Top 5 Expectations Regarding the Future of NLP in 2024
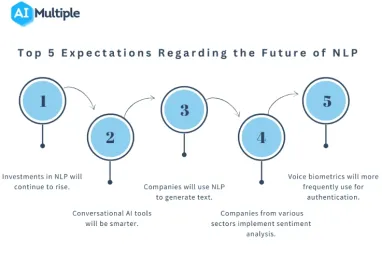
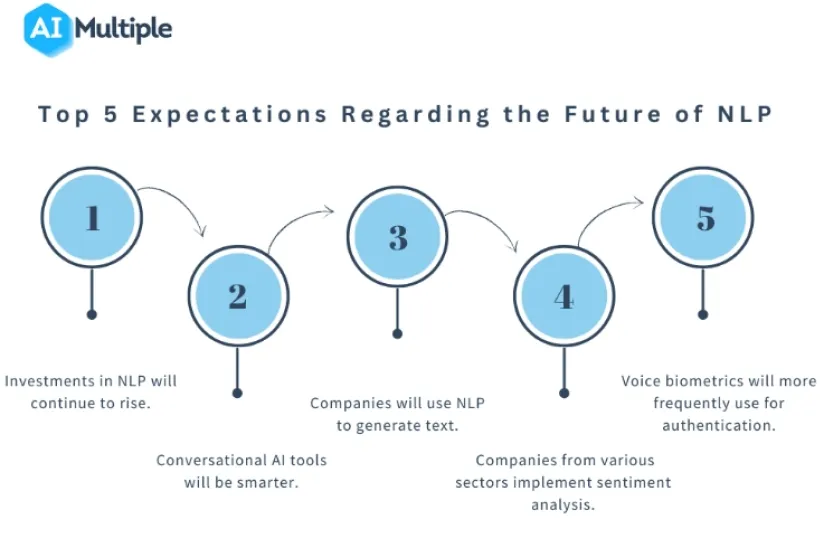
A subfield of artificial intelligence (AI) known as “natural language processing,” or simply “NLP,” enables machines to comprehend, decode, and interpret human language. NLP models can be trained to understand data in text format (such as PDF) or audio format (such as voice commands). The use of NLP technology is widespread among numerous industries already.
Businesses can now create more complex NLP models because of advancements in AI processors and chips, which has a favorable impact on investments and the adoption rate of the technology. The typical use cases of technology may alter as a result of more efficient NLP models. To aid executives from a range of businesses in making investment decisions, we present our top 5 predictions for the future of NLP in this article (see Figure 1).
1. Investments in NLP will continue to rise
According to Market & Market report,1 As of 2022, the NLP market size is around $16 billion in 2022 and will reach approximately $50 billion in 2027, representing an average annual growth rate of over 25% (see Figure 2). According to analysis, North America is the biggest market for NLP. On the other hand, the East Asia region invests heavily on NLP solutions.
Figure 2: NLP market overview.
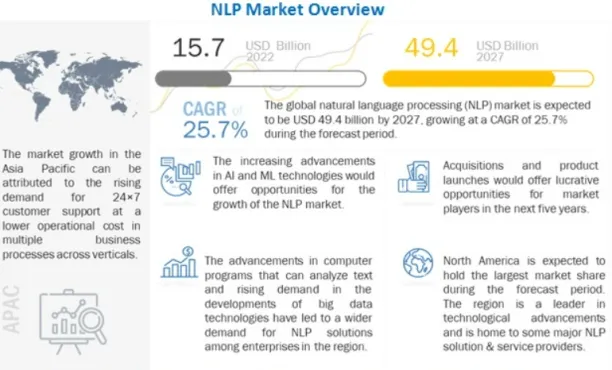
Rapid growth of the NLP market is associated with 3 factors.
1. Advancements in machine learning technologies
NLP models’ brains can be equated to AI chips. The more powerful the chips, the more computational power the machines have and the more human-like interactions they can carry out. AI chip makers design processors that can process more parameters, increasing the model size of NLP systems.
