.18 |
Voltage Park | H100 80 GB | 8x | 1.89**** |
Vultr | L40S 48 GB | 1, 2, 4, 8x | 1.75 |
Vultr | H100 80 GB | 1x | 7.50 |
Vultr | A100 80 GB | 1, 2, 4, 8x | 2.60 |
Vultr | A40 (48 GB) | 1, 4x | 1.83 |
Vultr | A16 (16 GB) | 1, 2, 4, 8, 16x | 0.51 |
*** On-demand price *($) per single GPU. Excludes cost of storage, network performance, ingress/egress etc. This is only the GPU cost.
**** Computed values. This was needed when single GPU instances were not available.5 6
Other cloud GPU considerations
Availability: Not all GPUs listed above may be available due to capacity constraints of the cloud providers and increasing demand for generative AI.
Data security: For example, cloud GPU marketplaces like Vast.ai offer significantly lower prices but depending on the specific resource requested, the data security of the workload could be impacted, givings hosts the capability to access workloads. Since we prioritized enterprise GPU needs, Vast.ai wasn’t included in this benchmark.
Ease of use: Documentation quality is a subjective metric but developers prefer some cloud providers’ documentation over others. In this discussion, GCP’s documentation was mentioned as lower quality than other tech giants’.7
Familiarity: Even though cloud providers put significant effort into making their services easy-to-use, there is a learning curve. That is why major cloud providers have certifications systems in place. Therefore, for small workloads, the cost savings of using a low cost provider may be less than the opportunity cost of the time it takes a developer to learn how to use their cloud GPU offering.
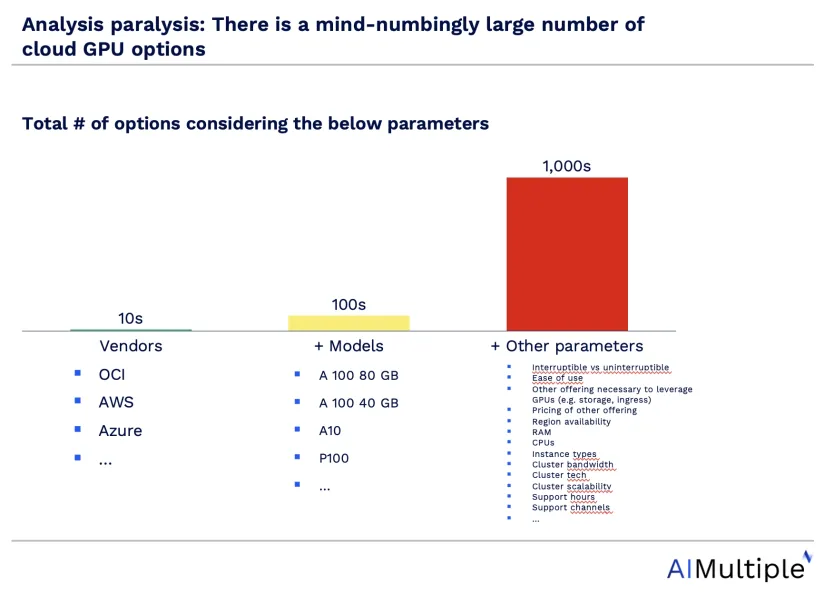
Buy GPUs or rent cloud GPUs
Buying makes sense
– If your company has the know-how and preference to host the servers or manage colocated servers.
– For uninterruptible workloads: For the volume of GPUs for which you can ensure a high utilization (e.g. more than 80%) for a year or more.8
– For interruptible workloads: The high utilization period quoted above needs to be a few times longer since on-demand (uninterruptible computing) prices tends to be a few times more expensive than spot (interruptible computing) prices.
Our recommendation for businesses with heavy GPU workloads is a mix of owned and rented GPUs where guaranteed demand runs on owned GPUs and variable demand runs on the cloud. This is why tech giants like Facebook are building their own GPU clusters including hundreds of GPUs.9
Buyers may be tempted to consider consumer GPUs which offer a better price/performance ratio however, the EULA of their software prohibits their use in data centers.10 Therefore, they are not a good fit for machine learning except for minor testing workloads on data scientists’ machines.
Cloud GPU benchmark methodology
Prices: Cloud GPU prices are crawled from
- Monthly from the top 3 providers.
- Twice a year from other providers.
Performance:
- All GPU models performance were measured on AWS.
- It is assumed that the same GPU provides the same performance in any cloud.
- High-end models like H100 were not available and therefore are not included above.
Performance on:
- Text finetuning was measured by finetuning Llama 3.2 with the first 5k conversations on FineTome using 1M tokens. Finetuning was carried over 5 epochs. Number of tokens times number of epochs was divided by finetuning time to identify number of tokens finetuned per second.
- Text inference was measured during inference of 1 million tokens including both input and output tokens. We divided number of tokens by the total duration to calculate the average number of tokens per second during inference.
- Image operations was measured by finetuning YOLOv9 with 100 images from SkyFusion for 4 epochs and then by inferencing the finetuned model with ~500 640×640 images.
Next steps:
- Data collection frequency will be increased
- We will run benchmarks in clouds other than AWS
What are the top cloud GPU hardware?
Almost all cloud GPUs use Nvidia GPU instances. AMD and other providers also offer GPUs however due to various reasons (e.g. limited developer adoption, lower price per performance etc.), their GPUs are not as widely demanded as Nvidia GPUs.
