Data collection is a crucial stage in developing AI/ML models, directly influencing their real-world performance. Whether you work with a data collection service or gather data yourself, it’s vital to execute this process correctly.
Here, we explore crowdsourcing, an effective method for data gathering, to help businesses select the best approach for their AI/ML projects.
What is crowdsourced data collection?
Crowdsourcing is a large group of dispersed participants all over the world who produce goods and services in exchange for compensation or as a voluntary job.
When it comes to AI development, crowdsourcing plays a pivotal role, especially in data collection. The service provided by this crowdsourced network in the AI context is to amass the specific kind and volume of data that a client necessitates for their AI development project. This data is critical as it forms the foundation upon which machine learning models are trained, refined, and subsequently deployed in various applications.
How to crowdsource data?
Collecting AI data through crowdsourcing can be performed through the following steps:
1. Determine the type of data that will be collected
Begin by clearly defining the nature and format of the data that’s required. This could range from images, videos, and text to more specific categories like personal data, medical data, or public datasets. It’s crucial to pinpoint the exact type of data needed to ensure accuracy and relevance in the AI training process.
2. Determine the participants
Identify the profile of participants who would be best suited to provide the data you need. For example, if you’re collecting medical data, you might want to engage healthcare professionals or patients. Understand the demographics and expertise required to ensure data accuracy.
3. Create (or outsource) a platform
Establish a user-friendly and secure platform where participants can register, submit their data, and engage in the data collection process. The platform should be intuitive and should allow for easy data management, with functionalities to sort, filter, and monitor incoming data.
4. Provide instructions to gather data
Clearly communicate the data collection process to participants. Offer detailed guidelines, possibly accompanied by examples or demonstrations, to ensure that they understand the expectations and the standards required.
5. Create a compensation method for the participants
Decide on a fair and motivating compensation mechanism. This could be monetary rewards, vouchers, recognition certificates, or other incentives. Ensure that the compensation aligns with the complexity and volume of the task.