As you can see from Figure 3, we had models that processed fewer than 100 million parameters in 2018 (which is impressive already). NLP models can now interpret more than 100 billion parameters, which indicates development of more than 1,000 fold.3 Although not all organizations use such big models (and they should not, it is not a smart investment for lots of businesses), improvements in chip technology positively affect the general capacity of NLP models.
Figure 3: The model size of large language models.
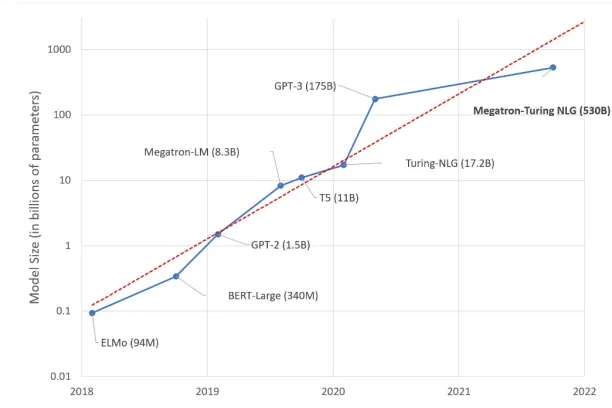
2. Enhanced data availability and quality
Another factor that enhances the ability of NLP systems is the availability and quality of the data. Figure 4 illustrates the exponential growth in data availability, which is anticipated to continue.5
To enhance the quality of training data, numerous data labeling tools may annotate text or audio data. These two together also contribute to the expansion of the NLP market.
Figure 4: Volume of data
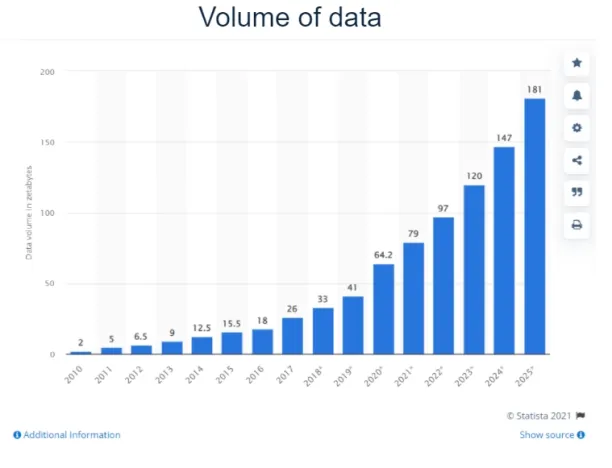
NLP models require training data, and AI data services can provide that. Check out the following articles to find the right AI data service for your projects:
- Top 12 AI Data Collection Services & Selection Criteria
- Data Crowdsourcing Platform: 10+ Companies & Criteria
To learn more about NLP data annotation, you can read our Data Labeling For Natural Language Processing (NLP) article.
3. Rising expectations of customers
According to research by Accenture, more than 75% of CEOs want to entirely alter their approach to managing customer relationships to keep up with changing consumer needs.7 Businesses are forced to implement NLP models as a result of customer expectations for quick interactions with brands.
2. Conversational AI tools will be smarter
Conversational AI is a subdivision of NLP that understands and responds to people. It is the technology behind:
- Chatbots
- Intelligent virtual assistants (IVAs)
- Voice bots (voice assistants)
- Digital workers (digeys).
Conversational AI tools can better recognize the nuance of human languages thanks to advancements in NLP models (Intent recognition). Additionally, these tools communicate with people better due to enhanced natural language understanding (NLU).
Thanks to advancements in conversational AI, we expect that the following 3 terms will be more relevant for companies shortly:
1. Conversational commerce
Conversational commerce is a new marketing strategy that aims to increase the comfort of clients. Businesses that engage in conversational commerce use omnichannel platforms where live agents, chatbots, and mass messaging tools communicate with customers on different channels such as:
- WhatsApp (WhatsApp Business API)
- Facebook Messenger
- Mobile applications of companies
- Websites of companies
- Call centers of companies, etc.