To see cloud GPU providers that offer non-Nvidia GPUs, please check out the comprehensive list of cloud GPU providers.
Read about all AI chips / hardware.
What are cloud GPU marketplaces?
Distributed cloud marketplaces like Salad, Vast.ai, and Clore.ai provide access to decentralized GPU computing power through a marketplace model. Users with idle hardware can list their GPUs for rent, while those needing GPU power can select from available resources at different price points. These platforms facilitate the connection between supply and demand without relying on centralized cloud providers. They offer a cost-effective and flexible solutions for GPU-intensive tasks.
Salad: decentralized network for tasks like AI training or crypto mining, with a focus on user rewards and ease of use.
Vast.ai: Connects GPU providers with users in need of affordable and scalable computational resources. Focus is on AI and machine learning workloads.
Clore.ai: A distributed marketplace for cloud GPUs. Focus is mostly on: AI, and other HPC needs.
Kryptex: A platform that enables users to earn cryptocurrency by renting out their GPUs. Main focus is to perform tasks like crypto mining or processing complex calculations.
Nvidia DGX Cloud Lepton: Connects AI developers with Nvidia’s network of cloud providers, including CoreWeave, Lambda, and Crusoe, to access GPU resources. Focus is on providing a flexible marketplace for training and deploying AI models across diverse cloud platforms.11
What are the top cloud GPU platforms?
Top cloud GPU providers are:
- AWS
- Microsoft Azure
- CoreWeave
- Google Cloud Platform (GCP)
- IBM Cloud
- Jarvis Labs
- Lambda Labs
- NVIDIA DGX Cloud
- Oracle Cloud Infrastructure (OCI)
- Paperspace CORE by DigitalOcean
- Runpod.io
For more on these providers, check out cloud gpu providers.
If you are not sure about cloud GPUs, explore other options like serverless GPU.
For tools allow users to collaborate on, check out cloud collaboration tools.
If you are unclear about what cloud GPUs are, here is more context:
What is a cloud GPU?
Unlike a CPU, which may have a relatively small number of cores optimized for sequential serial processing, a GPU can have hundreds or even thousands of smaller cores designed for multi-threading and handling parallel processing workloads.
A cloud GPU refers to a certain way of GPU usage that’s provided as a service through cloud computing platforms. Much like traditional cloud services, a cloud gpu allows you to access high-performance computing resources spot or on-demand, without the need for upfront capital investment in hardware.
What are the functions/application areas of cloud GPUs?
Cloud GPUs are primarily used for processing tasks that require high computational power. Here are some of the primary uses for cloud GPUs:
Machine Learning and AI
GPUs are particularly effective at handling the complex calculations required for machine learning (ML) and artificial intelligence (AI) models. They can process multiple computations in parallel, making them suitable for training large neural networks and algorithms.
Deep learning
Deep learning is a sub-field of machine learning. Deep learning algorithms greatly benefit from the parallel processing capabilities of GPUs, making training and inference faster and more efficient.
Data processing
Data analysis
GPUs are used to accelerate computing and data processing tasks, such as Big Data analysis and real-time analytics. They can handle high-throughput, parallel processing tasks more efficiently than CPUs.
Scientific computing
In scientific research, cloud GPUs can handle computations for simulations, bioinformatics, quantum chemistry, weather modeling, and more.
Simulations
Certain complex simulations can run more efficiently on GPUs.
Gaming & entertainment
Cloud GPUs are used to provide cloud gaming services, such as Google’s Stadia or NVIDIA’s GeForce Now, where the game runs on a server in the cloud, and the rendered frames are streamed to the player’s device. This allows high-quality gaming without the need for a powerful local machine.
Graphics rendering
GPUs were initially designed to handle computer graphics, and they still excel in this area. Cloud GPUs are used for 3D modeling and rendering, 3D visualizations, virtual reality (VR), computer-aided design (CAD), and computer-generated imagery (CGI).
Video processing
They’re used in video encoding and decoding, video editing, color correction, effects rendering, and other video processing tasks.
Cryptocurrency mining
GPUs are also used in tasks like cryptocurrency mining. However application-specific integrated circuits (ASICs) are offering better economics for more commonly mined crypto currencies.
Notes
Cloud providers are constantly updating their offering, this research will be constantly updated.
External links
- 1. GPU Benchmarks for Deep Learning | Lambda.
- 2. LLM-Perf Leaderboard - a Hugging Face Space by optimum. Hugging Face Optimum
- 3. website/docs/cloud-gpus/cloud-gpus.csv at main · the-full-stack/website · GitHub.
- 4. GPU Benchmarks for Deep Learning | Lambda.
- 5. 2023 GPU Pricing Comparison: AWS, GCP, Azure & More | Paperspace.
- 6. CloudOptimizer.
- 7. Cloud GPU Resources and Pricing | Hacker News.
- 8. Cloud GPU Resources and Pricing | Hacker News.
- 9. Meta Collaborates with NVIDIA on AI Research Supercomputer | NVIDIA Blog.
- 10. License for Customer use of GeForce Software | NVIDIA.
- 11. Nvidia Pushes Further Into Cloud With GPU Marketplace - WSJ. The Wall Street Journal
Comments
Your email address will not be published. All fields are required.