Machine Learning
Federated Learning: 5 Use Cases & Real Life Examples ['25]
McKinsey highlights inaccuracy, cybersecurity threats, and intellectual property infringement as the most significant risks of generative AI adoption.Federated learning addresses these challenges by enhancing accuracy, strengthening security, and protecting IP, all while keeping data private.
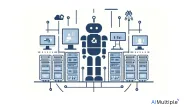
43 Back Office Automation Examples in '25: RPA, WLA, AI/ML
Many tasks in IT, HR, and finance are repetitive, data-based, and labor intensive, which makes the back-office a great candidate for automation using AI and RPA, and workload automation. According to research, RPA in the back office can reduce 40% of the cost of employees, providing a quick and tangible ROI to organizations.
Meta Learning Explained: How It Enhances Machine Learning
Traditional machine learning often relies on extensive trial and error to fine-tune models, leading to high costs and longer time-to-market. Meta learning overcomes this challenge by leveraging insights from past experiments, simplifying the optimization process, cutting down computational time, and enhancing overall model efficiency.
Few-Shot Learning: Methods & Applications in 2025
Imagine a healthcare startup developing an AI system to detect rare diseases, but there’s a problem: there’s not enough labeled data to train a traditional machine learning model. This is where few-shot learning (FSL) comes in.
![45 Statistics, Facts & Forecasts on Machine Learning [2025]](https://research.aimultiple.com/wp-content/uploads/2020/10/Screen-Shot-2023-01-23-at-8.55.33-PM-190x97.png.webp)
45 Statistics, Facts & Forecasts on Machine Learning [2025]
Machine learning is the study of computer algorithms that learn through data. Machine learning is regarded as a subset of artificial intelligence. Surveys and market researches are the best way to understand the overall view of the machine learning market because numbers can reveal metrics from the importance of the market to its challenges.
Machine Learning Accuracy: True-False Positive/Negative
Selecting the right metric to evaluate your machine learning classification model is crucial for business success. While accuracy, precision, recall, and AUC-ROC are common measurements, each reveals different aspects of model performance. We’ve analyzed these metrics to help you choose the most appropriate one for your specific use case, ensuring your models deliver real value.