Conversational commerce is a suitable strategy for retail, e-commerce, and hospitality sectors. Here are some use cases conversational commerce:
- Product recommendation via NLP: Users could be familiar with their issue (such as a water leak) but not with the products needed to solve it (e.g., roof shingles, tar). Through two-way communication between users and chatbots, product discovery with NLP helps clients discover relevant items (see Figure 5).
- Customer support: Clients have a variety of queries, from delivery details to frequently asked questions. Conversational AI tools allow companies to automate answering customer inquiries.
- Visa eligibility screening: Hospitality chatbots can assess your visa eligibility according to the personal information users provide.
Figure 5: By explaining the product details, a chatbot makes a product recommendation via NLP.
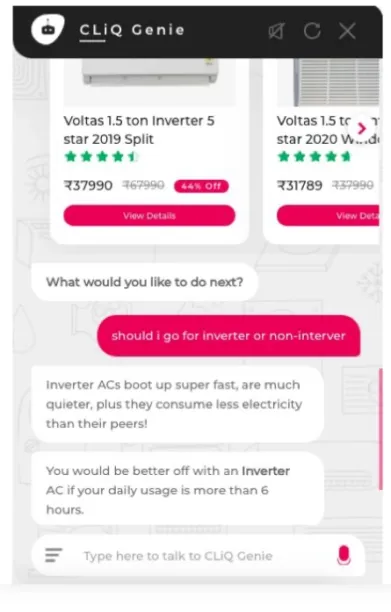
If you want to start your conversational commerce journey but have difficulties finding a suitable vendor you can read our following articles:
- Conversational Commerce Platforms: Data-driven Benchmarking.
- WhatsApp Business Partners: Everything You Need to Know.
2. Conversational banking
Conversational banking is the implementation of conversational commerce for financial services. Finance institutions leverage their customer interactions via:
Thanks to conversational banking, finance companies can automate:
- Customer onboarding.
- Document collection and verification process for issuing a mortgage.
- Providing stock recommendations (see Figure 7).
Figure 7: Chatbot provides stock ideas to customers.
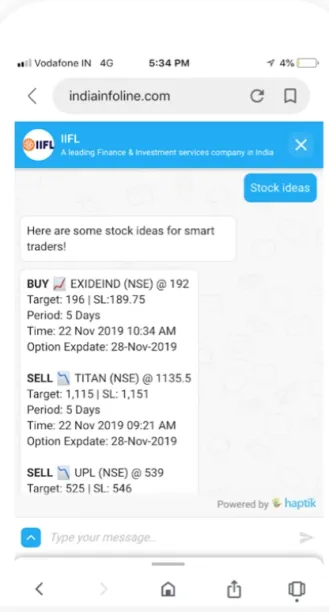
3. Intelligent automation
Employees may engage with intelligent automation technologies like digital workers and instruct them to carry out a variety of activities thanks to conversational AI (see Figure 8). End-to-end automation is provided through intelligent automation tools. They can work continuously and autonomously. Thus, they are effective tools for augmenting your employees and increasing their productivity.
Figure 8: How employees engage with digital workers.
Intelligent automation tools such as digital workers have the following use cases:
- Writing and sending emails.
- Extracting data from CRM and ERP accounting tools.
- Interpreting and visualizing data.
- Recruiting
- Reporting and more.
You can find our detailed expectations regarding conversational commerce by reading our Top 5 Expectations Concerning the Future of Conversational AI article.
3. Companies will use NLG to generate text
Natural language generation (NLG) is a sub-branch of NLP. NLG is already a useful AI application for content creators and marketers. However, AIMultiple considers more companies will use automated text generation and NLP-driven content editor tools since
- Companies started to invest more in marketing. For instance, in 2022, Companies’ marketing spending expanded from 6.4% to 9.5% of their total budgets.