6. Gather data through the platform
Facilitate the data submission process on the chosen platform. Make sure that the platform can handle large volumes of data and that it maintains data integrity. Provide support mechanisms, such as help desks or chatbots, to assist participants with any queries or challenges they might encounter.
7. Regularly evaluate data collection procedures
Continuously monitor and assess the efficiency and effectiveness of the data collection process. Identify any bottlenecks, quality issues, or areas for improvement. Adjust procedures as needed to optimize the flow of accurate and relevant data.
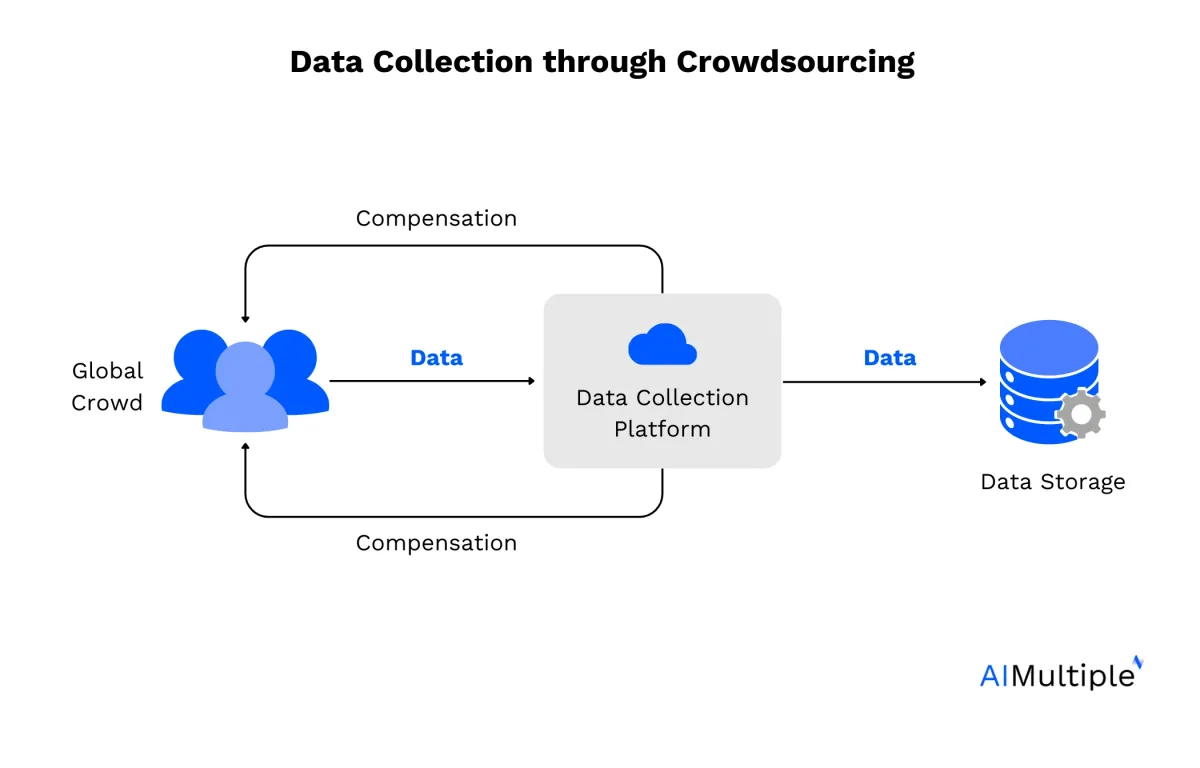
Benefits of data collection through crowdsourcing
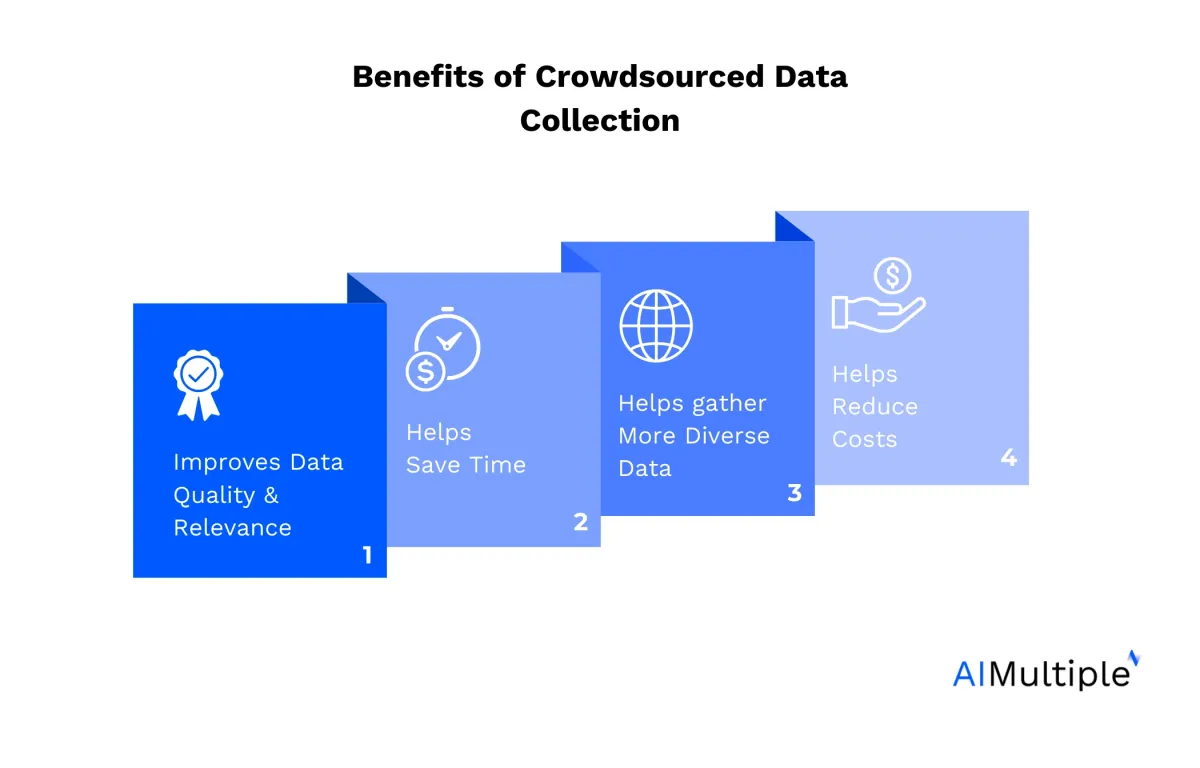
This section explores the advantages of data collection through crowdsourcing.
1. Improves data quality and relevance
Experienced crowdsourcing platforms have set standards of data quality inspection and an established recruitment process to ensure the relevance and accuracy of the data collected. For instance, an experienced crowdsourcing platform should:
- Evaluate the skills of the data collectors to match them to different data collection tasks aligned with their skills.
- Additionally, provide necessary training and instruction to data collectors on how to improve their data collection activities. They should also receive any domain-specific knowledge that might be helpful in the data collection process.
Prioritizing price over data quality is not a good practice. If a crowdsourcing platform is providing a cheaper rate, make sure they do not fall short on quality.
2. Helps save time
In the complete lifecycle of an AI project, around 80%1 of the time is spent on data-related tasks, from which data sourcing/collecting accounts for a significant portion. This only leaves about 20% of the time for core development tasks.
This is why developers usually delegate data collection to crowdsourcing platforms since it gives them more time to focus on development tasks. Data crowdsourcing platforms can recruit a large number of contributors in a short period of time, which makes them suitable for large data collection projects.
It should also be noted that some crowdsourcing platforms also offer annotation services to help further save AI project time.
3. Helps gather more diverse data
The performance of an AI model depends on the quality of data that was used to train it. A high-quality dataset translates into a diverse, unbiased, and all-inclusive AI model.