- Around 60% of companies gain new customers thanks to content marketing.
NLP can ease marketers’ tasks due to the following use cases:
- Translation of content: It is beneficial for companies to engage with customers via their preferred languages. The developments in NLP allow high-quality machine translation. Some vendors specifically provide automated translation services. However, over the years even Google Translate improved its accuracy.
- Paraphrasing content: There are tools that polish users’ writing. Such tools rewrite the content and make it more reader-friendly.
- Editing content: Even Microsoft Word flags errors and improper grammatical usage. Nowadays, there are specialized NLP models that proofread content.
- Generating content: Using only AI today, it is possible to create original content. You can choose the subject. Following that, NLP algorithms produce original content based on Google search inputs.
- Providing SEO-friendly advice: NLP models also provide data-driven recommendations that can optimize your content to make it appear on the first page of Google searches. These recommendations can be regarding (see Figure 9):
- Ideal word count of the topic
- Number of images you should use
- Keyword densities etc.
Figure 9: Example of SEO recommendations concerning the “future-of-nlp” keyword.
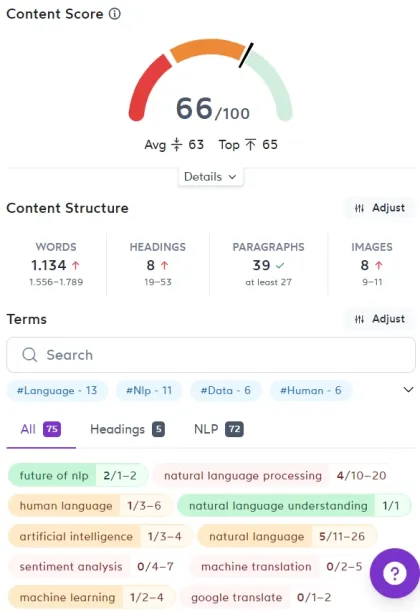
You can read our Top 10 Content Writing Best Practices article to improve your content marketing.
4. More companies from various sectors implement sentiment analysis
Sentiment analysis is an NLP application that uses big data as a source of insights. It analyzes consumer satisfaction by measuring the attitude of speech or text (negative, neutral, or positive).
Sentiment research is crucial for firms across industries since studies on consumer behavior show a strong link between client satisfaction, revenue, and client loyalty.8 Without implementing sentiment analysis, it is hard to measure exact customer happiness.
AIMultiple considers the following 3 industries/departments that will benefit a lot from sentiment analysis:
1. Finance
According to studies:
- Stock prices
- Commodities
- Coin values (see Figure 10).
relate to how the general public feels about particular financial assets. As a result, investor mood, industry reports, social media, and traditional media sentiment about the assets can all offer valuable investment data.
Figure 10: Relationship between BTC/USD and sentiment analysis that took place on Twitter.
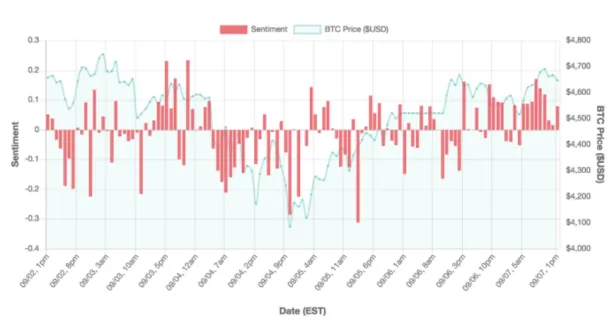
Therefore, we anticipate financial institutions will use sentiment analysis more effectively in the future.
2. E-commerce
Due to intense competition in e-commerce business, companies must come up with creative solutions to identify and address customer service inefficiencies. Sentiment analysis can help e-commerce firms in this regard since
- They can find products that customers satisfy (see Figure 11)
- They can scrape internet data to see how their e-commerce platform works in terms of customer service, product delivery, etc.
Figure 11: Sentiment analysis for product reviews:
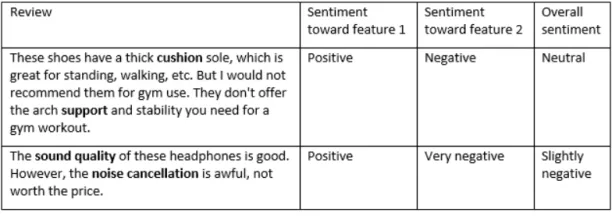
3. HR
According to Deloitte,11“around 75% of CEOs think that the Great Resignation poses the greatest threat to their businesses. Sentiment analysis can be used by HR departments to pinpoint the primary causes of employee attrition. Once you have determined the main pain points, you can take action to prevent Great Resignation.
5. Usage of voice biometrics will become more common
There are various applications for speech recognition in business. However, a special use of it known as voice biometrics may become more popular in the future because it boosts authentication security by using people’s voiceprints as a source of identification.
Voice biometrics has the following benefits:
- People’s voices, pronunciations, tones, and pitches are unique characteristics and they are almost impossible to mimic exactly. Thus, voice biometrics might provide more security than classic passwords.
- People frequently forget their passwords which causes dissatisfaction.
AIMultiple considers that voice biometrics will be used more frequently for detecting fraudulent transactions. We also expect that more companies from the healthcare sector will use speech recognition since data privacy is an important concern for healthcare companies.
To find out about the differences between NLP and NLU you can read our NLU vs NLP: Main Differences & Use Cases Comparison article.
If you have further questions regarding the NLP you can reach us:
This article was drafted by former AIMultiple industry analyst Görkem Gençer.
External Links
- 1. “Natural Language Processing (NLP) Market“. Market and Markets. August 2022.
- 2. Same with source (1).
- 3. “Large Language Models: A New Moore’s Law?“. Hugging Face. October 2021.
- 4. Same with source (3).
- 5. “Volume of data/information created, captured, copied, and consumed worldwide from 2010 to 2020, with forecasts from 2021 to 2025“. Statista. September 2022.
- 6. Same with source (5)
- 7. “The future of customer conversation: More than words, more than AI“. Accenture. (2021).
- 8. Sharma, A. et al. “Relationship Between Customer Satisfaction and Loyalty“. SSRN. August 2021.
- 9. “Understanding Cryptocurrencies with Sentiment Analysis.” Medium.
- 10. “Product Feature- vs. Review-Level Sentiment Analysis: When the Parts are Worth More than the Whole“. Towards Data Science. February 2021.
- 11. From Great Resignation to Great Reimagination“. Deloitte. 2022.

Cem has been the principal analyst at AIMultiple since 2017. AIMultiple informs hundreds of thousands of businesses (as per similarWeb) including 60% of Fortune 500 every month.
Cem's work has been cited by leading global publications including Business Insider, Forbes, Washington Post, global firms like Deloitte, HPE, NGOs like World Economic Forum and supranational organizations like European Commission. You can see more reputable companies and media that referenced AIMultiple.
Throughout his career, Cem served as a tech consultant, tech buyer and tech entrepreneur. He advised businesses on their enterprise software, automation, cloud, AI / ML and other technology related decisions at McKinsey & Company and Altman Solon for more than a decade. He also published a McKinsey report on digitalization.
He led technology strategy and procurement of a telco while reporting to the CEO. He has also led commercial growth of deep tech company Hypatos that reached a 7 digit annual recurring revenue and a 9 digit valuation from 0 within 2 years. Cem's work in Hypatos was covered by leading technology publications like TechCrunch and Business Insider.
Cem regularly speaks at international technology conferences. He graduated from Bogazici University as a computer engineer and holds an MBA from Columbia Business School.
To stay up-to-date on B2B tech & accelerate your enterprise:
Follow on
Comments
Your email address will not be published. All fields are required.