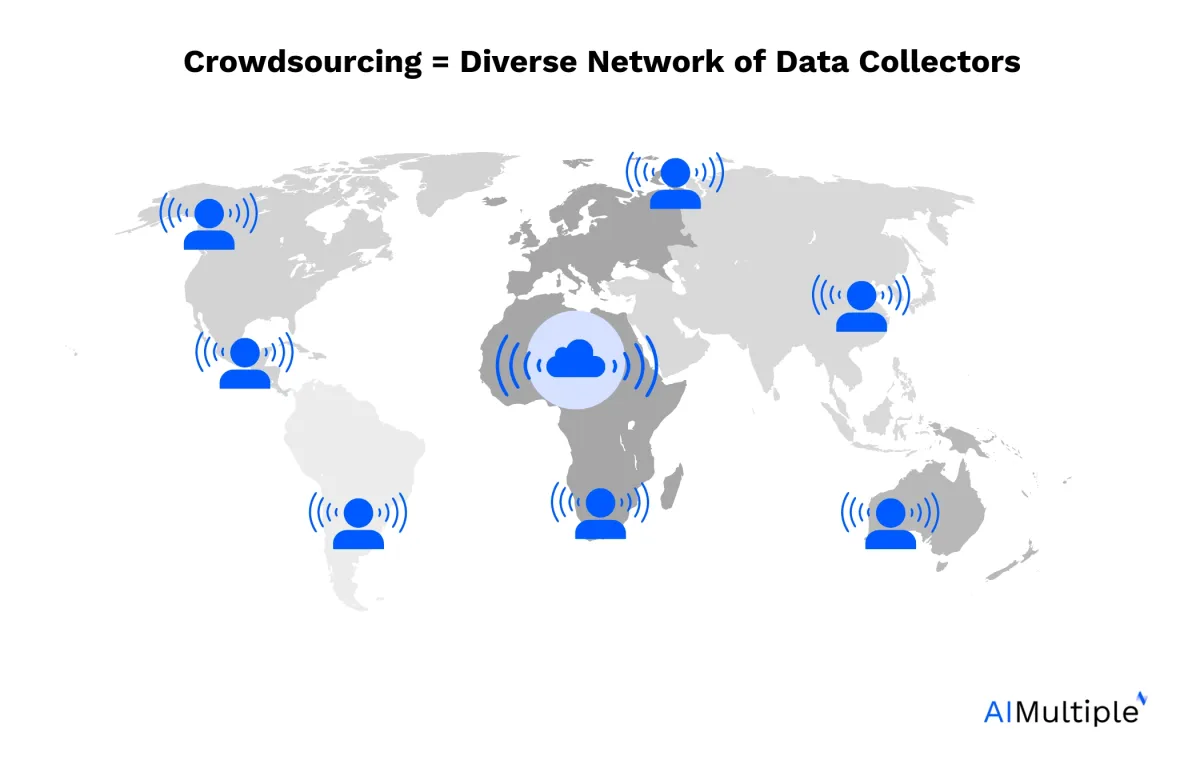
Crowdsourcing enables convenient access to a large base of skilled data collectors. As a result, data can be easily and quickly collected from thousands of diversified sources dispersed around the world.
4. Helps reduce costs
Collecting large datasets can be expensive since it is a labor-intensive and error-prone task. For instance, if a speech-to-text platform requires audio data from 20 different countries, each with a different language, it can be costly for the developers to collect it manually.
Crowdsourced platforms can perform data collection at significantly lower prices. This is possible because they work with a skilled workforce on a pay-per-task model and have a scalable business model.
Best practices to overcome the challenges of crowdsourcing data
While crowdsourcing data collection is an effective method of data collection, it can create some of the most complex business challenges that cannot be overlooked. In this section, we highlight some of those challenges and provide some best practices to help overcome the barriers.
1. Lack of confidentiality
Lack of confidentiality is a drawback of data crowdsourcing. If the project is sensitive or secretive, it can be very difficult to maintain secrecy while working with thousands of data collectors all over the world.
1.1. Recommendations
You can implement robust data protection policies and secure data transmission methods. You can also leverage encryption and secure data storage solutions to safeguard the data. Also, confidentiality agreements should be signed by all data collectors before they begin work.
If your data collection platform does not provide built-in security features, it may be necessary to work with a third-party crowdsourcing service provider that already has data protection standards in place for secretive projects.
2. Difficult to track and evaluate data collectors
Another drawback of collecting data through crowdsourcing is the difficulty of tracking and evaluating the data collectors. For instance, it is possible that data collectors can intentionally or unintentionally submit plagiarized data or produce content of lower quality.
2.1. Recommendations
To overcome this challenge, it is important to regularly evaluate data collection or work with experienced crowdsourcing companies that have already established methods of checks and balances.
3. Difficult to vet the competency
Evaluating the level of skillset can also be a challenge while recruiting the crowd. For instance, if a company wants to gather a large-scale dataset in the Chinese language to train a large language model, it can be significantly challenging to hire recruiters fluent in that language.
3.1. Recommendations
To overcome this, companies should have a recruitment process with filters that can separate the experts from the amateurs. If companies opt for in-house data crowdsourcing projects, it is important to recruit skilled data collectors.
You can also work with a crowdsourcing data service provider with a large and diverse network of contributors from many countries.
Further reading
For guidance on finding the right tool/service for your project, check out our data-driven list of data collection/harvesting services.
Comments
Your email address will not be published. All fields are required.